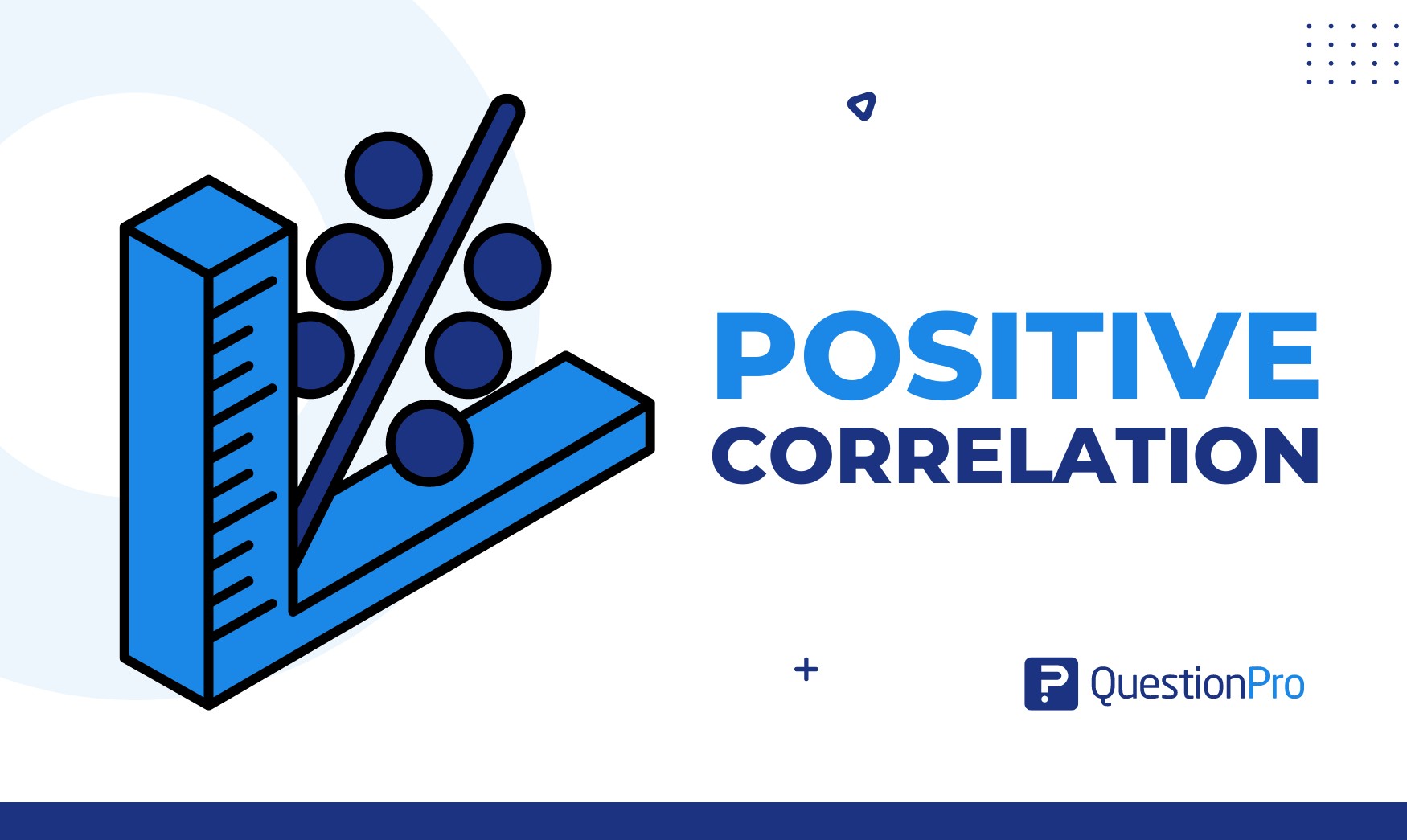
Correlation, the statistical measure of relationships between variables, illuminates how changes in one variable correspond with changes in another. Expressly, a positive correlation signifies that as one variable increases, so does the other, reflecting a direct connection.
Understanding this concept is pivotal across diverse domains, from economics to healthcare, as it enables predictions, informs strategic decisions, and drives advancements. This blog explains the definition of positive correlation, its significance in decision-making processes, and mechanics.
Discover how grasping positive correlation with the QuestionPro Research Suite can empower data-driven insights and enhance outcomes in various fields.
What is a Positive Correlation?
A positive correlation refers to a statistical relationship between two variables moving in the same direction. When one variable increases, the other tends to grow as well, and when one decreases, the other variable decreases.
