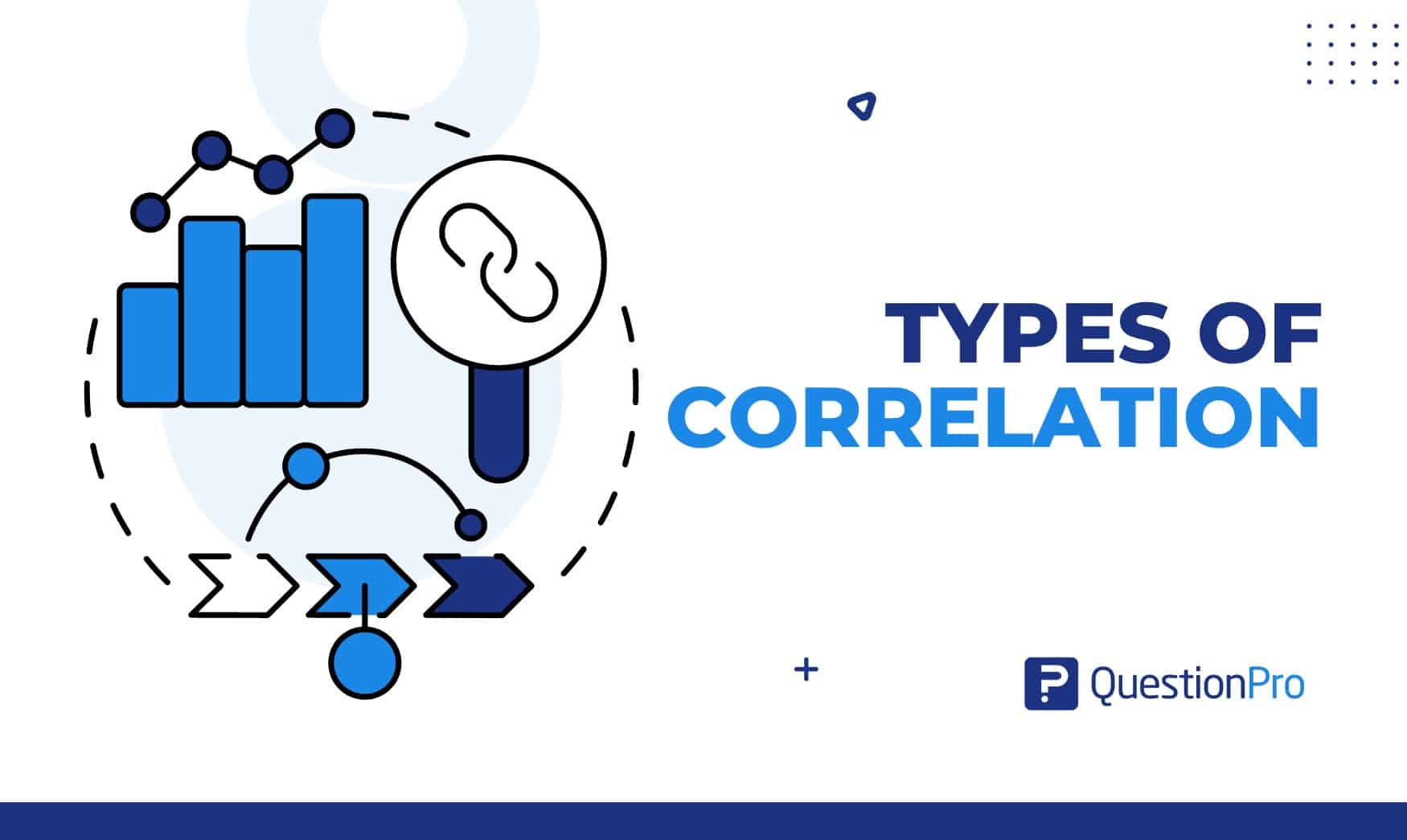
Have you ever wondered how two or more variables interact with each other? Correlation is the statistical measure that can help us understand this complex relationship. Join us as we unravel the mysterious types of correlation, positive, negative, and zero correlations, shedding light on their significance across various domains.
Whether you are a seasoned analyst or a curious learner, this blog promises insights that deepen your understanding and empower your decision-making processes. Let’s embark on this quest to decipher the language of correlation and uncover its secrets together.
What is a Correlation?
