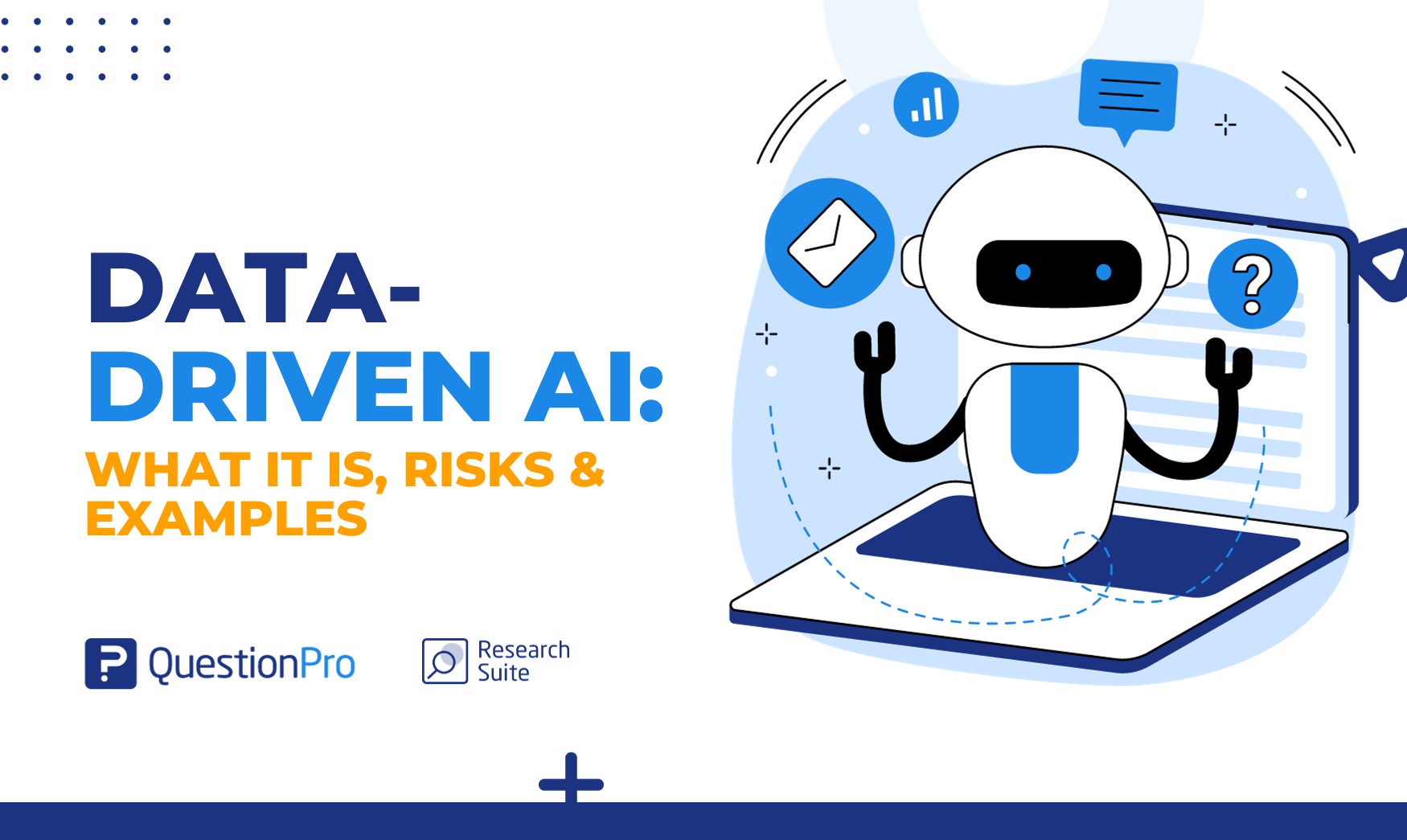
It’s fascinating to think that every moment you spend online, from that Google search to scrolling through social media, is feeding the maw of data-hungry AI algorithms. Indeed, the rapid growth of data-driven AI has taken center stage in numerous industries, from healthcare to finance, revolutionizing how we understand information, make decisions, and create value.
A simple yet profound concept fuels this surge in data-driven AI: data is the lifeblood of AI. The more data these algorithms consume, the more proficient they become at understanding, predicting, and delivering personalized solutions. But beneath this promise lies a complex world of challenges, from ethical concerns to data quality and privacy questions.
In this article, we’ll delve into the intricacies of it, exploring what it is, its risks, and how it’s transforming various industries. We’ll also compare it to model-driven AI, weigh the pros and cons, and even see how to harness the power of QuestionPro’s Research Suite in this AI-driven age.
What is Data-Driven AI
Data-driven AI is all about one thing: learning from data. It’s the practice of developing AI models that make decisions, predictions, or recommendations based on large volumes of data. Unlike traditional rule-based systems, where algorithms are explicitly programmed, data-driven AI excels at learning patterns, relationships, and behaviors from the data it encounters.
Consider recommendation engines like Netflix’s, which leverage your past viewing habits and the habits of other users to suggest your next binge-worthy series. Behind the scenes, its algorithms analyze massive datasets to understand your preferences, ultimately enhancing your streaming experience.
Risks and Mitigations of Data-driven AI
The rapid rise of data-driven AI comes with a fair share of risks. As these algorithms process vast datasets, privacy concerns loom large. Take social media platforms, for instance, which often find themselves in hot water for mishandling user data. Transparency and strong privacy measures are critical to mitigate these risks.
