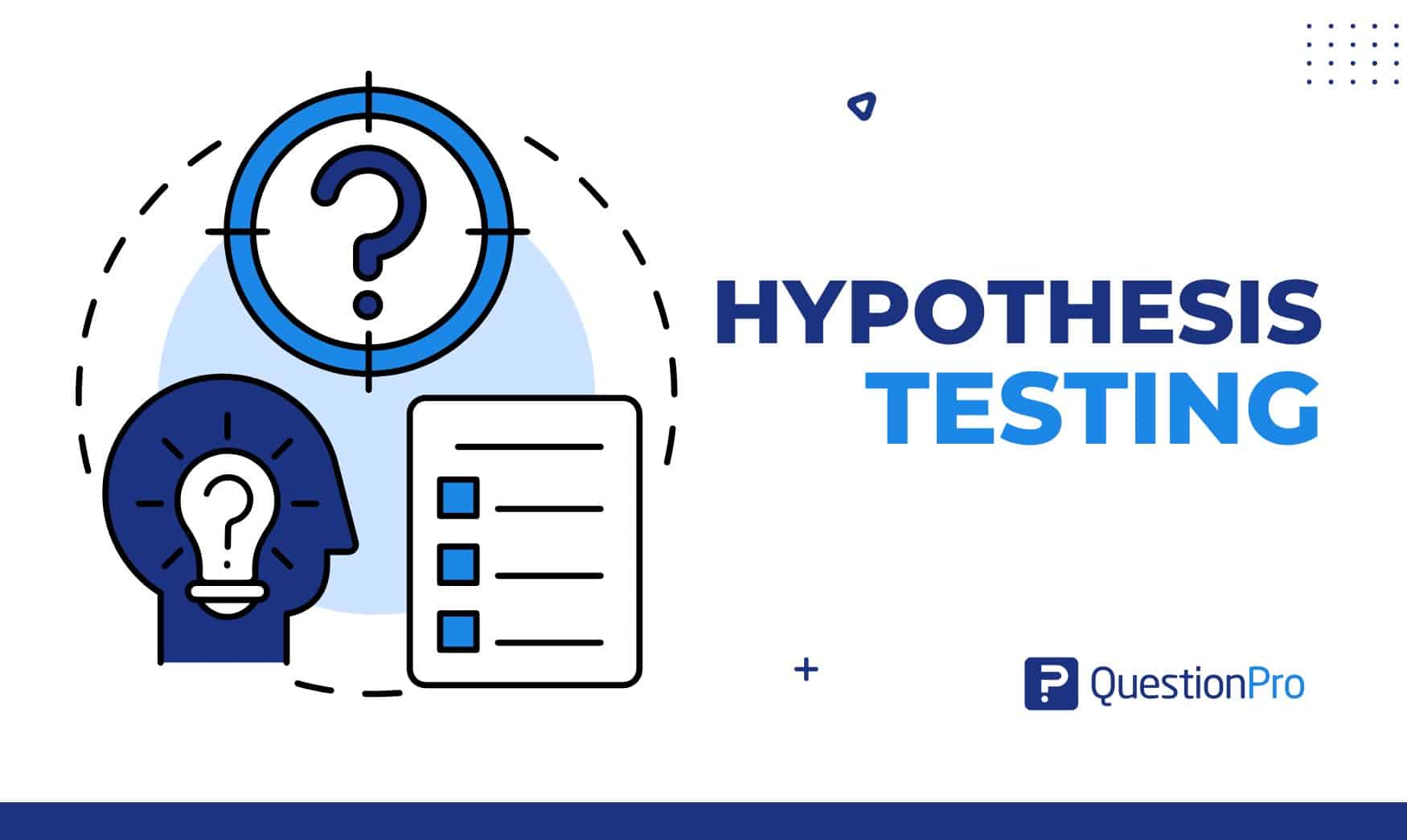
Have you ever faced a situation where you needed to decide based on data but weren’t sure how to confirm your assumptions? Hypothesis testing is the key to resolving such dilemmas. It’s a systematic way to analyze data and draw meaningful conclusions. Researchers make it an essential tool in research hypothesis, business, and everyday problem-solving.