This simultaneous movement indicates a direct connection between the two variables, and the strength of this relationship can be quantified using a measure called the correlation coefficient.
Correlation Coefficient:
The correlation coefficient, often represented by the symbol “r,” quantifies the degree to which two variables are related. It ranges from -1 to +1, with:
- +1 indicates a perfect positive correlation, where the variables move in complete harmony.
- 0 indicates no correlation, where the variables do not show consistent relationship patterns.
- -1 indicating a perfect negative (or inverse) correlation, where the variables move in opposite directions.
In the context of positive correlation, an “r” value closer to +1 suggests a strong positive relationship, meaning the variables closely follow each other’s changes.
For example, an “r” value of +0.9 indicates a strong positive correlation, whereas an “r” value of +0.2 indicates a weak positive correlation.
Importance of Understanding Positive Correlation
Understanding positive correlation is vital across multiple fields because it provides insights into how variables relate and helps make informed decisions.
- Predictive Power: Positive correlation allows us to predict one variable based on the changes in another.
For example, in finance, if an increase in consumer spending positively correlates with economic growth, analysts can predict future economic conditions based on spending trends.
This predictive ability is crucial for strategic planning and forecasting across industries.
- Strategic Decision-Making: In business, recognizing positive correlations can guide decisions such as budget allocations and marketing strategies.
If a company finds a strong positive correlation between its advertising expenditure and sales revenue, it can justify increased marketing spending to boost sales. This relationship also helps optimize resource allocation to areas that directly impact performance.
- Risk Management: Understanding positive correlations between different assets in investment allows for better portfolio management. Knowing which assets move in the same direction can help investors construct a diversified portfolio that minimizes risk while maximizing returns.
For instance, if two stocks are positively correlated, their prices tend to move together, indicating similar risk and return profiles.
- Scientific and Social Research: Identifying positive correlations can lead to significant discoveries in scientific research.
For example, studies may reveal a positive correlation between lifestyle choices and health outcomes, such as the relationship between regular exercise and longevity.
These insights can drive public health policies and personal choices that improve quality of life.
- Educational and Personal Development: Understanding positive correlations can influence behaviors and decisions on a personal level.
For instance, recognizing the positive correlation between study time and academic performance can motivate students to dedicate more time to their studies, leading to better grades and future opportunities.
How Does Positive Correlation Work?
A positive correlation describes a relationship between two variables moving in the same direction. Understanding how this concept works involves grasping its mechanics and implications across different scenarios.
1. Simultaneous Movement
When two variables exhibit a positive correlation, an increase in one variable tends to correspond with an increase in the other, and a decrease in one variable correlates with a reduction in the other. This simultaneous movement suggests that changes in one variable predict changes in the other, establishing a predictable pattern.
2. Correlation Coefficient
To quantify the strength and direction of a positive correlation, statisticians use a correlation coefficient denoted as “r.” This coefficient ranges from +1 to -1:
- A correlation coefficient close to +1 indicates a strong positive correlation, where the variables move closely together in the same direction.
- A coefficient of 0 suggests no correlation, meaning the relationship has no discernible pattern.
- A negative correlation coefficient (closer to -1) indicates an inverse relationship, where one variable moves in the opposite direction of the other.
3. Graphical Representation
Positive correlations are often depicted graphically using scatter plots. In these plots, each point represents a pair of values for the two variables. When plotted, data points tend to form a pattern where they cluster along an upward-sloping line. The closer the points are to creating a straight line, the stronger the positive correlation between the variables.
4. Real-World Examples
Examples of positive correlation abound in various fields:
- Economics: Consumer spending and economic growth often show a positive correlation, where increased spending stimulates economic activity.
- Healthcare: Studies may reveal a positive correlation between exercise and cardiovascular health, showing that increased physical activity correlates with improved heart health.
- Education: Time spent studying and academic performance typically correlate positively, with more study time correlating with higher grades.
5. Practical Applications
Understanding how positive correlation works is essential for decision-making in finance, marketing, healthcare, and beyond. Businesses use it to forecast demand, optimize resource allocation, and strategize marketing campaigns. Correlational research experts rely on it to identify patterns and relationships in data, leading to discoveries and advancements in various fields.
So, positive correlation elucidates how variables move together, providing predictive insights that inform strategic decisions and shape outcomes across industries and disciplines. By leveraging this understanding, individuals and organizations can harness data effectively to drive success and innovation.
Measuring Positive Correlation
Measuring positive correlation involves quantifying the strength and direction of the relationship between two variables. This process is essential in statistics and data analysis to understand how changes in one variable correspond to changes in another.
