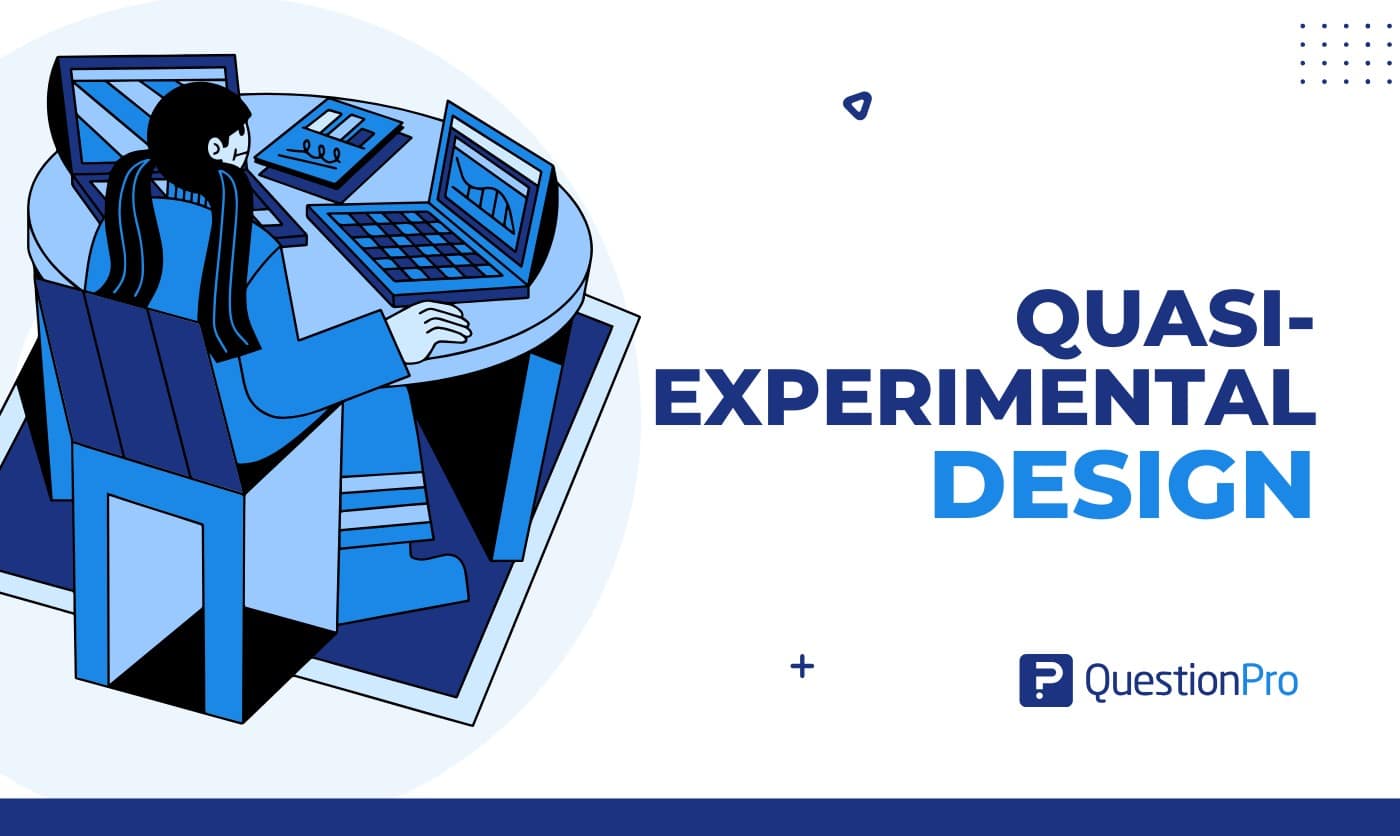
When it comes to research, experiments are often the gold standard for finding cause-and-effect relationships. But what if you can’t randomly assign people to different groups or conditions? A quasi-experimental design makes all the difference in helping you to handle this type of situation.
While quasi-experimental designs don’t offer the same level of control as true experimental designs, they are still incredibly useful for studying real-world situations where randomization is difficult or impossible.
In this article, we’ll explore what quasi-experimental designs are and their different types and provide examples to help you understand how they work.
What is Quasi-Experimental Design?
A quasi-experimental design is a research method used to understand cause-and-effect relationships when researchers can’t randomly assign people to different groups. Instead of randomizing, the study compares groups that already exist or assigns people to groups based on other factors.
For example, researchers might study the effects of a new teaching method by comparing two schools, one using the method and one not without randomly assigning students to the schools. While quasi-experiments can show us how something might affect an outcome, they are less certain than true experiments because the groups might differ in ways that affect the results.
This type of research is a great alternative in situations where true experimental designs are challenging to implement due to various logistical or ethical reasons.
Importance of Quasi-Experimental Design
The use of Quasi-Experimental Design has many benefits for researchers as it offers an alternative to other types of research that have limitations that can be overcome with this methodology. However, its importance goes beyond that, below we list some of the most important ones:
1. Real-World Application
Quasi-experiments are often used in real-life situations like educational research, hospitals, or communities because they help researchers study things as they naturally happen.
