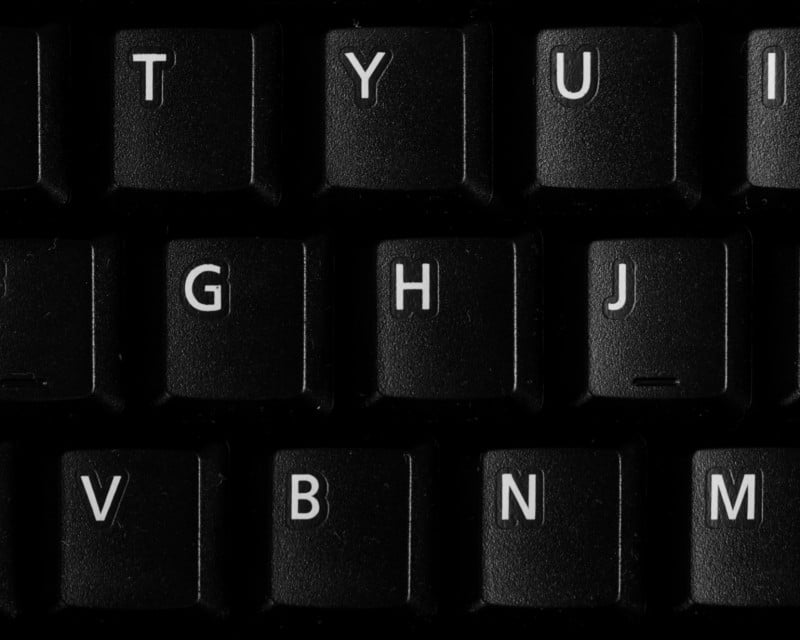
Last time we talked about the way that “text analytics” typically is not very analytical. Two exercises related to counting are the most commonly mentioned. Now let’s go on to the reasons for the bar being set so low.
This has happened in part because of the rapid evolution of computers and their abilities. Not that many years ago, simply get text into shape by doing activities such as stemming and stop word removal would have been the height of high technology. Doing only that much with text constituted a remarkable feat of computation.
As of about ten years ago, it was still easy to say that text analytics was in its infancy, as many writers did, and let it go at that. After all, counting is a high accomplishment for a toddler. But that was ten years ago, and with things moving very quickly, it is time that we expect more. Some writers have recognized this, For instance, Miner, Hill, Delen, Elder, Fast and Nisbet (along with some guest writers) collapsed themselves into a massive editorial “I” and gave the opinion that text analytics was perhaps in its “early adolescence.”
This does indeed happen to infants after about ten years, so they may be on to something. Although what this means is less clear. Does the method sulk and refuse to clean its room? Is it awkward around other methods it finds romantically interesting?
However, we need not worry too much about this, because text analytics already has made the next step. It already has moved from processing and enumerating to solving problems and forecasting outcomes so that actions can be changed. That is, we actually can move from the realm of summing up what is in text to the realm of forecasting outcomes—what is often called the predictive. (Not coincidentally, the successful use of three predictive methods is demonstrated in this author’s book Practical Text Analytics.)
Since the methods are there, we must conclude that adoption of true analytical methods has been slow because this involves challenges. First and foremost, we encounter the need to think and to frame the right questions. For instance, we should not stop with simply asking, “What are my customers saying?” We can instead ask, “What are my customers saying that we can use to get them to behave differently?”
Text commentary can indeed do this, in particular when linked with other data. The catch is in needing this connection to other data, whether it is purchasing patterns, online behavior, or responses to a survey. Without an outcome variable—such as percent of business renewed, share of wallet, a product or service rating, or willingness to recommend—text cannot be used for accurate prediction. That is, there must be a target behavior along with the text for predictions to become powerful and—to use a popular term—“actionable.”
You may otherwise see broad patterns—and indeed text comments alone may give early warnings about an impending disaster—but you must combine other knowledge with text to get beyond counting and describing, and so get to changing behaviors in ways you want. You need to measure a behavior to change it. This requires effort and forethought and cannot rely on data gathered for purposes other than analysis. This requirement may well be the main reason so much of text analytics has remained not quite analytic.
About the Author: Dr. Steven Struhl has been involved in marketing science, statistics and psychology for 30 years. Before founding Converge Analytic, he was Sr. Vice President at Total Research/ Harris Interactive for 15 years, and earlier served as director of market analytics and new product development at statistical software maker SPSS.