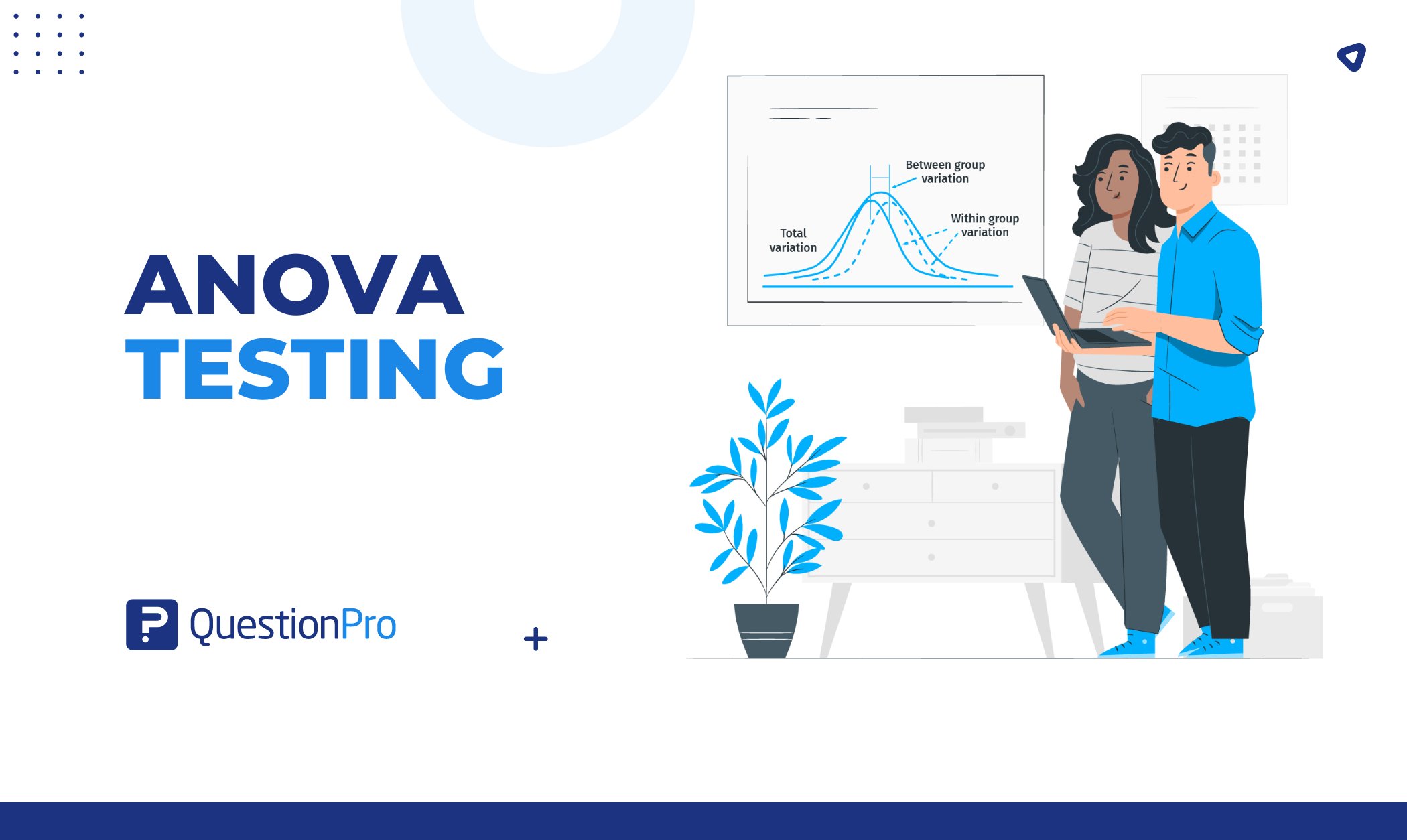
Surveys are effective at collecting data. However, insights develop after the fact and arise from the analysis we subject the data to. One of those techniques currently on my favored list is the tried and true analysis of variance (ANOVA) or ANOVA testing.
In the field of statistics, the Analysis of Variance (ANOVA) is a powerful and widely used technique for comparing means across multiple groups. ANOVA test provides researchers and data analysts with valuable insights into the variations between different groups and the effects of various factors.
Analysis of Variance (ANOVA) is a powerful statistical technique used to compare the means of two or more groups. It is widely employed in various fields, including psychology, biology, economics, and engineering, to name a few.
ANOVA helps researchers understand whether there are statistically significant differences among the group means and if those differences are due to random chance or actual effects.
This blog will explore what ANOVA testing is, its types and benefits, and provide some practical examples.
What is ANOVA?
Analysis of Variance (ANOVA) is a statistical method used to compare means between two or more groups to determine if they have statistically significant differences. It assesses whether the variations between the group means are greater than the variations within each group.
ANOVA is particularly useful when dealing with categorical data or when comparing the effects of different treatments or interventions on a continuous outcome variable.
The basic idea behind ANOVA is to decompose the total variance in the data into two components: variance between groups and variance within groups.
If the variance between groups is significantly larger than the variance within groups, it suggests that there are genuine differences between the groups being compared.
Types of ANOVA testing
There are different types of ANOVA based on the design of the study:
1. One-Way ANOVA
This term is used when one independent variable (factor) contains three or more levels or groups. For example, comparing the mean test scores of students from different schools (groups) based on a single factor like teaching method (e.g., traditional, online, hybrid).
2. Two-Way ANOVA
Involves two independent variables (factors) and is used when two main effects and interactions exist between them. For instance, comparing students’ performance based on teaching method and gender.