Correlation or correlational research is a statistical measure describing the extent to which two or more variables fluctuate. When the value of one variable changes, correlation measures how the value of another variable shifts in response.
Correlation can be positive, negative, or zero, indicating the direction and strength of the relationship between the variables. Understanding correlation helps research and data analysis by identifying patterns and relationships between variables, which can inform decision-making and predictions.
The Pearson correlation coefficient formula is commonly used to quantify the strength and direction of linear relationships between two variables. It represents one of the most widely recognized types of correlation measures in statistics.
Understanding whether a correlation is strong or weak is crucial for several reasons:
- Decision Making: Strong correlations can inform more confident decision-making, while weak correlations suggest that other factors might influence the outcome.
- Predictive Accuracy: Strong correlations provide more reliable predictions compared to weak correlations.
- Research and Analysis: Identifying the strength of correlations helps understand the underlying dynamics between variables and directs further research.
Types of Correlation
The population correlation coefficient formula is essential for calculating the strength and direction of linear relationships between variables in statistical analysis, elucidating the varying types of correlation present in datasets.
The linear correlation coefficient value, also known as the sample correlation coefficient, measures the strength and direction of the relationship between two variables in a dataset. There are several types of correlation coefficients commonly used to quantify the relationship between variables:
1. Positive Correlation:
A perfect positive correlation occurs when the values of two variables increase or decrease together. In other words, as one variable increases, the other variable also tends to increase, and vice versa. This is represented by a correlation coefficient (such as Pearson’s r) greater than 0.
Example: Consider a study examining the relationship between hours of study and exam scores. If there is a positive correlation between these variables, students who study more tend to achieve higher scores, and those who study less tend to achieve lower scores.
For instance, collecting data from a group of students and finding a Pearson correlation coefficient of +0.70 between hours of study and exam scores suggests a strong positive relationship. This means that exam scores also tend to increase as the number of study hours increases.
2. Negative Correlation:
A perfect negative correlation occurs when one variable increases while the other decreases, or vice versa. In other words, as one variable increases, the other tends to decrease, and vice versa. This is represented by a correlation coefficient (such as Pearson’s r) that is less than 0.
Example: Consider the relationship between outdoor temperature and hot chocolate sales. In this scenario, as outdoor temperature increases, sales of hot chocolate tend to decrease. Conversely, as outdoor temperature decreases, sales of hot chocolate tend to increase.
Collecting data and finding a Pearson correlation coefficient of -0.60 between outdoor temperature and hot chocolate sales indicates a moderate negative correlation. This means that as the outdoor temperature rises, the sales of hot chocolate tend to decrease.
3. Zero Correlation:
Zero correlation occurs when there is no relationship between two variables. In this case, changes in one variable are not associated with changes in the other. This is represented by a correlation coefficient (such as Pearson’s r) close to 0.
Example: Consider the relationship between shoe size and intelligence quotient (IQ) scores. There is likely no meaningful relationship between these two variables; having a larger or smaller shoe size does not indicate higher or lower intelligence.
If we collect data and find a Pearson correlation coefficient close to 0 (e.g., 0.05), it suggests that there is no significant correlation between shoe size and IQ scores. Knowing someone’s shoe size does not provide meaningful information about their intelligence level.
QuestionPro recently published a blog about correlation matrix. Explore to learn about it.
Uses Of Correlations
Correlation analysis is a powerful statistical tool used across various fields to identify and quantify the relationships between variables. Understanding these relationships can provide valuable insights and inform decision-making processes.
Here are some of the key uses of correlations:
1. Economics
- Market Analysis: Correlation helps economists understand the relationships between different economic indicators, such as inflation rates, unemployment rates, and GDP growth. For example, a strong negative correlation between unemployment and GDP growth can indicate that as economic output increases, unemployment tends to decrease.
- Investment Decisions: Investors use correlation to diversify their portfolios. By analyzing the correlation between different asset classes, investors can minimize risk. For instance, holding both can reduce portfolio volatility if stocks and bonds correlate negatively.
2. Healthcare
- Epidemiology: Correlation is used to study the relationship between various risk factors and health outcomes. For example, researchers may examine the correlation between smoking and lung cancer incidence.
- Treatment Efficacy: In clinical trials, correlations between treatment variables and patient outcomes can help determine the effectiveness of new medications or therapies. Strong correlations between treatment and positive health outcomes support the treatment’s efficacy.
