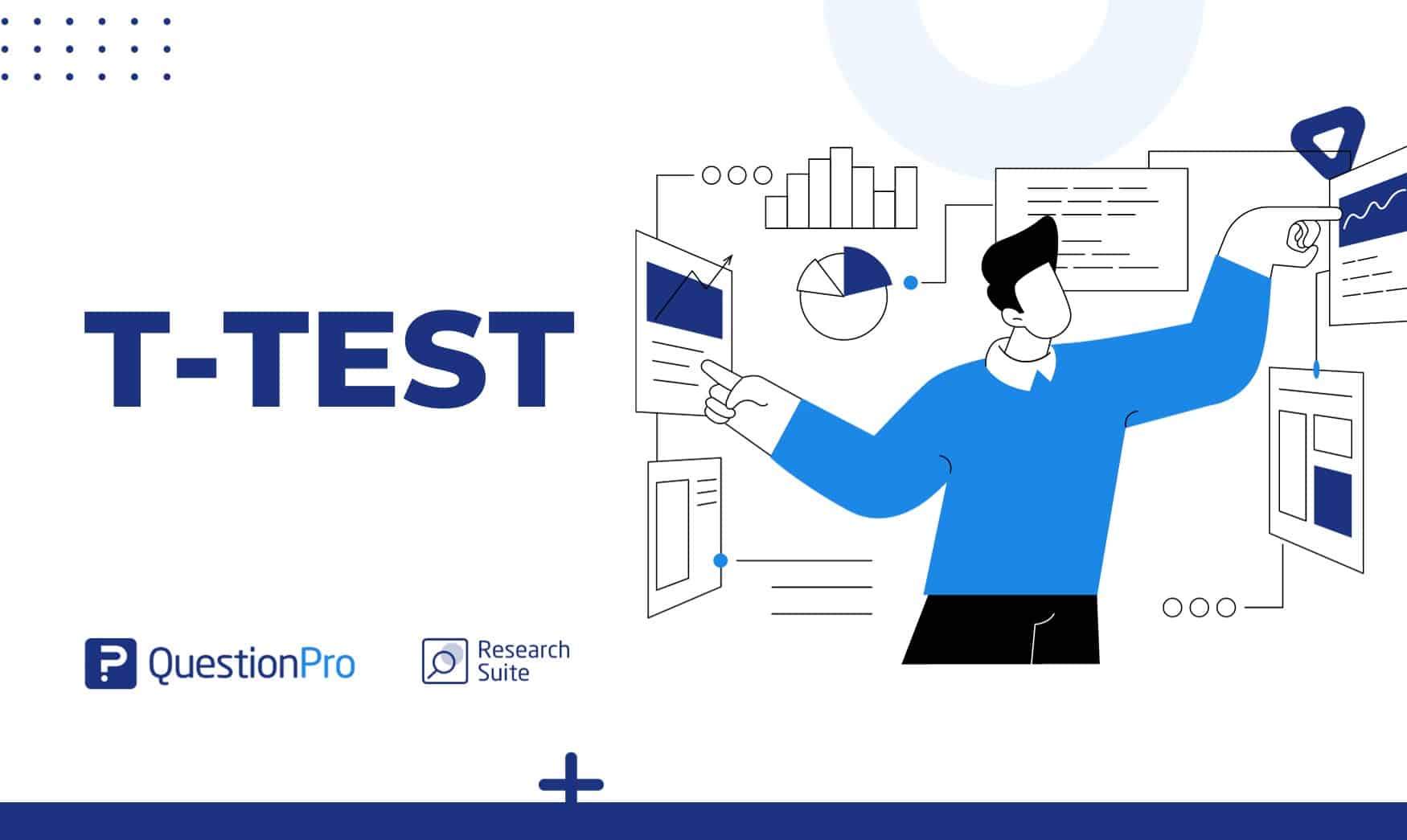
Using statistical analyses is crucial for making sense of research data, and the t-test is a key tool in this process. The test helps researchers find important differences between groups, whether they’re studying how different teaching methods affect student performance or evaluating the effectiveness of a new medical treatment.
This statistical test comes in two forms: independent and paired. It helps determine if differences in averages are likely because of real effects or just random chance. William Sealy Gosset, a British statistician, created it in 1908 while working at the Guinness Brewery. He needed a way to analyze small samples of data from beer production.
Nowadays, the t-test, also called Student’s t-test, is widely used in scientific and market research.
