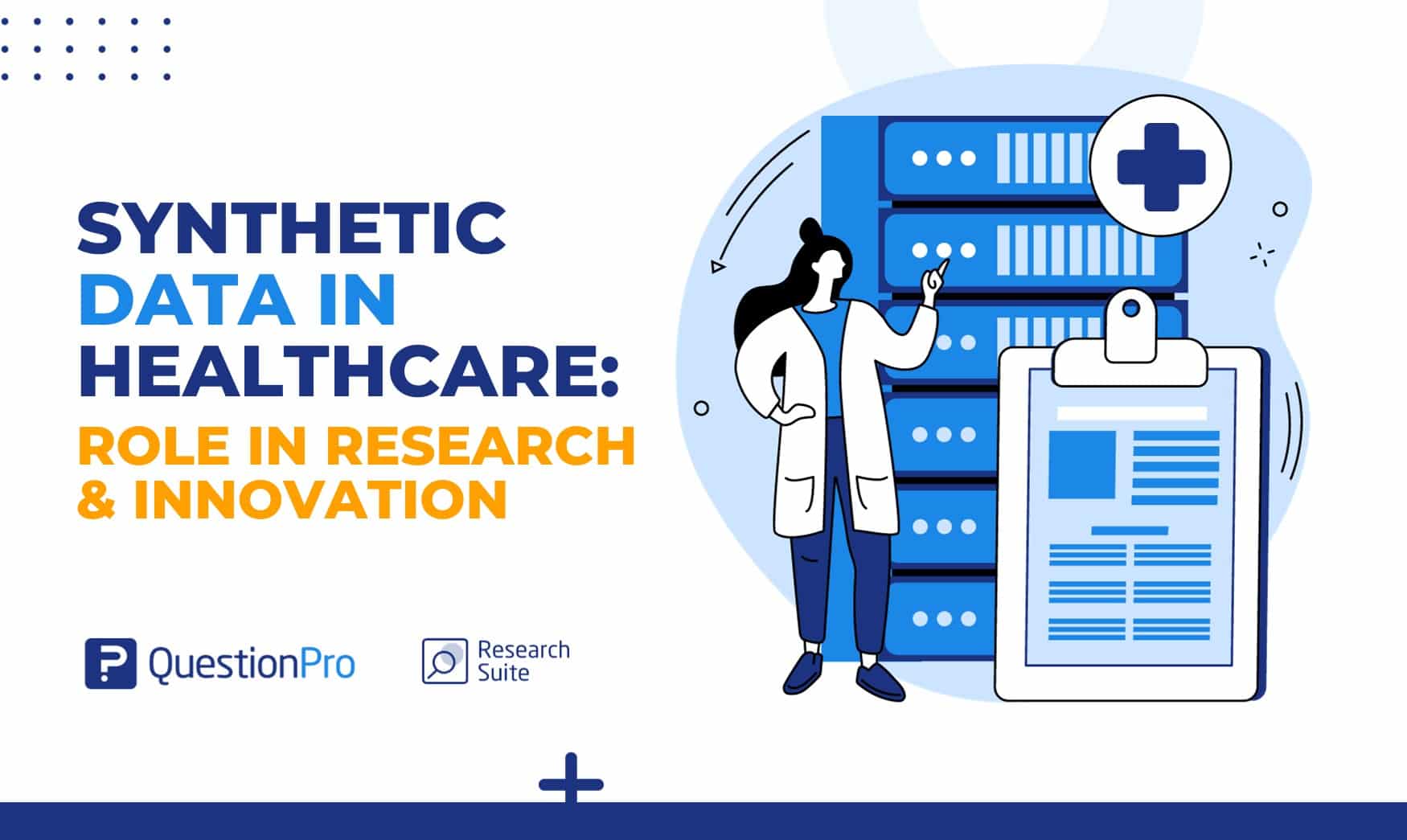
Synthetic data in healthcare is becoming a game-changer for you and many others in the medical field. It’s all about finding innovative solutions to our problems when getting critical healthcare information.
Data is critical in healthcare. It contributes to better healthcare, research, and the development of new ideas and treatments. Most data containing sensitive information about people’s health is kept private. It is difficult to disclose data that can be used to identify individuals. So, when researchers and analysts like you require this data, they face numerous challenges.
Synthetic data has the potential to be a significant tool in this sector because it allows the presentation of real patient health information while preserving privacy and confidentiality.
In this blog, we’ll learn about synthetic data in healthcare, the techniques used to generate this type of fake data, and its diverse usage for research and innovation.
What is Synthetic Data in Healthcare?
Synthetic data in healthcare refers to artificially generated data that replicates many characteristics of accurate patient health information without containing any actual patient-specific details.
Instead of using actual details about specific patients, you can use synthetic data that looks like the real stuff. You can use this to keep patient information private and safe. It helps researchers and doctors learn and test things without using actual patient data.
