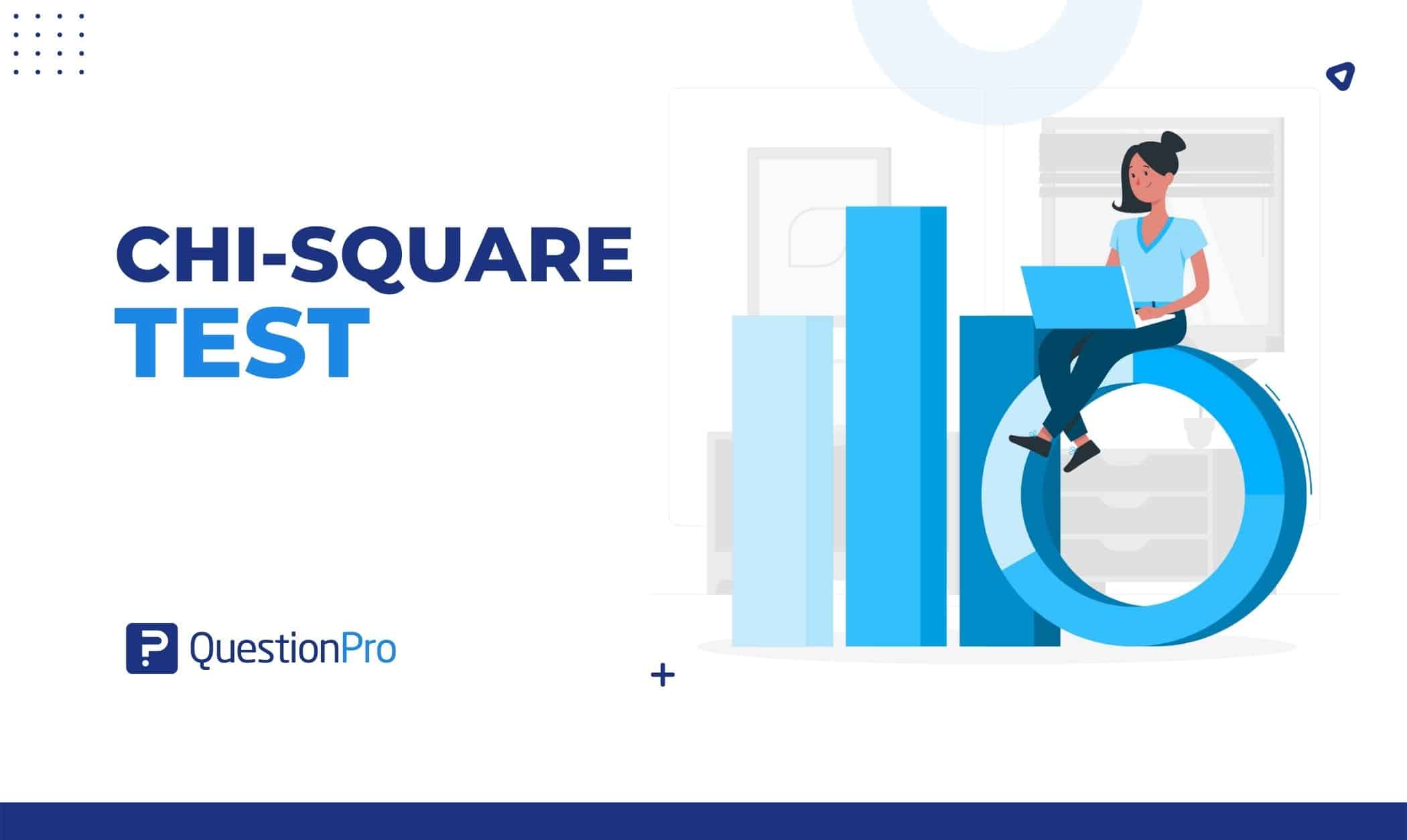
The chi-square test is often referred to as Fisher’s exact test. It is a statistical test used to examine categorical data. This article will explain the chi-square test and how to calculate it.
What is the chi-square test?
The chi-square test is a statistical way of determining differences between what was expected and what was seen in one or more categories.
Researchers utilize this nonparametric test to compare categorical variables within the same sample population. It also helps verify or provide background for frequency counts.
The basic idea behind the test is to examine the actual data values to determine what would be anticipated if the null hypothesis was correct.
Types of chi-square test
There are different types of chi-square tests that are frequently used:
- Goodness of fit test: It is a statistical test to detect if a variable comes from a particular distribution.
- Independence test: It is an inferential statistical test that draws conclusions about a population from a sample.
- Homogeneity test: It’s structured and runs like the independence test.
The independence test searches for a link between two categorical variables within the same population, while the homogeneity test checks whether a variable’s distribution is the same across populations.
Chi-square test in research
The Chi-square test makes it simple to comprehend and analyze the relationship between two categorical variables. Let’s examine the usefulness of this test to the research.
- The researcher can determine whether the observed cell counts are significantly different from the expected cell counts by statistically calculating Chi-Square and comparing it to a critical value of the Chi-Square distribution.
- It is sensitive to how cells are distributed. By consistently using categorical variables with a small number of categories, this problem can be solved.
Learn About: 180 vs 360 Feedback
Chi-Square test Perform guidelines
Now that you are more familiar with the Chi-Square test, let us show you how to perform it:
- Before beginning the data-gathering process, specify your null and alternative hypotheses.
- Set alpha. This includes assessing how risky an incorrect conclusion is. Assume alpha = 0.05 for independence testing. In this scenario, you’ve chosen a 5% risk of assuming that two variables are independent when they’re not.
- Verify the data for mistakes.
- Verify the test’s underlying assumptions.
- Get your test results.
How to calculate chi-square: The formula
The chi-squared test is used to determine whether the observed value and expected value differ in any way. The chi-square formula is represented by the following;
X2 = ∑(O – E)2/E
Here,
- O is the observed value
- E is the expected value
Chi-Square test example for categorical data
Let’s look at a society with 2000 residents divided into the four neighborhoods of A, B, C, and D as an example of categorical data.
1300 members who work in government, private, and doctors are chosen randomly. According to the null hypothesis, a person’s professional division is unrelated to the neighborhood in which they reside. The information is divided into
Categories | A | B | C | D | Total |
Government service | 180 | 120 | 208 | 190 | 698 |
Private Service | 60 | 100 | 102 | 40 | 302 |
Doctor | 60 | 80 | 90 | 70 | 300 |
Total | 300 | 300 | 400 | 300 | 1300 |
To determine what percentage of the 2,000 people reside in neighborhood A, assume that the sample population of 300 is in area A.
Similarly, we take 698/1300 to determine what proportion of the 2,000 comprises government employees. According to the independence presumption in the hypothesis, we should “expect” the following number of government employees in neighborhood A: 300 x (698 / 1300) = 161.08
Therefore, using the chi-square test formula for that specific table cell A, we obtain;
(Observed value – Expected value)2 / Expected value = (180 – 161.08)2 / 161.08 = 2.22
Conclusion
In this section, we looked at the chi-square test and how to perform and calculate it. Because it applies to categorical variables, the chi-square test is most commonly used by researchers studying survey response data. This research includes demographics, consumer and marketing research, political science, and economics.
The QuestionPro research suite is a set of tools that can be used to make the most of research and change experiences. At QuestionPro, we give all kinds of researchers tools for collecting data, like our survey software and insight repositories for all sorts of long-term research.
Talk to the QuestionPro team of experts if you need help researching how to make data-based decisions. QuestionPro can help you get the most out of your data and guide you through the process. Create a free account, or let us know what you need!