Correlation Coefficient (r)
The most common method to measure positive correlation is through the correlation coefficient, denoted as “r.” This numerical value ranges from +1 to -1:
- A correlation coefficient +1 indicates a perfect positive correlation, meaning the variables move in complete harmony. As one variable increases, the other also increases proportionally.
- A correlation coefficient of 0 indicates no correlation, suggesting that changes in one variable do not predict changes in the other.
- A correlation coefficient approaching +1 indicates a strong positive correlation, where the variables closely follow each other’s changes.
Calculation:
To compute the correlation coefficient for a set of data points:
- Step 1: Calculate the means (average values) of both variables.
- Step 2: Compute the deviations from the mean for each data point of both variables.
- Step 3: Multiply and sum these deviations pairwise for each data point.
- Step 4: Divide the summed value by the product of the standard deviations of both variables.
Interpreting the Coefficient
- A positive correlation coefficient indicates that as one variable increases, the other also tends to increase.
- The closer the correlation coefficient is to +1, the stronger the positive correlation.
- Scatter plots are often used to visually represent positive correlations, where data points cluster along an upward-sloping line.
Application
- In finance, measuring positive correlation helps investors understand how asset prices move together. Assets with a positive correlation may be risky to hold together in a portfolio during market downturns.
- In healthcare, positive correlation analysis can reveal relationships between lifestyle factors and health outcomes, guiding preventive strategies.
- Businesses use positive correlation measurements to optimize operations, such as correlating customer satisfaction scores with sales performance to enhance marketing strategies.
How Do You Determine a Positive Correlation?
Determining a positive correlation between two variables involves analyzing their relationship and quantifying the strength and direction of their association using statistical methods, particularly the correlation coefficient.
1. Collect Data
Firstly, gather data sets that include paired observations of the two variables of interest. For example, if examining the relationship between study hours and exam scores, collect data points where each pair represents the study hours and corresponding exam scores for individual students.
2. Visual Inspection
Create a scatter plot with one variable on the x-axis and the other on the y-axis. Plot each pair of data points as a single point on the graph. A visual inspection of the scatter plot can provide initial insights into the nature of the relationship. In the case of a positive correlation, the points on the graph will generally trend upwards from left to right.
3. Calculate the Correlation Coefficient (r)
To quantify the strength and direction of the relationship, calculate the correlation coefficient (r). The formula for r involves standardizing the covariance of the two variables by their respective standard deviations. The correlation coefficient ranges from -1 to +1:
- A correlation coefficient close to +1 indicates a strong positive correlation.
- A correlation coefficient of 0 suggests no linear relationship.
- A negative correlation coefficient (closer to -1) indicates a strong negative correlation.
4. Interpret the Correlation Coefficient
Once calculated, interpret the population correlation coefficient:
- If r is close to +1, it signifies a strong positive linear correlation.
For instance, if r = 0.8, it indicates that as one variable increases, the other tends to increase, with a high degree of consistency.
- If r is positive but closer to 0, it suggests a weaker positive correlation, where the variables move together but with more variability.
- Consider the context and the domain-specific knowledge when interpreting the correlation coefficient to avoid misinterpretations.
5. Statistical Significance:
Assess the statistical significance of the correlation coefficient to determine whether the observed relationship is likely to occur due to chance. This involves calculating the p-value associated with the sample correlation coefficient. A low p-value (typically less than 0.05) indicates that the observed correlation is statistically significant.
Positive Correlation vs. Negative Correlation
Understanding the differences between positive and negative correlation is essential in data analysis and decision-making. These concepts describe the direction and strength of relationships between variables, influencing how we interpret data and make predictions.
Positive Correlation:
In a positive correlation, as one variable increases, the other dependent variable also tends to increase. Conversely, as one decreases, the other tends to decrease.
- Graphical Representation: When plotted on a scatter plot, the points generally form an upward-sloping line, indicating the variables move together in the same direction.
- Examples:
- Height and Weight: Typically, taller individuals weigh more.
- Advertising Spend and Sales: More advertising usually leads to higher sales revenue.
- Implications: In business, recognizing positive correlations can guide where to invest resources for maximum return. In finance, understanding positive correlations helps forecast market movements.
Negative Correlation:
A perfect negative correlation describes a relationship where one variable increases as the other decreases.
- Graphical Representation: The scatter plot will show a downward-sloping trend, indicating that the other goes down as one variable increases.
- Examples:
- Exercise and Body Fat: Increasing exercise generally reduces body fat.