3. Marketing
- Customer Behavior: Marketers use correlation to understand the relationship between marketing strategies and consumer behavior. For example, analyzing the correlation between advertising spend and sales revenue can help optimize marketing budgets.
- Market Research: Correlation analysis helps identify factors influencing customer satisfaction and loyalty. By examining the correlation between product features and customer satisfaction scores, companies can focus on features that matter most to their customers.
4. Education
- Academic Performance: Educators and researchers study the correlation between various factors and student performance. For instance, they might analyze the correlation between attendance rates and academic grades to identify the impact of class attendance on learning outcomes.
- Teaching Methods: Correlation can help evaluate the effectiveness of different teaching correlation methods. Educators can adopt more effective instructional approaches by examining the correlation between teaching strategies and student engagement or performance.
5. Social Sciences
- Behavioral Studies: Sociologists and psychologists use correlation to study relationships between behavioral variables. For example, the correlation between social media usage and self-esteem can provide insights into how online interactions affect mental health.
- Policy Making: Correlation analysis helps policymakers understand the potential impact of social policies. Policymakers can make more informed decisions by examining correlations between policy changes and social outcomes, such as crime rates or educational attainment.
6. Business and Management
- Operational Efficiency: Businesses use correlation to identify factors that influence operational efficiency. For example, analyzing the correlation between employee training hours and productivity can help optimize training programs.
- Customer Satisfaction: Correlation analysis helps businesses understand the factors driving customer satisfaction. Companies can improve their service strategies by examining the correlation between customer service quality and satisfaction ratings.
7. Environmental Studies
- Climate Research: Environmental scientists study the correlation between various environmental factors to understand climate change. For example, the correlation between carbon dioxide levels and global temperatures can highlight the impact of greenhouse gases on climate change.
- Pollution Control: Correlation helps identify pollution sources and their environmental impact. Effective control measures can be implemented by analyzing the correlation between industrial activities and pollution levels.
8. Technology and Innovation
- Product Development: Correlation analysis helps understand the relationship between product features and user preferences. For example, tech companies might analyze the correlation between user interface design and user satisfaction to enhance product usability.
- Research and Development: In R&D, correlation helps identify the relationship between different experimental variables and outcomes, facilitating innovation and improvement.
Strong Correlations vs. Weak Correlations
When analyzing relationships between variables, it is essential to understand the strength of the correlation. The strength of a correlation is measured by the correlation coefficient, which ranges from -1 to 1. This coefficient indicates how closely the variables are related to each other.
Strong Correlations
A strong correlation means that the variables have a robust and consistent relationship. Changes in one variable are closely associated with changes in the other variable. Strong correlations are indicated by correlation coefficients close to -1 or 1.
Characteristics of Strong Correlations:
- Correlation Coefficient: Typically between -0.7 and -1.0 or 0.7 and 1.0.
- Predictability: One variable can reliably predict the other.
- Graphical Representation: Points on a scatter plot will closely follow a straight line (linear) or a clear curve (non-linear).
Examples:
- Positive Strong Correlation: Height and weight in adults. Generally, taller people tend to weigh more.
- Negative Strong Correlation: Amount of time spent studying and the number of errors made on a test. More study time typically results in fewer errors.
- Visual Example:
Imagine plotting the relationship between the number of hours studied and exam scores. With a strong positive correlation, you will see points tightly clustered around an upward-sloping line.
Weak Correlations
A weak correlation means that the relationship between the variables could be stronger and more consistent. Changes in one variable are not closely associated with changes in the other variable. Weak correlations are indicated by correlation coefficients close to 0.
Characteristics of Weak Correlations:
- Correlation Coefficient: Typically between -0.3 and 0.3.
- Predictability: One variable does not reliably predict the other.
- Graphical Representation: Points on a scatter plot will be more dispersed and not follow a clear pattern.
Examples:
- Positive Weak Correlation: The number of hours spent on social media and academic performance. There might be a slight trend that more social media use correlates with lower academic performance, but it is not strong.
- Negative Weak Correlation: Daily coffee consumption and productivity levels. There might be a slight trend that higher coffee consumption correlates with slightly higher productivity, but it is not strong.
- Visual Example:
Imagine plotting the relationship between daily coffee consumption and productivity. With a weak correlation, the points will be scattered more widely around the graph, showing no clear pattern.