In this article, we will learn how the t-test works, its different applications, and how it is used in practice.
What is a T-test?
The t-test is a statistical test that helps you compare the mean of two sets of data to see if they’re noticeably different.
Imagine you have two groups of students: one group took math classes, and the other group didn’t. You can use the t-test to find out if the group that took math classes scored significantly higher on a math test than the group that didn’t.
When you use the t-test, you will get a “t value,” which indicates whether the difference between the averages of the two groups is important or not.
What are the main uses of T-test?
The test is used in many fields, such as medical research, psychology, economics, and education. Here are some of the main uses of the t-test:
- Comparing Two Groups: This test is used to compare data from two groups. For example, it helps assess if there’s a difference in test scores between two sets of students.
- Assessing Treatment Effectiveness: The t-test can be used to evaluate if a treatment has a significant impact on a variable compared to a control group that did not receive the treatment.
- Analyzing Experiments: In scientific experiments, the t-test is frequently used to compare results between a treatment group and a control group.
- Exploring Gender Differences: Gender studies often use the t-test to compare mean differences between men and women concerning a particular variable.
- Survey Data Analysis: You can also use it for survey data analysis to compare the means of two groups of data, such as comparing average income between different groups of men and women.
Types of T-test?
The Student t-test is an important statistical tool used in various forms, each designed to address specific research details. It’s essential for you to understand these types to ensure accuracy in your analysis. The most common types are:
01. Two-sample T-test for Independent Data
This test helps you compare the averages of two separate groups that aren’t connected. It’s handy when the observations in one group have no relation to the observations in the other group.
For instance, you can use it to compare the average grades of students from two different courses.
02. Two-sample T-test for Related or Paired Data
It is also known as a related samples t-test or paired t-test. In this type, the difference looks at the average values of connected groups in a detailed way.
For example, you can examine measurements taken before and after treatment within your own group of people.
03. One-sample T-test
This test helps you check if the average of one group is different from a known or expected value, like the overall average. It’s used to see if the group’s average is significantly different from what you expected.
04. Equal or Heterogeneous Variance T-test
Student t-tests usually expect the variances of the two groups being compared to be the same. But sometimes, this might not be the case.
The equal variances t-test is used when we assume the variances are equal, and the heterogeneous variances t-test is used when we assume they are different between the two groups.
05. One-tailed or Two-tailed t-test
A Student’s t-test can be either one-tailed or two-tailed, based on the research question.
If you want to know if one average is significantly higher or lower than another, use a one-tailed test. On the other hand, a two-tailed test is used to find any significant difference between the averages, whether higher or lower.
What is the One-sample Student’s t-test?
The one-sample Student’s t-test is a method used to find out if the average of a sample is different from a known or assumed average of the entire population. It’s particularly handy when the population doesn’t have a normal distribution or when the sample size is small (less than 30).
This test involves calculating the t-statistic. You get this by dividing the difference between the sample mean, and the assumed or known means by the sample standard deviation and then dividing that by the square root of the sample size.
Here’s the key: If the calculated t statistic is larger than the critical value of t, you find in a table specific to the Student’s t distribution (based on the chosen significance level and degrees of freedom, which is one less than the sample size), it means there’s enough evidence to say the sample mean is significantly different from the supposed or known mean.
In simpler terms, the one-sample Student’s t-test is a helpful tool for checking if a sample accurately represents a bigger population and for figuring out if the difference between the sample mean and the population mean is statistically significant.
Advantages of performing the T-test
The Student t-test is a handy statistical tool with several advantages for different research situations. Some of the main advantages are:
- Works with Different Sample Sizes: Unlike other tests, the t-test is flexible and can be used with both small and large samples.
- Doesn’t Require a Perfectly Normal Distribution: The t-test is robust, meaning it can handle situations where the data doesn’t perfectly follow a normal distribution, especially when the sample size is large.
- Easy to Calculate: This test is relatively simple and straightforward to calculate. This simplicity makes it practical and applicable in various research scenarios.
- Versatile Application: The test finds use in diverse fields like medical research, education studies, market research, and engineering, showcasing its wide-ranging applicability.
- Detects Statistical Significance: One of its main purposes is to determine whether the observed difference between the sample mean and the known or assumed population mean is statistically significant or not.
