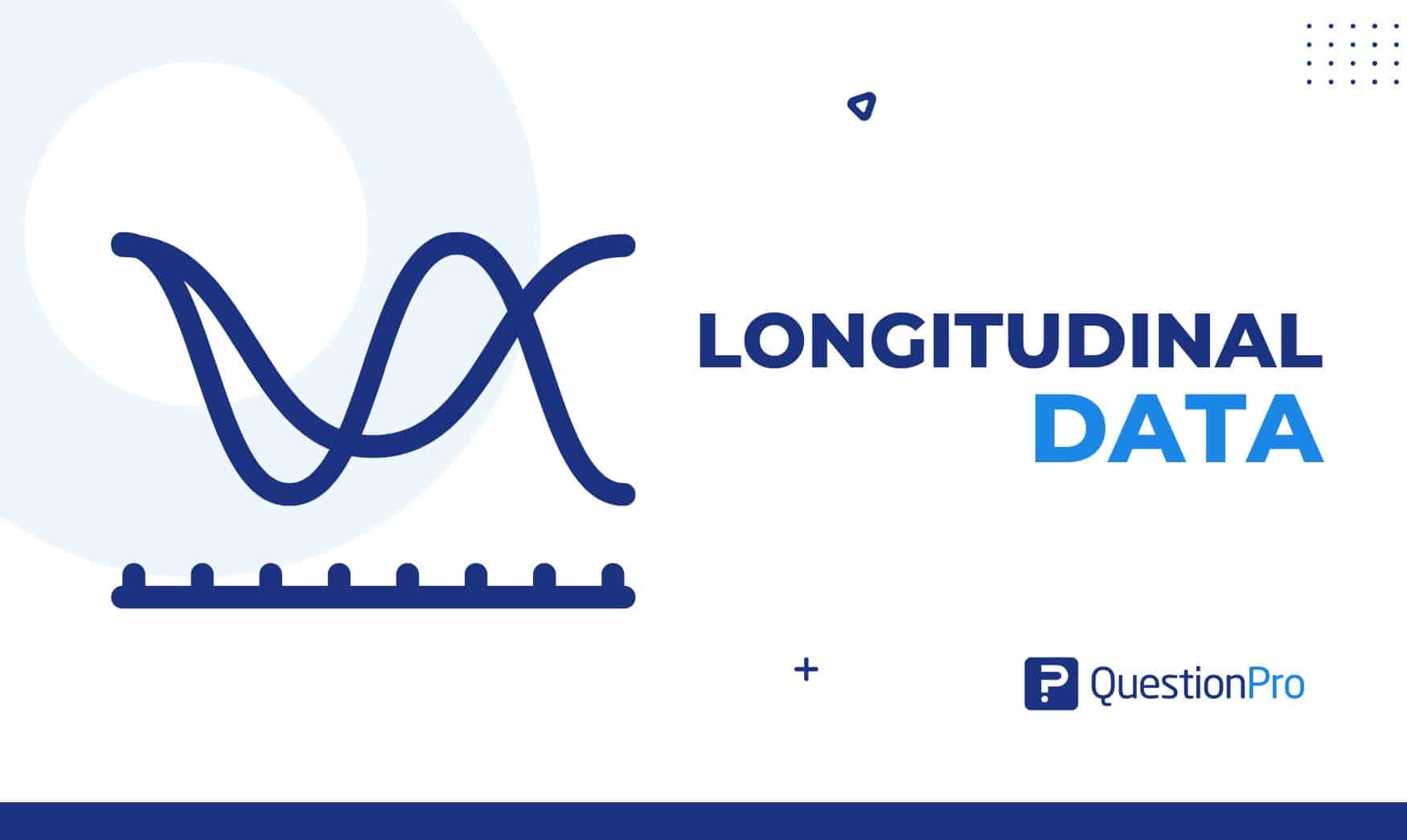
Longitudinal data is powerful and versatile information key to understanding changes and developments over time. This type of data collection involves observing and recording subjects or variables multiple times, allowing researchers and analysts to track trends, patterns, and variations.
Unlike cross-sectional data, which provides a snapshot in time, this data provides a dynamic perspective, revealing how variables evolve throughout the study.
In this blog, we’ll explore the definition of longitudinal data, its diverse uses, and the intriguing patterns and trends that emerge from its analysis.
What is Longitudinal Data?
Longitudinal data, or panel data, is a type of research design where the same subjects, entities, or observations are measured or observed at multiple points in time. This method allows researchers to examine changes within individual units and explore trends and patterns over an extended period.
Researchers strive to balance the need for complete data with the complexities of repeated cross-sectional data, recognizing that the accurate estimation of model parameters is crucial for understanding the dynamic relationships between standardized test scores and cardiovascular disease progression.
Scientists aim to derive meaningful insights from longitudinal studies through sophisticated analytical techniques despite the inherent challenges of missing data and incomplete data sets.
In longitudinal studies investigating the impact of cardiovascular disease, researchers often encounter challenges associated with missing data and incomplete longitudinal data. The presence of repeated observations within the same group necessitates careful handling of insufficient data to ensure accurate parameter estimates.
Analyzing standardized test scores over time in the context of cardiovascular health requires a comprehensive approach to address missing data, as the incomplete nature of this data can affect the reliability of model parameters.
Uses of Longitudinal Data
Longitudinal data refers to information collected from the same subjects or entities over an extended period. This data type is valuable for studying changes and trends over time, understanding development and growth, and exploring causal relationships.
