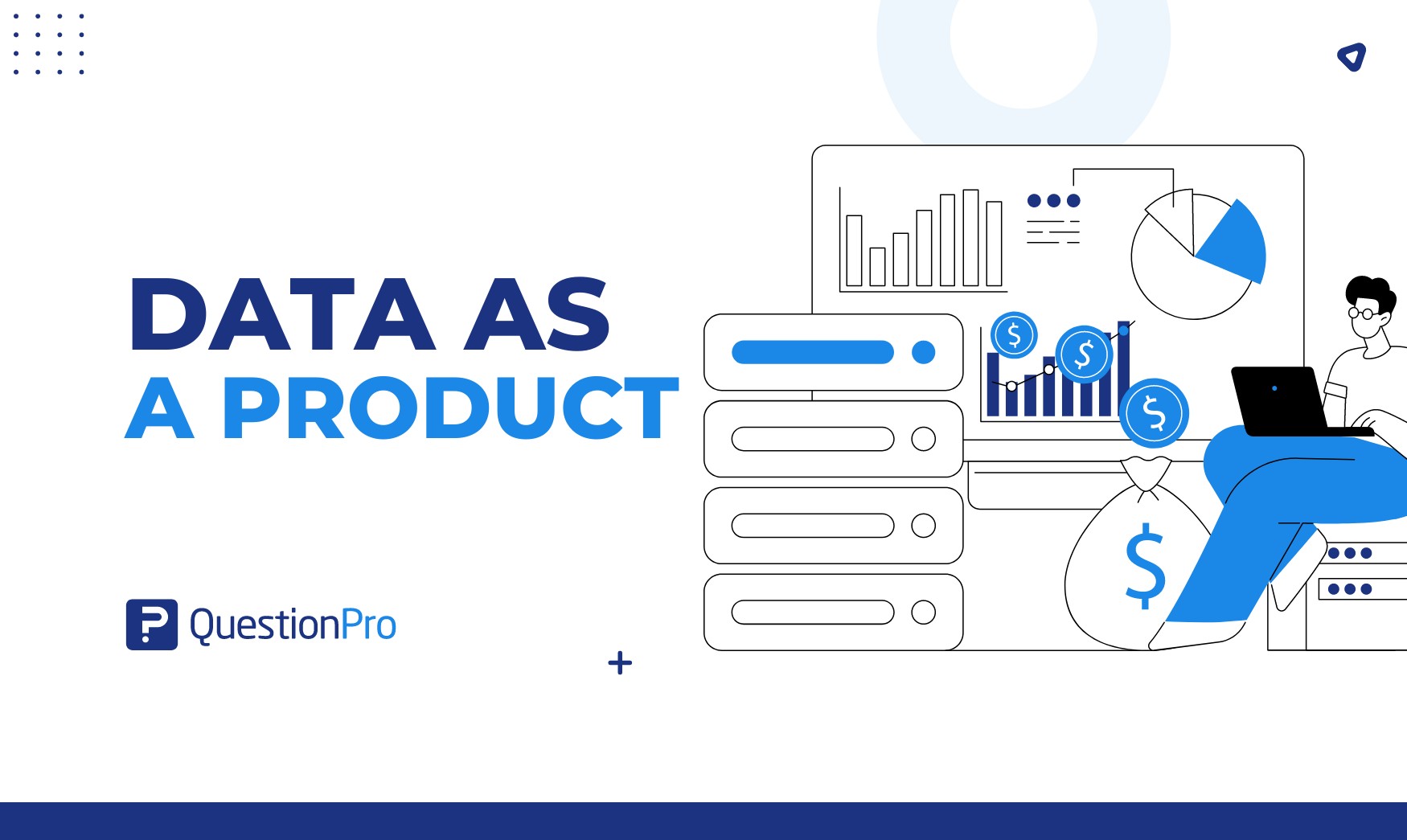
Businesses are constantly seeking innovative ways to extract value from their operations. One such transformative concept is “Data as a Product” (DaaP), which revolutionizes the traditional perspective on data.
