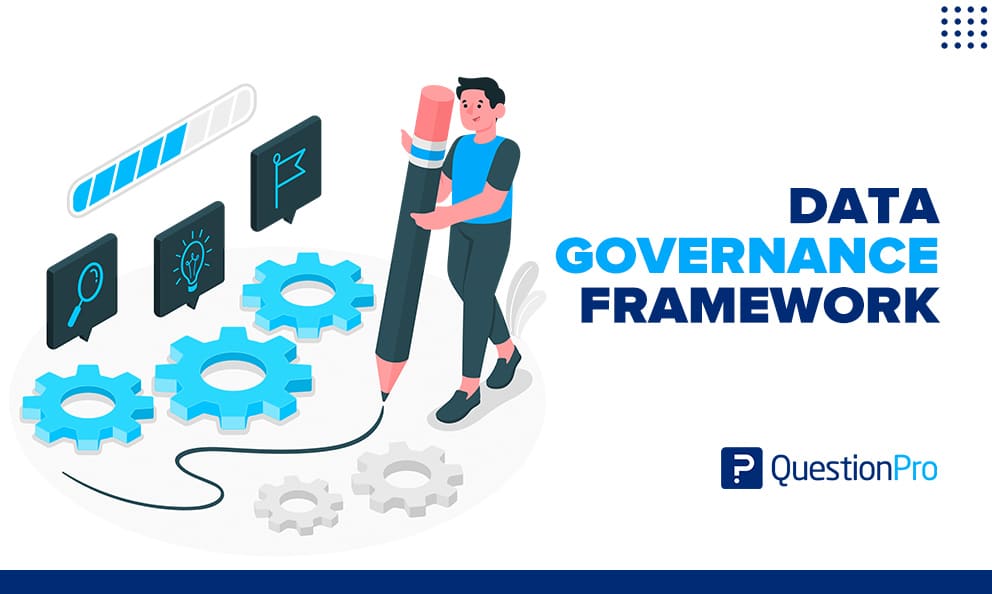
Now is the opportune moment if you’ve decided to establish a data governance framework. Effective Data collection, storage, and interpretation have become pivotal in enhancing an organization’s flexibility and competitiveness in today’s global business landscape. When managed successfully, these data assets can serve as the bedrock for digital transformation, allowing you to align them with your organization’s future business plans, models, and strategies.
For instance, within a business ecosystem, a well-structured data governance framework can assign relevant roles and responsibilities to ensure meticulous adherence to data standards. Selecting the data governance framework that best aligns with your specific needs is crucial to making the most informed choice for your company’s future business goals, models, and ambitions.
In the realm of data governance, a dedicated data governance team plays a pivotal role in spearheading data management efforts. This team ensures data ownership, oversees master data management, and implements best practices in data protection. A well-structured data management framework, including data modeling, data cataloging, and good data governance practices, forms the backbone of a successful data governance initiative. Moreover, incorporating data quality measurements into your good data governance strategy is essential to ensure that your data remains reliable and consistent, supporting informed decision-making in your organization.
What is Data Governance?
Data governance encompasses a comprehensive strategy for overseeing an organization’s data assets, including sensitive data. It entails the creation of guidelines, protocols, and norms to uphold data quality, security, and regulatory adherence. Within a core data governance program, organizations establish specific data governance frameworks and processes to ensure they actively and responsibly manage their data assets. Data governance includes defining roles and responsibilities, implementing data access controls, and continually monitoring and enhancing data governance practices.
Effective data governance is crucial for informed decisions, data integrity, and trust. It promotes a data-driven culture, maximizes data value, and supports responsible data management, aligning with strategic goals and compliance.
What is a Data Governance Framework?
A data governance framework establishes a unified set of rules and procedures for data collection, storage, and utilization. This framework simplifies and facilitates the scaling of fundamental governance processes, ensuring ongoing compliance, data sharing, and collaborative efforts, regardless of the rapid growth in data volume.
The primary objective of this data governance framework is to provide every member of the organization with a consistent and comprehensive view of the data. It encompasses the data itself, data links and lineages, technical and business context, data assessment, verification, clustering techniques, advanced analytics, and collaborative aspects.
By embracing this framework, organizations can enforce consistent data governance policies, rules, and definitions that apply to all facets of the organization’s data. Data governance frameworks empower organizations to deliver trustworthy data to various individuals in various roles, from corporate leaders to data stewards and engineers. The framework is critical in managing data throughout its lifecycle, from diverse data sources to its ultimate utilization. It ensures that data governance efforts are seamlessly integrated into the organization’s data management processes.
Importance of Data Governance Framework
Organizations must establish a comprehensive data governance framework to achieve tremendous business success. Such a framework provides a solid structure with numerous advantages:
Data Quality and Consistency:
This framework ensures data accuracy, reliability, and uniformity across the organization, fostering trust in the data and enabling more informed decision-making.
Compliance and Risk Mitigation:
By facilitating compliance with data privacy regulations and industry standards, it mitigates the risks related to data breaches and potential penalties. It also involves defining data access controls and establishing audit trails for enhanced data security.
Efficient Data Management:
The framework streamlines data-related processes through clearly defined roles and responsibilities, minimizing redundancy and enhancing operational efficiency.
Alignment with Business Goals:
Data governance aligns data management practices with an organization’s strategic objectives, ensuring that data drives business units growth, innovation, and competitive advantage.
Data-Driven Decision-Making:
Nurturing a culture of data-driven decision-making empowers employees at all levels to make informed choices based on high-quality data.