- Price and Demand: As the cost of a product goes up, demand typically decreases.
- Implications: Negative correlations are crucial for financial diversification strategies, as they can offset potential losses. In operations, understanding negative correlations helps identify areas where resources can be reallocated to maintain balance.
Example of Positive Correlation
Example: Study Hours and Exam Scores
Consider a scenario involving students’ study habits and exam performance. We want to examine the relationship between studying hours and exam scores.
Data:
Here is a small dataset illustrating this relationship:
Student Study Hours (X) Exam Score (Y)
A 2 70
B 4 75
C 6 80
D 8 85
E 10 90
Analysis:
- Scatter Plot: If we plot these data points on a scatter plot, with study hours on the x-axis and exam scores on the y-axis, we will notice that as study hours increase, exam scores also increase. The points will generally trend upwards from left to right.
- Correlation Coefficient: Calculating this dataset’s correlation coefficient (r) will yield a value close to +1, indicating a strong positive correlation. This means there is a direct and consistent relationship between study hours and exam scores: as one increases, so does the other.
Interpretation:
- Positive Correlation: The data shows a positive correlation between studying hours and exam scores. This relationship can be helpful for students, educators, and researchers to understand and predict performance outcomes based on study habits.
- Practical Implication: Students can use this information to plan their study schedules effectively, knowing that increasing study time will likely improve their exam scores.
This simple example demonstrates a positive correlation, where two variables, study hours and exam scores, move together in the same direction. Understanding such correlations can help make informed decisions and predictions based on observed data patterns.
How QuestionPro Research Suite Can Define Positive Correlation
The QuestionPro Research Suite offers robust tools and features that can effectively define and analyze positive correlations within datasets. Here’s how QuestionPro facilitates the understanding and utilization of positive correlation
Data Collection and Survey Design
QuestionPro allows researchers and analysts to design customized surveys and collect data efficiently. By structuring surveys to include variables of interest, such as consumer behaviors and preferences, researchers can gather comprehensive data sets necessary for correlation analysis.
Advanced Analytics and Statistical Tools
One of QuestionPro’s strengths lies in its advanced analytics capabilities. It provides built-in statistical tools enabling users to calculate variables’ correlation coefficients. Specifically, for positive correlation, these tools compute the correlation coefficient (r) to quantify the strength and direction of relationships.
Visualization and Reporting
QuestionPro simplifies data interpretation through intuitive visualization tools. Users can generate scatter plots and other graphical representations that depict positive correlations. These visualizations help identify trends and patterns within the data, such as the upward-sloping alignment of data points characteristic of positive correlations.
Insight Generation
Beyond basic correlation calculations, QuestionPro supports more profound insight generation. It allows users to conduct regression analyses and explore additional factors influencing the observed correlations. This capability is invaluable for researchers who understand the underlying mechanisms driving positive relationships between variables.
Analyzing Patterns and Trends
Through QuestionPro’s correlation analysis, researchers identify relationships between variables:
- Positive Correlation: Both variables move in tandem. For example, higher advertising spending correlates with increased sales.
- Negative Correlation: Variables move inversely. For example, more screen time correlates with lower academic performance.
- Zero Correlation: No discernible relationship exists. For example, years in school may not correlate with the number of letters in a person’s name.
Integration and Accessibility
The suite’s compatibility with various data sources and its cloud-based accessibility ensure that users can seamlessly integrate data from different platforms. This feature enhances the scalability and applicability of correlation analyses across diverse research settings and organizational needs.
Real-World Applications
Practical applications of QuestionPro’s correlation analysis capabilities span industries such as market research, academia, healthcare, and beyond. For instance, businesses can use it to correlate customer satisfaction scores with sales performance, while educators can analyze correlations between teaching methods and student outcomes.
Conclusion
A positive correlation signifies a direct relationship between two variables moving together in the same direction. This concept is fundamental in data analysis and helps predict outcomes, make informed decisions, and understand the intricate relationships within data sets.
Whether you’re analyzing financial markets, studying human behavior, or conducting scientific research, recognizing positive correlations is essential for drawing meaningful insights.
QuestionPro Research Suite simplifies defining and understanding positive correlations through robust data collection, analytical, and visualization capabilities.
By providing intuitive tools to measure, visualize, and interpret correlations, QuestionPro empowers researchers and businesses to make data-driven decisions, uncover valuable insights, and drive strategic actions based on their data.
Whether you’re a market researcher, academic, or business analyst, QuestionPro equips you with the tools to explore how variables interact positively and leverage these insights for growth and success.