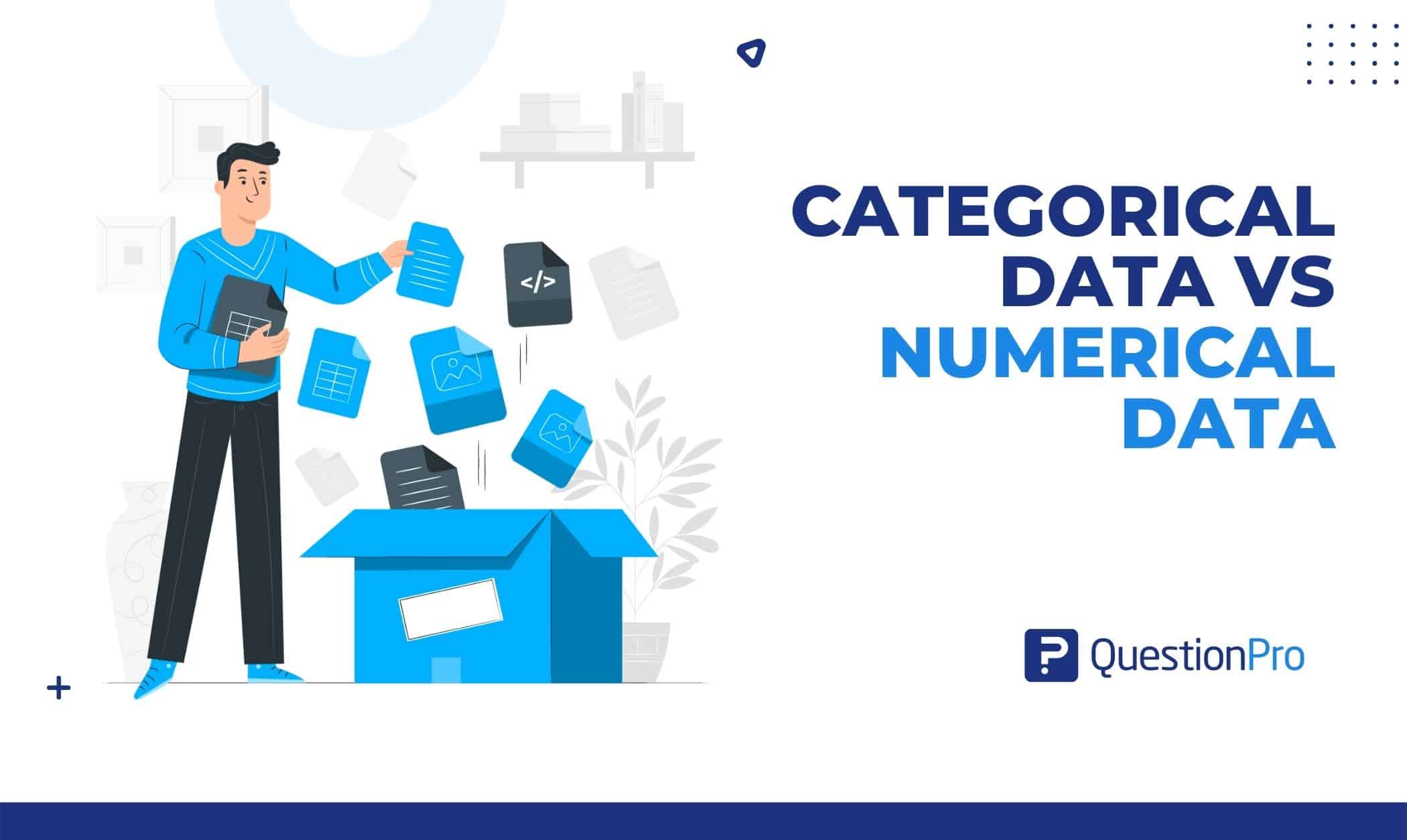
Data are facts or pieces of information gathered for reference or analysis. Most of the time, these data are collected as part of the subject being looked at. This attribute can be different for each person. Let’s talk about Categorical Data vs Numerical Data.
When researching and collecting data, it’s essential to know what kind of data you’re getting so you can interpret and analyze it well. Most of the time, there are two types of data in a research study:
- Categorical data
- Numerical data.