Rather than treating data as a mere byproduct of business processes, organizations are now strategically collecting, processing, and packaging data to create marketable products. This shift signifies the recognition of data as a valuable asset that can enhance internal decision-making and generate revenue by offering useful insights to external entities.
In this blog, we dive into the key aspects of the Data as a Product concept and explore how teams can effectively apply this approach to unlock the full potential of their data warehouse.
Understanding Data as a Product?
Data as a Product refers to treating data as a valuable and marketable asset. Instead of viewing data solely as a byproduct of business operations, organizations can intentionally collect, process, and package data to create products sold or used to generate revenue.
For example, a company might gather and analyze customer behavior data to create insightful reports or predictive analytics tools. These data products can then be sold to businesses or individuals seeking valuable insights.
In essence, Data as a Product involves recognizing the intrinsic value of data and leveraging it as a tangible offering in the market. It’s a way for businesses to monetize their data assets and provide additional value beyond their core products or services.
The data engineers collaborated closely with the centralized data team to design a robust data architecture that ensured data integrity and quality, ultimately transforming data into a valuable product.
Key Aspects of the Data as a Product Concept
The “Data as a Product” (DaaP) concept involves treating data as a valuable asset managed, developed, and delivered with the same level of attention and care as any other product or service within an organization. Here are key aspects of the Data as a Product concept:
Here are some key aspects of the Data as a Product concept:
Value Proposition
Data is a valuable resource that can provide insights, support decision-making, and drive innovation. Companies recognize the potential to generate revenue by offering their data to external parties or creating new data-driven products and services.
Monetization
Organizations may monetize the company’s data by selling it directly to other businesses, researchers, or analysts. This could involve providing access to raw datasets or offering more refined and analyzed information.
Data Quality and Governance
Organizations must maintain high data quality and governance standards to ensure the data’s marketability. This involves ensuring accuracy, reliability, and compliance with privacy regulations to build trust among potential consumers.
Packaging and Delivery
Data as a product involves packaging data that is easily consumable by the target audience. This could include creating APIs, data feeds, reports, or dashboards that provide meaningful insights or raw data for further analysis.
Leveraging Technology
The advent of advanced technologies such as big data analytics, machine learning, and artificial intelligence has played a crucial role in enabling organizations to extract valuable insights from large datasets, making data more attractive as a product.
Internal Use
Data as a Product is not limited to external monetization. Organizations may also treat their data as a product for internal consumption, enabling different departments or teams to leverage it for decision-making and strategic planning.
Subscription Models
Some organizations adopt subscription models where internal customers pay regularly for access to updated datasets or ongoing data services.
The Data as a Product concept reflects a shift in mindset towards recognizing data as a strategic asset with the potential for internal and external value creation. It aligns with the broader trend of data-driven decision-making and the increasing importance of information in today’s digital economy.
How Teams Can Apply the Data as a Product Approach
Teams should follow a structured and collaborative process to apply the Data as a Product (DaaP) approach successfully. Here is a step-by-step guide on how data teams can use the Data as a Product approach:
1. Define Objectives and Goals
- Clarify Purpose: Clearly articulate the purpose and goals of the data product. Understand the specific business problems it aims to solve and ensure alignment with overall organizational objectives.
- Value Proposition: Define the value the data product should deliver, whether in terms of insights, efficiency improvements, or innovation.
2. Identify Stakeholders
- Inclusive Involvement: Involve a diverse set of stakeholders, including business leaders, data scientists, data analysts, and end-users. Gather insights from each perspective to understand diverse requirements and expectations.
- Cross-functional collaboration: Foster collaboration between different teams to comprehensively understand the data product’s potential impact.
3. Data Discovery and Exploration
- Thorough Exploration: Conduct a comprehensive exploration of available data sources. Understand the types of data and their quality, and identify how they can be leveraged to meet the defined objectives.
- Gap Identification: Identify gaps in the available data and formulate strategies for resolving or supplementing missing information.