In this blog, we’ll simplify everything you need to know about hypothesis testing. From understanding the basics to exploring real-world examples, we’ll make it easy for you to grasp and apply this powerful method. Ready to uncover the facts? Let’s begin!
What is Hypothesis Testing?
Hypothesis testing is a statistical method used to determine whether there is enough evidence in a sample of data to support or reject a specific assumption (hypothesis) about a population. It involves formulating a null hypothesis (usually indicating no effect or no difference) and an alternative hypothesis, then analyzing sample data to decide whether the evidence is strong enough to reject the null hypothesis in favor of the alternative.
Hypothesis testing helps you decide if your guess about something is backed up by evidence. It’s used in all sorts of fields, like science, business, and healthcare, to make decisions based on data.
Importance of Hypothesis Testing
Hypothesis testing is really important because it helps us make decisions based on data rather than just guessing or assuming things. It’s a way to test whether an idea or theory is likely to be true using real evidence. Here’s why it’s so important:
- Making Data-Driven Decisions: In business, science, and everyday life, decisions are often made using data. Hypothesis testing helps make those decisions more reliable because it turns guesses into evidence-based conclusions.
- Reducing Risk: When making decisions, especially in areas like medicine or business, it’s important to avoid making mistakes. Significance testing helps reduce the risk of making incorrect conclusions. For example, in medicine, it ensures that a new drug is truly effective before it’s widely used.
- Providing Clear Answers: Sometimes, we have many possible explanations for a situation. Hypothesis testing helps narrow down these possibilities and give us a clear answer. It helps us decide whether something is happening by chance or if there’s something real behind it.
- Building Confidence in Results: By using hypothesis testing, we can say with confidence whether our results are reliable. This builds trust in research findings and helps others believe in the conclusions.
- Improving Problem-Solving: Statistical significance testing helps identify patterns or causes that we might not have considered. It’s like a tool for digging deeper into problems and finding solutions that are supported by evidence.
Types of Hypothesis Testing
Hypothesis testing is a crucial tool in statistics that helps us make decisions based on data. It allows us to test assumptions and theories to see if they hold up in the real world. There are different types of hypothesis tests, and each is used for specific situations. Let’s break down the main types in a simple way so you can understand how they work and when to use them.
1. One-Sample Test
A one-sample hypothesis test is used when we want to test a claim or assumption about a single group. For example, let’s say a teacher believes that the average score of her students on a test is 75. To test this, they can collect the scores of a sample of students and perform a hypothesis test to see if the average score really is 75.
- Null Hypothesis (H₀): The average score is 75.
- Alternative Hypothesis (H₁): The average score is not 75.
If the data shows a significant difference from 75, the null hypothesis is rejected.
2. Two-Sample Test
The two-sample test compares the means of two different groups to see if there is a significant difference between them. This test is useful when you want to compare two groups, like checking if the average test scores of students from two different schools are different.
- Null Hypothesis (H₀): The average scores of both schools are the same.
- Alternative Hypothesis (H₁): The average scores of the two schools are different.
If the test shows a significant difference, the null hypothesis is rejected.
3. Paired Sample Test
A paired sample test is used when you want to compare two sets of related data. This usually happens when you are measuring the same group of people or things at two different times. For example, testing the effect of a new diet on the same group of people before and after the diet.
4. Chi-Square Test
The Chi-square test is used when you’re dealing with categorical data (data that falls into categories, like “yes” or “no”). It helps to see if there’s a significant relationship between two variables. For example, you might use a Chi-square test to see if gender and voting preferences are related.
- Null Hypothesis (H₀): There is no relationship between gender and voting preferences.
- Alternative Hypothesis (H₁): There is a relationship between gender and voting preferences.
This test checks if the frequencies of different categories differ significantly from what we expect.
5. ANOVA (Analysis of Variance)
ANOVA is used when you want to compare the means of three or more groups. It’s like the two-sample test but for more than two groups. For example, if you want to compare the average test scores of students from three different teaching methods, ANOVA can help you determine if there’s a significant difference.