Here are some common uses of this data:
1. Understanding Change Over Time
Longitudinal data is invaluable for tracking changes within a subject or population over time. This can be crucial in various fields, such as medicine, psychology, and sociology, where understanding the evolution of particular variables is essential.
2. Causality and Relationships
Longitudinal studies enable researchers to establish causal relationships by observing variable changes over time. This is particularly useful in fields like economics, where researchers seek to understand the impact of policy changes on economic indicators.
3. Forecasting and Prediction
The ability to observe trends over time allows for better forecasting and prediction. Businesses, for example, can use longitudinal data to anticipate market trends, plan for future demands, and adapt strategies accordingly.
4. Health Research
In medical research, a longitudinal study is crucial for understanding the progression of diseases, the effectiveness of treatments, and the long-term impact of various health interventions.
5. Educational Research
It is often employed in educational research to assess the impact of teaching methods, interventions, and academic policies on student outcomes over an extended period.
Analysis of Longitudinal Panel Data
Analyzing longitudinal panel data involves studying the same subjects or entities over multiple time points. This method provides a dynamic view of changes and trends. Key aspects of analysis include:
- Understanding Change: Track changes in variables to uncover patterns, behaviors, and trends within individual units.
- Causality and Relationships: Use longitudinal data to establish causal relationships by observing how changes in one variable impact others over an extended period.
- Forecasting and Prediction: Leverage this data for forecasting and prediction, aiding businesses in anticipating trends and planning for future demands.
- Health and Educational Research: Apply longitudinal analysis in medical and educational research to assess disease progression, treatment effectiveness, and the impact of interventions on outcomes.
- Identifying Patterns: Recognize patterns in longitudinal data, such as linear or non-linear trends, cyclical behaviors, and threshold effects.
- Advanced Techniques: Employ advanced analytical techniques like machine learning to extract deeper insights from these datasets.
- Real-Time Analysis: Respond to the growing demand for real-time insights by analyzing this data as it unfolds, enabling timely decision-making.
Patterns in Longitudinal Data
Analyzing patterns in longitudinal data involves identifying trends, variations, and correlations over time. Here are several common patterns that researchers often explore when working with this data:
1. Linear Trends
Linear trends depict a consistent and uniform change in a variable over time. When plotted on a graph, this pattern is characterized by a straight line and indicates a steady increase or decrease in the variable.
2. Non-linear Trends
Non-linear trends showcase a more complex relationship between variables over time. This may involve exponential growth or decline, oscillations, or irregular fluctuations.
3. Cyclical Patterns
Some of this data exhibit cyclical patterns, repeating specific trends or behaviors in a regular sequence. This is common in economic data, where business cycles demonstrate growth, recession, and recovery periods.
4. Threshold Effects
Longitudinal data can reveal threshold effects, where a variable experiences a sudden and significant change after crossing a certain threshold or trigger point.
Emerging Trends in Longitudinal Data Analysis
Emerging trends in longitudinal data analysis include adopting advanced statistical techniques and methods, integrating with other data types, and developing more sophisticated software tools.
Here are some trends that were relevant at that time, and it’s possible that there have been further developments since then:
Advanced Analytical Techniques
With the rise of big data and technological advancements, researchers now employ sophisticated analytical techniques such as machine learning and artificial intelligence to extract deeper insights from these datasets.
Integration of Multiple Data Sources
Combining longitudinal data with other sources, such as geospatial data or social media analytics, allows for a more comprehensive understanding of the factors influencing trends.
Focus on Real-Time Analysis
The demand for real-time insights has increased the emphasis on analyzing longitudinal data as it unfolds. This approach enables organizations to make timely decisions based on the most up-to-date information.
Advantages and disadvantages of longitudinal Data
Longitudinal data refers to information collected from the same subjects or participants over an extended period of time. This type of data is valuable for studying changes and trends over time. Here are some advantages and disadvantages of it.
Advantages:
- Temporal Analysis: Provides insights into changes over time, allowing researchers to observe trends, patterns, and developments.
- Causality: Enables the investigation of cause-and-effect relationships by tracking variables over an extended period.
- Individual Variation: Captures individual-level changes, helping understand intra-individual variability and factors influencing it.
- Holistic Understanding: Offers a more comprehensive view of complex phenomena by considering the dynamic nature of variables.
Disadvantages:
- Resource Intensive: Requires significant time, effort, and financial resources to collect and maintain data over an extended period.
- Attrition Bias: Participants may drop out or be lost to follow-up, leading to biased results and reduced sample sizes.
- Time-Dependent Confounding: Changes in external factors over time may confound results, making it challenging to isolate causal relationships.
- Logistical Challenges: Longitudinal studies may face logistical issues such as managing data integrity, participant compliance, and tracking changes consistently.
Conclusion
Longitudinal data is a treasure trove of insights, providing a dynamic perspective on the evolution of variables or subjects over time. Its applications span various disciplines, from healthcare to economics, enabling researchers and analysts to make informed decisions and predictions.
As technology continues to evolve, the ability to harness the power of longitudinal data will undoubtedly lead to more profound insights and transformative discoveries in various domains.
QuestionPro Research is an invaluable tool for this data studies, providing a robust platform for seamless data collection and analysis over extended periods. Its user-friendly interface, advanced survey features, and powerful analytics empower researchers to track changes, trends, and correlations over time.
With QuestionPro, longitudinal studies become efficient and insightful, yielding meaningful results for informed decision-making and comprehensive research.