4. Data Quality Assessment
- Evaluate Data Quality: Assess the accuracy, completeness, consistency, and reliability of the data. Implement thorough data quality assessments and establish data cleansing and validation processes to ensure high-quality outputs.
- Continuous Monitoring: Establish mechanisms for constant data quality monitoring, as maintaining high standards is ongoing.
5. Infrastructure Setup
- Robust Infrastructure: Establish a robust and scalable data infrastructure for storage, processing, and analysis. Consider leveraging cloud platforms for flexibility, scalability, and efficient resource and data management.
- Technology Integration: Ensure seamless integration of advanced technologies such as big data analytics, machine learning, and artificial intelligence, maximizing the potential for valuable insights.
6. Prototyping and Iteration
- Prototype Development: Build prototypes or minimum viable products (MVPs) to test and validate the data product’s feasibility and functionality.
- Iterative Refinement: Embrace an iterative approach, allowing continuous refinement based on feedback and evolving requirements.
7. Packaging and Delivery
- User-Friendly Formats: Package data in user-friendly formats such as APIs, data feeds, reports, or dashboards. Ensure that the delivery methods align with the preferences and needs of the target audience.
- Meaningful Insights: Strive to provide raw data and meaningful insights that can inform decision-making and drive value.
8. Training and Adoption
- User Training: Provide training sessions to data users who will interact with the data product. Ensure they effectively leverage the product management principles for their specific roles.
- Promote Adoption: Implement strategies to promote adopting the data product within the organization, emphasizing its value and potential impact.
9. Continuous Improvement
- Feedback Mechanisms: Establish feedback mechanisms to collect insights from business users and stakeholders. Use this feedback to drive continuous improvements to the data product.
- Adaptation to Changing Needs: Stay adaptive to evolving business needs and technological advancements, making necessary adjustments to enhance the data product’s relevance.
How QuestionPro InsightHub Can Define Data as a Product
QuestionPro InsightHub is a platform that provides tools for market research and community engagement. While it doesn’t inherently define data as a product, the platform allows organizations to gather, analyze, and derive insights from data collected through surveys, discussions, and other research methods.
To consider data as a product within the context of QuestionPro InsightHub, you can follow these general steps:
- Data Aggregation: QuestionPro InsightHub gathers data from various sources, consolidating information for a comprehensive view.
- Segmentation and Analysis: The platform allows for slicing and dicing data, enabling in-depth analysis to extract meaningful insights.
- Customization: Users can tailor the data presentation to meet specific needs, ensuring it aligns with the end goals.
- Visualization Tools: InsightHub provides robust visualization tools for clear and impactful representation of data trends and patterns.
- Accessibility: The platform ensures easy access to data, making it readily available for decision-makers and stakeholders.
- Monetization Opportunities: By packaging and presenting data effectively, organizations can explore monetization avenues, treating data as a valuable product.
- Continuous Updates: InsightHub facilitates real-time data updates, ensuring the product remains relevant and up-to-date.
- Security Measures: Robust security features protect the integrity and confidentiality of the data product, fostering trust among users.
Conclusion
The Data as a Product concept represents a paradigm shift in how organizations perceive and leverage their data. By recognizing data as a valuable asset with marketable potential, businesses can enhance internal decision-making and create additional revenue streams.
The systematic approach outlined for teams to apply the Data as a Product approach serves as a guide for organizations looking to unlock the full potential of their data.
As businesses continue to navigate the evolving landscape of the digital economy, embracing data as a strategic asset will undoubtedly be a cornerstone for innovation, growth, and sustained competitiveness. The future belongs to those who understand and capitalize on the power of data as a product.
QuestionPro InsightHub is a powerful tool for organizations looking to redefine data as a product. With its comprehensive suite of features for data collection, analysis, and visualization, the platform empowers users to transform raw data into actionable insights.
Organizations can package and deliver valuable information to stakeholders by leveraging these capabilities, turning data into a marketable product. This enhances decision-making processes and creates new possibilities for generating value from data assets.
QuestionPro InsightHub’s role in this process highlights its significance in helping organizations derive meaningful outcomes from their data initiatives.