Stakeholder Trust:
By demonstrating unwavering commitment to data integrity, security, and privacy, the data governance framework builds and maintains trust among customers, partners, and stakeholders.
A strong data governance framework is essential for maximizing data potential. It manages risks, ensures compliance, and guides data from sources to decision-making, enhancing business performance and success.
LEARN ABOUT: Employee Experience Framework
How to Build a Data Governance Framework
You need to follow some tips and tricks and steps to build a data governance framework. Now we will explore some of these to help you build a data governance framework for your organization.
1. Document assets, procedures, and pipelines
Everything starts with being able to identify and document your physical data assets, procedures, and pipelines. This provides a foundation that accurately replicates the reality on the ground, detailing the structures that store data as well as the processes that transfer and consume data.
This creates the framework for offering thorough data lineage, impact analysis, and navigation tools that let stakeholders move about the environment contextually.
To break down organizational silos, you need a flexible data governance system that is aware of and supports hybrid cloud deployments, different data formats, and both traditional and modern data integration and movement methods (ETL, ELT, streaming, etc.).
It also needs to normalize the vast range of data-consuming use cases, technologies, and advanced analytics (BI/Reporting, ML/AI, and advanced analytics) into a single, all-encompassing physical picture.
2. Establish a business context
The capacity to build business context comes next. Flexible business vocabulary capabilities are essential in this regard. Business glossaries allow companies to define the meanings of various words used in the data world and then link those definitions to the actual data that exists on the company’s network infrastructure.
The true benefit arises when businesses can quickly develop and manage additional business data assets that represent their particular requirements and link those assets to every component of the relevant framework.
There are several ways to do this, such as data sharing agreements, regulatory compliance tagging, data categorization systems, and metrics that make it possible for enterprises to better understand their data assets’ implications, dangers, and value.
3. Knowing data quality
Business users need to understand data quality to balance its worth and insights before making choices. Knowing data quality is the third step of a data governance framework. It begins with supplying profile statistics to understand the data’s subtleties better. This builds the trust needed to make choices based on the information.
Accessibility to data quality standards is necessary so that stakeholders can understand what should be present, regularly compare the data to the rules, and seek quality correction when issues arise.
4. Stakeholder networking and cooperation
Stakeholder networking and collaboration are the following critical features to allow you to record and merge your data’s business and physical viewpoints. A read-only view of the data governance framework enables corporate stakeholders to find and explore their data in their function’s context and helps promote a data-driven culture.
Organizations can better manage and share their “tribal knowledge” by implementing controlled feedback loops and guided processes. These approaches also help them maintain and improve the framework’s defined roles and responsibilities.
To break down organizational silos, boost cross-functional collaboration, and raise organizational confidence in and strategic use of data, “data communities” and a social network may be built around data.
5. Automation
The last piece of the puzzle is automation. A framework for data governance wouldn’t be a difficult thing to do if you had previously done it. Setting up a flexible data governance system is one thing. But it’s another to keep it accurate, complete, and current. Stakeholders will no longer have faith in this facility if it is out-of-date or incomplete. And inaccurate data insights might have negative consequences for your organization.
So, suppose you can automate as much of your data governance framework as possible. In that case, you can be sure that it will stay relevant and flexible no matter where your digital transformation efforts take you.
LEARN ABOUT: Data Management vs Data Governance
Best Practices with Examples
Implementing a robust data governance framework necessitates the application of best practices for effective data asset management. Here are some recommended practices, accompanied by illustrative data governance framework examples:
Establish Clear Goals and Objectives:
Best Practice: Define precise, quantifiable, and attainable goals for your data governance initiatives. For example, develop a plan to enhance customer data accuracy by 10% over the next 12 months.
Create a Data Governance Team:
Best Practice: Assemble a dedicated team comprising roles like Data Stewards, Data Owners, and Data Governance Managers. For example, designate a Data Steward responsible for upholding data quality standards within the customer database.
Define The Data Ownership:
Best Practice: Assign ownership of specific datasets to individuals or departments. For example, entrust the marketing department with data ownership, while financial data falls under the purview of the Finance department.
Data Cataloging and Inventory:
Best Practice: Maintain a comprehensive data catalog that documents all data assets thoroughly. For example, develop a data catalog containing detailed descriptions, data lineage, and metadata for each dataset.
Data Quality Measurements:
Best Practice: Implement data quality metrics and conduct regular assessments to monitor data quality. For example, continuously track data accuracy using metrics such as error rates and completeness percentages.
Data Security and Privacy:
Best Practice: Ensure data security through stringent access controls and encryption measures. For example, enforce access restrictions to safeguard sensitive customer data, limiting access to authorized personnel.
Create a practical data governance framework by following best practices tailored to your organization. It aids data governance initiatives, manages data throughout its lifecycle, and ensures effective data governance, even in data warehouses. Moreover, it can enable data democratization while protecting sensitive data.
Conclusion
We have tried our best to explain a data governance framework and how we can build a data governance framework for business processes. Adopting a flexible and automated data governance framework will provide your organization with an accessible, complete, appropriate, and sustainable supply of data insight to support your business and help you build critical data capabilities and a data-driven business strategy.
LEARN ABOUT: Data Management Framework
QuestionPro is more than survey software, offering solutions for every topic and sector. Also, they offer data management software such as the InsightsHub research library. If you need any help regarding the data governance framework, contact the QuestionPro team.