Another challenge is data quality. AI models trained on incomplete or biased data can perpetuate biases or generate inaccurate results. It’s essential to ensure that data used for training AI systems is diverse, representative, and regularly audited.
The ethical aspects of AI are increasingly important. Decisions made by AI can have profound implications, from hiring processes to medical diagnoses. Ensuring fairness and accountability in AI models is paramount.
Data-driven AI Examples
AI is omnipresent today. In the healthcare industry, it’s transforming disease diagnosis and drug discovery. In finance, AI algorithms analyze stock market data for trading insights. Even in agriculture, AI-powered precision farming optimizes crop yields based on weather, soil, and historical data.
An example that resonates with many is voice recognition. Voice assistants like Siri and Alexa understand and respond to your voice commands by continuously analyzing the data generated by your interactions. These assistants learn and adapt to better understand you over time, showcasing the power of data-driven AI.
Model-Driven AI vs Data-Driven AI
Unlike data-driven AI, model-driven AI relies more on predefined rules and logic. It’s based on human-crafted models that explicitly encode the knowledge required for tasks. These models may not require as much data to perform well but might lack their adaptability and generalization capabilities.
One typical example of model-driven AI is traditional expert systems used in industries like finance for rule-based decision-making. They operate based on a set of predefined rules.
The choice between model-driven and data-driven AI depends on the specific use case and data availability. The first shines in scenarios where ample data is available and can be used to uncover complex patterns.
Data-driven AI Pros and Cons
Data-driven AI’s pros are compelling: it can extract insights from massive and complex datasets, make personalized recommendations, and adapt to changing circumstances. These capabilities have led to breakthroughs in many fields.
However, the cons include the need for extensive data, concerns about privacy, and the potential for biases in the training data. The black-box nature of some models can also be challenging, making it difficult to explain their decision-making processes.
Pros
- Unparalleled Insights: Data-driven AI models can analyze vast amounts of data to uncover patterns and trends that might be impossible for a human to discern. This capability gives businesses a goldmine of insights into customer behavior, market dynamics, and operational efficiency.
- Personalization: In sectors like e-commerce, entertainment, and content delivery, data-driven AI excels at personalization. It can tailor recommendations, advertisements, and content to individual users based on their preferences, past behavior, and demographics. This personal touch significantly enhances user experience.
- Scalability: Data-driven AI can scale with data volume. AI models can continue to learn and adapt as more data becomes available. This scalability is crucial in a world where data generation is growing exponentially.
- Real-time Decision Making: Data-driven AI can make real-time decisions based on incoming data streams. This capability is vital in applications like fraud detection, autonomous vehicles, and healthcare monitoring systems, where quick decisions can make a significant difference.
- Consistency: AI models are consistent and can tirelessly perform repetitive tasks without getting tired or making errors. This is valuable in processes that require precision and reliability, such as quality control in manufacturing.
Cons
- Data Quality Dependency: The effectiveness of it hinges on the quality of the training data. If the data is inaccurate, incomplete, or biased, it can lead to flawed results. Ensuring data quality is a considerable challenge.
- Privacy Concerns: The hunger for data raises significant privacy concerns. As it collects and analyzes personal information, there’s a fine line between enhancing user experience and intruding on privacy. Striking a balance is essential.
- Ethical Issues: Data-driven AI can inadvertently perpetuate biases present in the training data. For example, if historical data reflects gender or racial biases, the AI model may replicate these biases in decision-making. This raises important ethical questions.
- Black Box Problem: Many models are considered “black boxes,” meaning it’s challenging to understand how they arrive at their decisions. This lack of transparency can be problematic, especially in critical applications with clear decision rationale.
- Data Quantity and Cost: Training AI models often requires massive amounts of data, which can be expensive and time-consuming to acquire and process. This can be a barrier to entry for smaller businesses.
- Human Labor: While AI can automate many tasks, it often requires human oversight. This human labor can include cleaning and labeling data, explaining model decisions, and ensuring the AI operates ethically.
Understanding these pros and cons is crucial for businesses and organizations looking to harness the power of data-driven AI while mitigating its potential downsides. The key lies in responsible and ethical AI development, ensuring that data quality is maintained, privacy is respected, and biases are actively addressed. As the AI landscape evolves, striking this balance will become increasingly critical.
QuestionPro for your Data-Driven AI
QuestionPro’s research suite can integrate well with your AI initiatives. Leveraging QuestionPro’s comprehensive survey and research tools, you can efficiently collect the data required for your AI projects. Our platform’s analytics capabilities can help you derive valuable insights from your data, making it a valuable companion for your data-driven AI endeavors.
Additionally, the feedback and insights you gather with QuestionPro can be used to fine-tune and validate AI models, ensuring they align with the needs and expectations of your target audience. Combining data collection and analysis tools in QuestionPro can be valuable in your AI-driven decision-making processes.
Conclusion
In the era of data-driven AI, the power of algorithms to transform industries and provide meaningful insights is unparalleled. However, the challenges are also significant, from privacy concerns to data quality and ethical considerations.
By understanding these challenges and harnessing the capabilities of platforms like QuestionPro, we can embark on this data-driven journey more responsibly, ensuring that AI’s remarkable potential is realized while safeguarding its ethical and practical aspects. With data as the AI and fuel as the engine, the possibilities are boundless, and the future is data-driven.