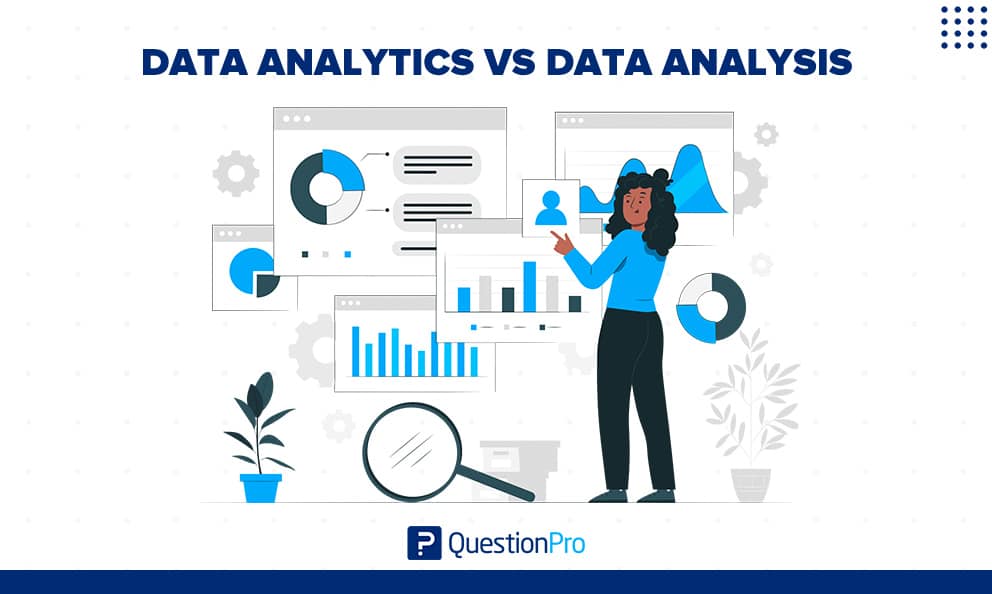
Data is becoming the new fuel for businesses because it helps them gain key insights and grow. However, there is a big difference between data analytics vs data analysis, and it’s important to know what it is. But, even though these words are often used interchangeably, they mean different things and have different values.
People frequently confuse data analysis and data analytics. Surprisingly, the terms are occasionally used interchangeably by data scientists and data analysts!
To clarify this misconception, let’s discuss the difference between Data Analytics vs Data Analysis. In this blog, we’ll look at both terms, how they differ, and how they’re used.
What is data analytics?
Data analytics refers to a broad range of data-related activities and concepts. It is a process for translating basic facts and figures into specific actions by examining raw data assessments and perceptions in the context of organizational problem-solving and decision-making.
The purpose is to help businesses make better decisions and achieve greater success. Analytics uses data, machine learning, statistical analysis, and computer-based models to gain insight and make better decisions from collected data.
A data analyst often works with structured data to address practical business problems through the use of technologies, data visualization software, and statistical analysis.
Data analytics is an excellent way for businesses and people to use data to identify concrete solutions for their concepts. An effective approach can provide a more comprehensive strategy for where your company can go. The following are some of the ways in which data analytics might help you:
- To identify trends and patterns.
- To seek out new opportunities.
- To determine possible risks and benefits.
- To make a strategy of action.
What is data analysis?
Data analysis consists of cleaning, manipulating data, modeling, and questioning data to discover relevant information. It is a vital part of data analytics. It helps us identify solutions by providing information.
There are several approaches we can take when it comes to data analysis. These are some of the approaches you can use depending on what you want to accomplish.
- A/B testing: A comparison of one test group to another.
- Data fusion, integration: It develops accuracy by analyzing and combining data from various sources.
- Data mining: This identifies patterns in massive data sets and extracts them for analysis.
- Machine learning: Here, computer algorithms are used to automate the process of developing analytical research models.
- Natural language processing (NLP): It uses computer algorithms to study human languages.
LEARN ABOUT: Data Mining Techniques
Key differences between data analytics vs data analysis
Data analytics and data analysis are frequently used synonymously. Data analytics is a general term for a field that includes the entire management of data and the methods and instruments employed.
On the other hand, data analysis is the process of dissecting a given data set into its component pieces and analyzing each one separately, as well as how the parts relate to one another. Let’s take a look at their differences and similarities now.
Data Analytics | Data Analysis |
Data analytics is a traditional or generic type of analytics used in enterprises to make data-driven decisions. | Data analysis is a specialized type of analytics used in businesses to evaluate data and gain insights. |
It has one or more users and generally consists of data collection, data validation, and data visualization and inspection. | It involved defining the data, looking into it, cleaning it up, and altering it to get a helpful result. |
It consists of numerous stages, such as data collecting and business data inspection. | Here to process data, raw data must first be defined in a meaningful way before relevant information can be recovered from it through data cleaning and conversion. |
It processes data using various technologies such as Tableau, Python, Excel, Google Analytics, and others. | It analyzes data using many tools such as SPARK, Google Fusion tables, Node XL, Excel, etc. |
This does not offer itself to descriptive analysis. | This can be subjected to descriptive analysis. |
With the use of this, one might discover anonymous relationships. | This cannot be used to find unknown relations. |
Inferential analysis is not included here. | Inferential analysis is supported here. |
Learn More: Data Analysis
Example of data analytics vs data analysis
Let’s look at a real-life example to help clarify the principles. The example provided will assist you in understanding the ideas.
Most of us are at least somewhat knowledgeable about the stock market. Imagine that you are a newbie and that you want to start your trade with a profit. Now, describe your initial plan of action.
- As a new trader, you’ve probably researched sharemarket and trend records to get a sense of what’s going on in the market. This technique includes data analysis.
- As a result of your newfound understanding of the stock pattern, you can now estimate the stock’s future market price and purchase some shares. This serves as an example of a data analytics process.
Data analytics vs data analysis, Which Is the better option?
The research and processes utilized by the analytics specialist to make predictions and inferences are challenging for a layperson to understand. Someone without the necessary expertise could find it challenging to comprehend post-processing, such as creating new ones from the dataset to produce a better and desired conclusion.
On the other hand, improved graphical and visual representations of data analysis are possible, allowing even illiterate persons to grasp the dataset’s contents more quickly and easily.
LEARN ABOUT: Pricing Analytics
Conclusion
Data is becoming the new fuel source for businesses. Using it properly, you can quickly increase overall sales and net income. You need to understand the distinction between data analysis and data analytics to accelerate growth, especially in today’s cutthroat environment.
Analytics is the study of previous data to make better decisions. Data interpretation is aided by data analysis. It provides crucial historical insights that enable us to understand what has occurred up to this point.
QuestionPro offers solutions for every issue and industry, making it far more than just survey software. In addition, we have data management softwares like our InsightsHub research library.
Organizations worldwide are utilizing knowledge management tools and systems, such as InsightsHub, to manage data better, shorten the time it takes to gain insights and enhance the utilization of historical data while cutting costs and raising ROI.