Advantages of Using Types of Correlation
Correlation analysis is a powerful statistical technique that measures the strength and direction of the relationship between two variables. Here are several advantages of using correlation:
- Simplicity: Correlation analysis is relatively simple to understand and apply, making it accessible to researchers and analysts with varying levels of statistical expertise.
- Identifying Relationships: Correlation helps identify and quantify relationships between variables, providing valuable insights into patterns and associations within datasets.
- Predictive Power: Strong correlations enable more accurate predictions. Researchers can more confidently forecast future trends and outcomes by understanding the relationship between variables.
- Data Reduction: Correlation analysis can streamline data by identifying highly correlated variables. This simplifies models and analyses by focusing on the most influential factors, improving efficiency and clarity.
- Foundation for Advanced Analysis: Correlation is a foundational tool for more complex statistical techniques such as regression analysis, factor analysis, and structural equation modeling.
- Diagnostic Tool: Correlation helps diagnose issues like multicollinearity, which occurs when independent variables are highly correlated. Detecting and addressing multicollinearity enhances the reliability of regression models.
Disadvantages of Correlation
While correlation analysis is valuable for understanding relationships between variables, it has several limitations and potential pitfalls. Here are some disadvantages of correlation analysis:
- Causation Misinterpretation: Correlation does not imply causation, leading to potential misinterpretation of relationships between variables.
- Outliers and Influences: It is sensitive to outliers, which can skew results and affect the accuracy of correlations.
- Non-linear Relationships: Correlation measures linear relationships and may not accurately capture non-linear relationships, limiting its applicability in specific scenarios.
- Spurious Correlations: It can identify meaningless correlations due to random chance or external factors, leading to false conclusions.
- Omitted Variable Bias: Missing important variables in the analysis can lead to biased correlations and inaccurate interpretations.
- Multicollinearity: A high correlation between independent variables in regression models can cause problems in estimating coefficients accurately and may affect model stability.
- Limited to Pairwise Relationships: Correlation analysis typically focuses on the relationship between pairs of variables, overlooking complex interactions involving multiple variables.
How QuestionPro Research Can Help in Defining the Types of Correlation
QuestionPro Research is a comprehensive tool for advanced data analysis, including correlation studies. Here’s how it can help:
1. Advanced Data Collection
QuestionPro provides robust data collection capabilities, enabling researchers to gather high-quality data from various sources. Accurate and comprehensive data is crucial for reliable correlation analysis.
2. Data Management
QuestionPro offers powerful data management tools that help clean and organize data, ensuring that the datasets used for correlation analysis are accurate and free from errors or inconsistencies.
3. Statistical Analysis Tools
QuestionPro includes built-in statistical analysis tools to calculate rank correlation coefficients and perform other statistical tests. These user-friendly tools allow even those with limited statistical knowledge to conduct sophisticated analyses.
4. Visualization
The platform offers advanced data visualization options, including scatter plots and heatmaps, which help visually identify and interpret correlations between variables. Effective visualization makes it easier to communicate findings to stakeholders.
5. Custom Reports
QuestionPro allows users to generate custom reports highlighting key findings from correlation analyses. These reports can be tailored to specific audiences, ensuring the insights are presented clearly and impactfully.
6. Integration with Other Tools
QuestionPro integrates with various other tools and software, facilitating the import and export of data for more extensive analysis. This interoperability enhances the analytical capabilities and allows for seamless workflow integration.
7. Expert Support
QuestionPro provides access to a team of research experts who can offer guidance and support in designing studies, analyzing data, and interpreting results. This expert assistance ensures that the correlation analyses are robust and reliable.
Conclusion
Understanding types of correlation is essential for practical data analysis and informed decision-making. Correlation analysis helps identify and quantify relationships between variables, providing valuable insights across multiple fields.
While it offers several advantages, such as simplicity and predictive power, it also has limitations, including the potential for misinterpreting causation and sensitivity to outliers.
QuestionPro Research is an invaluable tool for conducting correlation analyses. It offers advanced data collection, management, and analysis capabilities.
With its user-friendly interface, powerful statistical tools, and expert support, QuestionPro Research assists researchers in defining and interpreting types of correlation, enabling them to make confident, data-driven decisions.
By leveraging these tools, researchers can uncover meaningful patterns and relationships in their data, leading to better outcomes and more effective strategies.