6. Z-Test and T-Test
Z-tests and T-tests are two common types of hypothesis tests used to compare means, but they’re used in different situations.
- Z-Test: Used when the sample size is large (usually over 30) and the population standard deviation is known.
- T-Test: Used when the sample size is small (less than 30) or the population standard deviation is unknown.
Both tests help you compare sample data to a population mean or compare two sample means to each other.
7. One-Tailed vs. Two-Tailed Test
This is more about how you set up your hypothesis rather than a separate type of test.
- One-Tailed Test: This test is used when you are looking for evidence of a specific direction (e.g., is the average score greater than 50?).
- Two-Tailed Test: This test is used when you are looking for any significant difference, either greater or smaller (e.g., is the average score different from 50, but not specifying which way?).
8-Steps Hypothesis Testing Guide
Here, we’ll walk through the steps of hypothesis testing in an easy-to-understand way.
Step 1. State the Hypotheses
The first step in hypothesis testing is to define your hypotheses. There are two key hypotheses in every test:
- Null Hypothesis (H₀): This is the default assumption that there is no effect, difference, or relationship. For example, if you’re testing whether a new teaching method improves student scores, your null hypothesis might be: “The new teaching method has no effect on students’ scores.”
- Alternative Hypothesis (H₁): This is what you want to test for, the opposite of the null hypothesis. It suggests that there is an effect or a difference. For example, “The new teaching method improves students’ scores.”
Step 2. Set the Significance Level (α)
Next, you need to decide on the statistical significance level, commonly denoted as α. This represents the probability of rejecting the null hypothesis when it’s actually true (also called a Type I error). A common choice for α is 0.05 (5%), meaning you’re willing to accept a 5% chance of making an error.
In simpler terms, this is the threshold at which you’ll say, “Yes, there’s enough evidence to reject the null hypothesis.” If your test results show a probability lower than this threshold, you can reject the null hypothesis.
Step 3. Choose the Appropriate Test
Depending on the type of data you have and the question you’re asking, you’ll choose the correct statistical test. For example, you might use:
- T-tests are used to compare the means of two groups.
- ANOVA is used to compare the means of three or more groups.
- Chi-square tests for categorical data.
- Z-tests for large sample sizes.
Choosing the right test ensures that your results are reliable and valid for your specific situation.
Step 4. Collect and Analyze Data
Once you’ve set your hypotheses and chosen the test, the next step is to collect your data. This could involve surveys, experiments, or existing datasets. Make sure your data is reliable and relevant to the hypothesis you’re testing.
After collecting the data, you’ll perform the statistical test. This could involve calculating means and variances or using statistical software to compute the results. The goal here is to get a test statistic (like a t-value, z-value, or chi-square statistic) that you can use to make a decision about the null hypothesis.
Step 5. Calculate the p-value
The p-value tells you how likely your results are, assuming the null hypothesis is true. It’s a crucial step in statistical hypothesis testing.
- Low p-value (< 0.05): If the p-value is lower than your significance level (e.g., 0.05), it suggests that the results are unlikely to have happened by chance, and you can reject the null hypothesis. In other words, there is enough evidence to support the alternative hypothesis.
- High p-value (> 0.05): If the p-value is higher than the significance level, you fail to reject the null hypothesis. This doesn’t prove that the null hypothesis is true, but it means there isn’t enough evidence to say it’s false.
Step 6. Make a Decision
Now that you have the p-value, you can make your decision about the null hypothesis. There are two possible outcomes:
- Reject the Null Hypothesis (H₀): If your p-value is small (less than α), you reject the null hypothesis and accept the alternative hypothesis. This means the evidence suggests something statistically significant is happening, such as a real effect or difference.
- Fail to Reject the Null Hypothesis: If your p-value is large (greater than α), you fail to reject the null hypothesis. This doesn’t mean the null hypothesis is true; it just means there isn’t enough evidence to support the alternative hypothesis.