The Role of Synthetic Data in Healthcare
Synthetic data in healthcare helps safeguard patient privacy, comply with rules, secure data, and advance medical research. It lets researchers work with data that closely matches accurate patient data without compromising data security or privacy, leading to medical advances and better patient care.
Imagine a medical research team working on a study to develop a new treatment for a rare disease. In that case, the team needs access to patient data, including medical histories, test results, and treatment outcomes. Such research using actual medical data leads to significant privacy and legal problems because patient data must be kept safe and secure.
Instead of using actual patient records, the research team can create synthetic patient data that closely resembles genuine medical data. They can construct fake patient profiles with identical demographics, medical diagnoses, and treatment histories. These fake profiles protect actual patients’ privacy by removing personal information.
Synthetic Data Generation in Healthcare
In healthcare, generating synthetic data provides a new approach to handling sensitive data while prioritizing privacy and security. Let’s look at the ways to generate synthetic data, as well as data sources and the delicate balance between realism and confidentiality.
Algorithms and Techniques
The generation of synthetic healthcare data relies heavily on advanced algorithms and statistical techniques. You’ll find that these algorithms are specifically designed to replicate the patterns, distributions, and relationships discovered in real patient data. Several methods are commonly used:
- Statistical Sampling: In this method, you can draw samples from an existing dataset and then apply statistical techniques to create synthetic data that mirrors the characteristics of the original data.
- Generative Models: Machine learning models, such as Generative Adversarial Networks (GANs) and Variational Autoencoders (VAEs), have become prominent in creating synthetic data. GANs, for instance, consist of a generator and a discriminator that compete to produce exceptionally realistic synthetic data.
- Differential Privacy: This technique involves adding a layer of noise to real data when creating synthetic data. It’s a way to ensure privacy preservation, making it nearly impossible to identify any specific individual’s data within the synthetic dataset.
- Synthetic Data Generators: Synthetic data generators are specialized software and solutions that automatically generate synthetic healthcare datasets. These generators employ strategies, including those mentioned above, to generate data that meets specific privacy and statistical criteria.
Data Sources for Synthesis
Your success depends on the quality and diversity of the data sources you utilize to generate synthetic data for use in healthcare. Think about the following common data sources for synthesis:
- EHRs (Electronic Health Records): EHRs are synthetic data vaults storing complete medical histories, diagnosis, and treatment records. They provide a solid foundation for your synthetic datasets by serving as a major source for developing synthetic healthcare data.
- Medical Imaging Data: When building and testing image analysis algorithms, synthetic data for medical pictures such as X-rays, MRIs, and CT scans can be generated. This type of synthetic data is important for guaranteeing the quality and robustness of your medical imaging algorithms.
- Clinical Trials Data: You can use clinical trial data to test new therapies and interventions. These trials involve controlled tests with patient volunteers and can provide useful information for developing synthetic datasets customized to specific research objectives.
- Health Surveys and Public Health Data: You can take a look at population-level health surveys and public health data sources to increase the diversity and relevancy of your synthetic healthcare data. These databases provide useful information regarding overall health trends and demographics.
Balancing Realism and Privacy
Balancing realism and privacy is a critical challenge in developing synthetic data in healthcare. When working with synthetic health data, you must find a difficult balance between producing data that closely matches real patient data for relevant research and innovation and protecting individual privacy. Consider the following to achieve this balance:
- Noise Addition: You can add controlled levels of noise into the data. This noise makes it more difficult to re-identify individuals while keeping the data useful for study and analysis.
- Data Aggregation: A different strategy is to combine data at a higher level, such as a regional or institutional level. As a result, there is a lower chance of patient re-identification because the data is less specific.
- Evaluating Utility: It is essential to evaluate the utility of synthetic data regularly. This review guarantees that the data stays useful for research while protecting individual privacy. These factors must be balanced for synthetic data to be used ethically and effectively in healthcare research.
Use of Synthetic Data in Healthcare
In healthcare, synthetic data has a wide range of uses, each fulfilling a distinct purpose. Here, you’ll find several healthcare applications of synthetic data.
Research and Development
You can utilize synthetic datasets to examine medical conditions, treatment outcomes, and patient demographics without compromising patient privacy.
For example, suppose you’re studying the effects of a new treatment. In that case, synthetic data allows you to predict patient responses, refining your theories and testing methods before taking on resource-intensive clinical trials.
Algorithm Training and Validation
Algorithms are important in activities such as medical image processing and disease prediction in healthcare. Synthetic data provides a safe and secure environment for training and verifying these algorithms.
Suppose you’re developing an AI model for radiology. In that situation, you can use medical synthetic images to create a wide range of patient cases before applying your model to accurate patient information.
Medical Education and Training
If you are a medical teacher or student, synthetic data can help you with your training and education. You can provide synthesized health data to your students or trainees to let them practice diagnosing and treating virtual patients. This hands-on training improves their clinical knowledge and decision-making skills.
For example, medical students can hone their skills by working with fake patient records before treating actual patients.