In statistics, it is vital to understand the different kinds of data. It is crucial to figure out who they both are based on how they are different and how they are the same. This will make it easy to gather, use, and analyze them correctly.
In this article, we will discuss what categorical data are and how they differ from numerical data. Let’s get started.
What is categorical data?
Categorical data can be put in groups or categories using names or labels. This grouping is typically generated using a matching procedure based on data attributes and similarities between these qualities.
Each piece of a categorical dataset, also known as qualitative data, may be assigned to only one category based on its qualities, and each category is mutually exclusive.
There are two primary categories of categorical data:
- Nominal data: This is the data category that names or labels its categories. It has features resembling a noun and is occasionally referred to as naming data.
- Ordinary data: Elements with rankings, orders, or rating scales are included in this category of categorical data. Nominal data can be ordered and counted but not measured.
What is numerical data?
Data expressed in numerical terms rather than in natural language descriptions are called numerical data. It can only be gathered in numerical form, keeping its name. This numerical data type also referred to as quantitative data can be used to measure a person’s height, weight, IQ, etc.
Numerical data can be of two types:
- Discrete Data: Countable numerical data are discrete data. They are mapped one-to-one to natural numbers, in other words. Age, the number of students in a class, the number of candidates in an election, etc., are a few examples of discrete data in general.
- Continuous Data: This is an uncountable data type for numbers. A series of intervals on a natural number line is used to depict them. Student CGPA, height, and other continuous data types are a few examples.
The difference between categorical data vs numerical data
Many things are different between these 2 types of data. Let’s find out what and how they are different below:
No | Features | Categorical data | Numerical data |
1 | Definition | Categorical data can be stored and identified by names or labels. | Numerical data are numbers, not words or descriptions. |
2 | Alias | Because it qualifies data before categorizing it, it is sometimes referred to as qualitative data. | Quantitative data represents numerical values for arithmetic processes. |
3 | Examples | Define gender.
|
Test score out of 20?
|
4 | Types | Nominal data and Ordinal data. | Discrete data and Continuous data. |
5 | Characteristics |
|
|
6 | User-friendly design | Long surveys are a possibility and may turn off responders. | Survey interaction is quick and short, reducing abandonment. |
7 | Data collection method |
|
Mostly multiple-choice, sometimes open-ended questions. |
8 | Data collection tools | Questionnaires, surveys, and interviews | Questionnaires, surveys, interviews, focus groups and observations |
9 | Uses | Used when a survey demands respondents personal information, opinions, and experiences. Used in business research | Statistical calculations based on arithmetic performance. |
10 | Compatibility | It’s incompatible with most statistical approaches. Thus researchers avoid it. | It supports most statistical calculations. |
11 | Visualization | Bar and pie charts alone. | Bar graphs, pie charts, and scatter plots can be used. |
12 | Structure | Unstructured data Like Google, Bing, etc., it can index data. | Since it is structured, it can be easily arranged and understood. |
Similarities between categorical data and numerical data
As we have already discussed the differences, the 2 following data have some similarities as well, which are described below:
-
Ordinal data
It is a cross between category and numerical data. Although it is commonly referred to as a subtype of categorical data, it can also be referred to as numerical data.
-
Uses
The results will be the same for research and statistical analysis whether you use a numerical or a categorical approach. Researchers sometimes use them both together in a survey to find out different ways to look at the data.
-
Collection tools
The most typical methods for gathering categorical and numerical data include surveys, questionnaires, and interviews.
The most popular method of data collecting employed by researchers is surveying. It may be made to collect both numerical and category data.
You can use yes/no questions or Likert scale questions to collect numerical data from your participants. Open-ended inquiries can also acquire essential data from the intended audience.
Conclusion
Depending on the research being conducted, categorical data vs numerical data may be used for statistical analysis. When tackling an issue, a researcher may decide to gather category data, numerical data, or even both in some circumstances.
When gathering information for an analysis to consider alternative viewpoints, the researcher may gather numerical and category data. To correctly use these two sorts of data in a study, one must be aware of their distinctions.
Another justification for why it’s critical to comprehend the various data kinds is provided by this.
QuestionPro is more than just survey software because it offers solutions for various problems and industries. For example, our InsightsHub research library is a data storage and analysis platform.
InsightsHub’s knowledge management solutions and platforms help companies enhance data management, speed insight development, and better leverage historical data while decreasing costs and enhancing ROI.