3. Factorial ANOVA
An extension of the two-way ANOVA that includes multiple factors with different levels, allowing for more complex designs and interactions.
4. Repeated Measures ANOVA
Employed when the same group of subjects is measured multiple times, such as before and after an intervention, to evaluate changes within the same subjects over time or conditions.
Benefits of ANOVA testing
ANOVA testing offers several significant benefits in statistical analysis and data-driven decision-making. Let’s explore some of the key advantages of using Analysis of Variance:
Comparison of Multiple Groups
One of the primary benefits of the ANOVA test is its ability to compare means across three or more groups simultaneously.
Instead of conducting multiple t-tests for each pair of groups, ANOVA allows researchers to analyze the variations between all groups in one comprehensive test. This saves time and reduces the chances of making type I errors (false positives) that can occur when conducting multiple tests.
Identifying Significant Differences
ANOVA helps identify whether there is a statistically significant difference between the means of the groups being compared.
By calculating the F-statistic and corresponding p-value, researchers can determine whether the observed differences between the group means are due to genuine effects or random chance. If the p-value is below a predetermined significance level (usually 0.05), researchers can confidently conclude that the groups have various differences.
Understanding the Impact of Factors
In experimental designs or observational studies with multiple independent variables, ANOVA enables researchers to understand the impact of each factor on the dependent variable.
By partitioning the variance into different components, researchers can quantify the contributions of individual factors and their interactions on the overall variability of the dependent variable. This helps in better understanding the underlying relationships and aids in making informed decisions based on the results.
Flexibility and Adaptability
ANOVA comes in various forms, such as one-way ANOVA, two-way ANOVA, and factorial ANOVA. This flexibility allows researchers to choose the appropriate model based on the complexity of their data and research question.
Additionally, ANOVA can be extended to handle different data types and distributional assumptions, making it applicable to a wide range of research fields.
Assumptions and Remedies
While the ANOVA test has numerous benefits, it is essential to be aware of its assumptions. The main assumptions include normality of the residuals, homoscedasticity (equal variance) of the residuals, and independence of observations.
However, if these assumptions are not met, certain remedies and alternative hypothesis approaches like non-parametric ANOVA or transformation of data can be applied to make the analysis robust.
Interpretation and Post-hoc Analysis
Following the ANOVA test, if the null hypothesis is rejected, researchers can employ post hoc tests to identify which specific group means differ significantly from one another.
Commonly used post-hoc tests include Tukey’s Honestly Significant Difference (HSD), Bonferroni, or Scheffe tests. These additional analyses provide deeper insights and help establish more accurate comparisons between individual groups.
Example of How to Use ANOVA
The researchers analyze the performance of students at various colleges. An R&D researcher can try two different product production techniques to assess whether a procedure saves money. ANOVA tests are a synthesis of several elements.
If the data is experimental, the system is applicable. Analytical variance is utilized when a person cannot access software that allows for manual calculating of variances. Simple to use and excellent for tiny sample amounts.
If we are collecting metric data with our surveys, perhaps in the form of responses to a Likert scale, the amount spent on a product, customer satisfaction scores, or the number of purchases made, then we open the door for analyzing differences in average scores between respondent groups.
Suppose we are comparing two groups at a time (e.g., men vs. women, new vs. existing customers, employees vs. managers, etc.). In that case, it is appropriate to use a t-test to assess the significance of any differences. However, if there are more than two groups, it becomes necessary to look to another technique.
ANOVA, or its non-parametric counterparts, allow you to determine if differences in mean values between three or more groups are by chance or if they are indeed significantly different. ANOVA is particularly useful when analyzing the multi-item scales common in market research.
In the table below, respondents in a restaurant survey rated three diners on overall satisfaction. The null hypothesis is there is no difference in satisfaction between the three restaurants. However, the data seems to imply otherwise.
Larry’s Diner 6.28
Curly’s Diner 6.05
Moe’s Diner 5.33
Overall 5.65
ANOVA makes use of the F-test to determine if the variance in response to the satisfaction questions is large enough to be considered statistically significant.
In this example, the F-test for satisfaction is 51.19, which is considered statistically significant, indicating a real difference between average satisfaction scores. ANOVA indicates whether or not there is a significant difference. It does not provide, however, direction as to which group is higher or lower.
Statistical test packages, such as SPSS and SAS, allow the survey researcher the option of selecting a post hoc test that compares groups for individual differences.
In regard to satisfaction, Larry’s Diner was the clear winner, with an average score significantly greater than either Curly’s or Moe’s. The difference between Curly’s and Moe’s was not large enough, given the number of respondents, to be significant.
The proper use of ANOVA in analyzing survey data requires that a few assumptions be met, including normal distribution of data, independence of cases, and equality of variance (each group’s variance is equal). If these assumptions cannot be met, non-parametric tests that do not require these assumptions are available.
Data by itself is just that. However, when we judiciously employ statistical tests, we can create insight that can positively impact our marketing efforts.
Conclusion
ANOVA testing is an indispensable statistical tool for researchers and data analysts seeking to compare multiple groups efficiently and effectively. Its ability to identify the significant difference, understand the impact of various factors, and provide flexibility in analyzing diverse datasets makes it a preferred choice in a wide range of fields, including medical research, social sciences, marketing, and manufacturing, among others.
However, it is crucial to recognize the assumptions associated with ANOVA and take appropriate measures to ensure the validity of the results. By harnessing the power of ANOVA, researchers can make well-informed decisions and contribute to advancing knowledge in their respective domains.