Steps to perform a Student t test
Performing a Student t-test is a careful and detailed process that requires close attention at every step. Let’s take a thorough look at the various aspects involved:
Step 1: Define the Null and Alternative Hypothesis
Start by creating a straightforward null hypothesis that says there’s no big difference between the averages. Then, make an alternative hypothesis that suggests there is a noticeable difference.
This first step is crucial because it sets up the hypotheses that will steer the whole analysis. It gives a clear direction for the investigation.
Step 2: Select the Appropriate Type of T-test
Decide whether to use an independent samples t-test or a paired samples t-test based on how the data sets are related.
The type of data you have will guide your decision. If you’re comparing data from separate groups, go for the independent samples t-test. If you’re working with related observations, choose the paired samples t-test.
Step 3: Calculate Mean, Standard Deviation, and Sample Size
Collect important information about each group, such as the average (mean), how spread out the values are (standard deviation), and the number of observations in each group (sample size).
These numbers will help you understand the typical value, the range of values, and how many data points are in each group. They are important for doing further calculations.
Step 4: Calculate the t-statistic
Use the right formula to calculate the t-statistic, taking into account the average differences, the spread of data, and the size of the samples.
This calculation helps measure how much the groups differ, combining information about the average and how spread out the data is for a detailed evaluation.
Step 5: Determine the Critical Value of t
Look at a Student t distribution table to find the important t value for the selected significance level, usually 0.05.
The critical t value helps decide whether to reject the null hypothesis in statistical analysis. It’s an important factor in making decisions based on statistics.
Step 6: Compare Calculated and Critical t Values
Check if the calculated t value is higher than the critical value from the distribution table.
This comparison is really important. If the calculated t value is greater than the critical threshold, it means you can reject the null hypothesis, showing there’s a significant difference between the means.
Step 7: Interpret Results and Conclude
Combine the results to make sense of them and understand the importance of the differences you observed.
In this last stage, turn the numbers and data into practical insights that have real-world meaning. This helps answer the research question and supports making well-informed decisions.
Conducting a t-test can be a bit tricky, especially when you need to think about whether your data is normal and if the variances are similar. If you find yourself dealing with these issues, it might be helpful to use statistical software or get help from a statistician.
Example of T-test
Here’s an example of using the Student t test in marketing research:
Let’s say a company wants to find out if there’s a big difference in customer satisfaction with two versions of its product. To do this, they randomly picked two groups, each with 50 customers, and asked them to rate their satisfaction on a scale of 1 to 10.
The first group tries version A, and the second group tries both version A and version B. The data they get looks like this:
Cluster | Half | Standard deviation |
TO | 7.5 | 1.5 |
b | 8.2 | 1.3 |
To check if there’s a notable difference between the two product versions, you can use a test called the Student’s t-test for independent samples. The results of the test show a t-value of -2.69 and a p-value of 0.009.
Comparing this p-value to a 5% significance level, you can conclude that there’s a significant difference in customer satisfaction between the two versions. Simply put, there’s statistical evidence supporting the idea that customers prefer version B over version A.
This information is valuable for the company in deciding how to produce and market the product. It suggests that version B is likely more appealing to customers and, therefore, could be more profitable in the long term.
What is the difference between the t-test and ANOVA?
The t-test and ANOVA (Analysis of Variance) are tools used to compare averages in different sets of data. However, there are some key differences between them:
- Number of groups:
- T-test: Used when comparing the average of two sets of data.
- ANOVA: Used when comparing the average of three or more sets of data.
- Type of variables:
- T-test: Works with continuous numerical variables and independent data.
- ANOVA: Works with continuous numerical variables and can handle both dependent and independent data.
- Type of result:
- T-test: Gives a t value, showing how significant the mean difference is between two groups.
- ANOVA: Provides an F value, indicating the significance of mean differences among three or more groups.
- Type of analysis:
- T-test: Conducts a univariate analysis, examining one independent variable at a time.
- ANOVA: Conducts a multivariate analysis, allowing the examination of several independent factors simultaneously.
Conclusion
In summary, the Student’s t-test is a valuable and flexible statistical technique that allows the mean of a sample to be compared with a hypothetical or known population mean, with a series of advantages that make it useful in various research contexts.
It is especially useful when working with small samples because it is based on the Student’s t distribution, which takes into account the additional uncertainty that occurs when working with small samples.
Remember that with QuestionPro, you can collect the necessary data for your investigation. It also has real-time reports to analyze the information obtained and make the right decisions.
Start by exploring our free version or request a demo of our platform to see all the advanced features.