Step 7. Draw a Conclusion
Once you’ve made your decision, it’s time to draw your conclusion and interpret the results. For example, if you reject the null hypothesis, you might conclude that the new teaching method does improve student scores. If you fail to reject the null hypothesis, you would conclude that there’s not enough evidence to say the new method works better.
Remember, failing to reject the null hypothesis doesn’t mean the hypothesis is true. It simply means there wasn’t enough evidence to prove it wrong with the data you have.
Step 8. Report the Results
Finally, you’ll report the results of your hypothesis test. This includes:
- The hypotheses you tested.
- The significance level (α) you chose.
- The test you used.
- The p-value and the decision (reject or fail to reject the null hypothesis).
- A conclusion based on your findings.
Clear communication of the results is essential, especially if you’re presenting your findings to others, whether it’s in a research paper, a business report, or a scientific study.
Hypothesis Testing Example
Here, we’ll go through a real-world example of hypothesis testing so you can see how it works in action. Don’t worry; we’ll keep it simple and easy to follow.
Let’s say you’re a restaurant owner, and you’ve introduced a new dessert on the menu. You want to find out if this new dessert increases customer satisfaction compared to your old dessert. Here’s how hypothesis testing would work in this situation:
Step 1: State the Hypotheses
- Null Hypothesis (H₀): The new dessert does not improve customer satisfaction (there’s no difference).
- Alternative Hypothesis (H₁): The new dessert improves customer satisfaction.
Step 2: Collect Data
You ask 50 customers who tried the old dessert and 50 customers who tried the new dessert to rate their satisfaction on a scale of 1 to 10.
Step 3: Choose a Significance Level
You decide on a significance level of 0.05 (this means you’re okay with a 5% chance of concluding something is true when it’s not).
Step 4: Analyze the Data
You calculate the average satisfaction score for both desserts and use statistical tools like a t-test to compare them.
- Old dessert average score: 7.2
- New dessert average score: 8.5
- The t-test gives you a p-value of 0.02.
Step 5: Make a Decision
Since the p-value (0.02) is less than the significance level (0.05), you reject the null hypothesis.
The data suggests the new dessert does improve customer satisfaction! You can confidently keep it on the menu. This is how hypothesis testing helps you make data-driven decisions in a simple and logical way.
How QuestionPro Helps in Hypothesis Testing?
With QuestionPro, the hypothesis testing process becomes easy, quick, and reliable. Let’s see how QuestionPro makes hypothesis testing simple for everyone.
1. Easy Data Collection
The first step in hypothesis testing is collecting data, and QuestionPro makes it super simple. You can create surveys that match exactly what you need.
- Multiple Question Types: Use multiple-choice, rating scales, or open-ended questions.
- Smart Logic: Customize the survey flow with skip logic and branching.
- Language Options: Create surveys in different languages to reach more people.
2. Organize Data Easily
After collecting responses, you need to sort and organize the data. QuestionPro helps you segment data effortlessly. You can group responses by age, location, or any other category to focus on what matters.
3. Get Real-Time Results
With QuestionPro’s live dashboard, you can see responses as they come in. This helps you quickly spot trends and patterns without waiting for the survey to close.
- Visual Charts: See results in graphs and charts instantly.
- Quick Insights: Spot important trends as soon as they appear.
4. Analyze Data Like a Pro
QuestionPro gives you tools to analyze data right in the platform. For advanced testing, you can export data to software like Excel or SPSS.
- Built-In Tools: See patterns and trends directly on QuestionPro.
- Data Export: Download your data to perform tests like t-tests or ANOVA.
5. Create Clear Reports
Once your data analysis is done, QuestionPro helps you create reports that are easy to understand. These reports are perfect for sharing with teams or stakeholders.
Conclusion
Hypothesis testing is essential for making a statistical inference about populations based on sample data. By following the systematic steps of hypothesis formulation, selection of tests, and interpretation of results, researchers can draw valid conclusions about their data.
QuestionPro ensures your hypothesis testing is efficient, accurate, and actionable, helping you make decisions backed by reliable data. Ready to dive into hypothesis testing? Start small, follow the steps, and let data guide your decisions! Contact QuestionPro for detailed information!