Collaboration and Data Sharing
Due to privacy concerns and regulatory limits, healthcare organizations frequently face obstacles when sharing actual patient data. Synthetic data saves the day by allowing organizations to share synthetic datasets for cooperative R&D projects.
As a healthcare worker, you can find that this collaborative approach leads to development in areas such as medication discovery and disease epidemiology.
Epidemiological and Public Health Research
Synthetic data can be a game changer in epidemiology and public health research. It allows you to model various situations and analyze illness spread, intervention effects, and healthcare resource allocation while maintaining patient privacy.
For example, you can simulate various vaccination procedures and disease breakout scenarios using synthetic data.
Algorithm, hypothesis, and methods testing
As a researcher, it’s important to test new algorithms, theories, or research methodologies frequently. Synthetic data provides a controlled environment for conducting such tests.
For example, in cancer research, you can utilize synthetic patient data to test the accuracy of a new diagnostic algorithm before applying it to actual patient records.
Advantages of Synthetic Data
The advantages of using synthetic data in healthcare are significant, and it covers several areas of data-driven healthcare research, development, and practice. Here are the main benefits:
- Privacy Protection: One of the most critical advantages of synthetic data in healthcare is its capacity to protect patient privacy. You can protect patient information by using synthetic data. It allows you to work with data that appears to be patient data but does not reveal personal information.
- Compliance with Regulations: The healthcare industry is extensively regulated, and these regulations require strict compliance with data protection and privacy requirements. Synthetic data helps you comply with these standards by eliminating the usage of genuine patient data. It lowers the chance of legal and ethical violations.
- Research and Innovation: Synthetic data provides a secure healthcare research and development environment. You can perform tests, test theories, and develop new treatments and technologies without the ethical considerations that come with real patient data.
- Data Diversity and Balance: Real-world patient data can be biased or insufficient. You can use synthetic data to overcome bias issues and represent distinct patient populations.
- Risk Reduction: Synthetic data reduces the risks of using genuine patient data, such as data breaches, patient identity theft, and legal consequences. This risk reduction improves the safety and responsibility of healthcare data usage.
Challenges and Limitations
Let’s look at some of the challenges and limitations of using synthetic data in healthcare:
- Realism vs. Accuracy: Establishing a balance between realistic synthetic data and data accuracy is difficult. It should resemble real data but may not capture all complexity. This may affect the practicality of research or algorithms in healthcare.
- Bias in Synthetic Data: Synthetic data generation is based on existing data, which may be biased. If the original data has biases, your generated data might as well. Detecting and eliminating discrimination in synthetic data is a never-ending task.
- Ethical Considerations: While patient privacy is protected, ethical considerations may arise. You have to ensure that your usage of synthetic data follows ethical principles. Furthermore, ethical concerns may arise when using algorithms trained on synthetic data on real patient data.
- Validation and Generalization: It is critical to confirm that Synthetic data-based research findings and models are applicable to real-world scenarios. To avoid dependency on synthetic data, you must systematically evaluate how well your results translate to genuine clinical settings.
- Data Source Representativeness: The value of synthetic data depends on your source data’s accuracy. If the original data does not represent a full range of natural patient populations, your synthetic data may not adequately reflect all healthcare scenarios and patient demographics.
- Limited Historical Data: Long-term historical patient data is required in some healthcare applications. Due to the lack of historical data for synthesis, creating synthetic data that accurately reflects patient health histories can be challenging.
Synthetic Data in Clinical Trials
Synthetic data provides a solution by allowing you to design clinical trials without the need for actual patient data. It assures the protection of patient privacy while allowing you to complete your tasks. It enables you to simulate patient groups, which helps you to identify the optimal trial size to generate meaningful results. This method of planning trials is strategic and cost-effective.
Synthetic data enables you to test concepts and procedures without involving actual patients in the trial preparation process, including question formulation and data collection strategies. This safeguards the efficiency of your trial when you transition to real-world implementation.
Furthermore, synthetic data is a useful instrument for training purposes. You and your team can engage in practice sessions without the risks of using actual patient information. It encourages collaboration amongst researchers, facilitating mutual learning and knowledge sharing while alleviating privacy regulations-related concerns.
Conclusion
Synthetic data in healthcare is a crucial invention that addresses the complicated challenges of balancing data-driven advancements with patient privacy and data security. Its importance cannot be emphasized, as it provides a safe and ethical framework for healthcare research.
Researchers may interact across borders and institutions using synthetic data generated by AI trained on realistic data. It is one of the most adaptable tools with many use cases and a proven track record.
Synthetic data accelerates healthcare research and innovation by enabling quick algorithm training, eliminating bias, and encouraging cross-institutional collaboration. It links the increased demand for data-driven healthcare solutions and the need to protect patient privacy.
QuestionPro is a versatile survey and data collection platform that can be used to generate and refine synthetic data in healthcare. Its versatility, customization, data security, and analytical capabilities help researchers, healthcare providers, and organizations use synthetic data while protecting data.