For example, if a school starts using a new teaching method, researchers can compare students’ performance before and after the change without randomly assigning some students to different groups. This makes the findings more realistic and easier to apply to everyday life
2. Ethical and Practical Limitations
Sometimes, random assignments aren’t possible or ethical. It wouldn’t be ethical to randomly assign people to live in different environmental conditions to study their health outcomes. Quasi-experimental designs allow researchers to explore these types of questions without violating ethical standards.
3. Flexibility in Research
Quasi-experiments give researchers the flexibility to study a wide range of topics. They can study the effects of new policies, treatments, or interventions in natural settings. It gives us insights into how things work in the real world.
4. Cost-Effective
Running a full-scale randomized experiment can be both expensive and time-consuming. Quasi-experimental designs, on the other hand, are often more budget-friendly because they can use existing groups or data. This means researchers don’t have to set up expensive experiments from scratch, making it a more practical option when time or money is limited.
5. Helps Identify Trends and Effects
While quasi-experiments provide valuable insights. Researchers can spot patterns and trends that help them understand how different factors might be influencing outcomes, even if they can’t prove a cause-and-effect relationship for sure.
These studies still give us a good sense of what’s happening in real-world situations. For example, comparing regions that have different policies can help show if those policies are working, even without a perfect experiment.
Key Concepts in Quasi-Experimental Design
To better understand how these designs work, it’s helpful to grasp some key concepts that are central to their structure and application. Here are the main concepts you need to know:
- Non-Random Assignment: Participants in quasi-experiments are not randomly assigned to groups. Instead, groups are pre-existing or assigned based on other criteria.
- Comparison Groups: Quasi-experiments compare a treatment group (exposed to an intervention) with a control group (not exposed) to assess differences in outcomes.
- Causal Inference: The goal is to understand cause-and-effect relationships, even without random assignment. Researchers infer causality based on group comparisons and observed changes.
- Confounding Variables: These are variables other than the treatment that might influence the results. Quasi-experiments must account for these to avoid biased conclusions.
- Pre- and Post-Testing: Many quasi-experiments measure outcomes before and after an intervention, helping to identify changes that might be caused by the treatment.
- Threats to Internal Validity: Since there’s no random assignment, there’s a higher risk of biases, like selection bias or history effects, which can affect the reliability of findings.
- Natural Experiments: These are situations where a naturally occurring event or policy change provides an opportunity to study causal effects without direct manipulation by researchers.
- Regression Discontinuity: This design assigns people to different groups based on a cutoff score (e.g., test scores), allowing researchers to compare outcomes just above and below the threshold.
Types of Quasi-Experimental Design With Examples
There are different types of quasi-experimental designs because researchers need flexibility depending on the situation, the kind of data they can collect, and the questions they’re trying to answer. Each type has its strengths and weaknesses, and they are usually classified based on how the groups are formed and how data is collected over time.
Here’s an explanation of some common types of quasi-experimental designs:
1. One-Group Posttest-Only Design
In this design, researchers test a single group of participants after an intervention or treatment has occurred. There is no pretest or comparison group involved. Without a comparison group or pretest, it’s difficult to know if any observed changes were due to the treatment and control groups or some other factor.
- Example: A school introduces a new reading program for its students and wants to see if it improves their reading skills. After the program ends, the school tests students’ reading levels to measure improvement. Since there is no pre-test or control group, the results can only show how students performed after the program, but it’s unclear whether the program itself caused the improvement.
- Challenge: Without a pre-test or comparison group, it’s hard to tell if any changes are truly due to the new reading program or if they are the result of other factors (like more time spent reading or natural development).
2. One-Group Pretest-Posttest Design
This design involves measuring participants before and after an intervention. Researchers compare the scores or outcomes from the same group at two different times—before and after the treatment.
- Example: A teacher wants to test whether a new teaching method improves students’ math scores. The teacher gives a math test to the students before using the new method (pretest) and then gives the same test to the same group after using the method for a month (posttest). By comparing the pretest and posttest scores, the teacher can evaluate any improvements.
- Challenge: Since there is no control group with which to compare the results, it’s hard to know if the improvement is because of the new teaching method or if other factors (like additional studying or changes in student motivation) played a role.
3. Non-Equivalent Groups Design
In this design, researchers compare two or more groups that are not randomly assigned but are instead pre-existing. One group receives the treatment, and the other group does not. Since the groups are not randomly assigned, the differences between them may be due to pre-existing characteristics, not the treatment.
- Example: A researcher wants to study the effects of a new wellness program on employee productivity. The program is introduced in one company, but another company doesn’t implement the program. The researcher compares productivity in both companies at the end of the program.
- Challenge: Since the two companies weren’t randomly assigned to the program, differences between the companies (such as workplace culture or resources) may influence the results, making it difficult to attribute any changes solely to the wellness program.
4. Time Series Design
In this design, researchers observe the same group over a period of time, measuring outcomes at multiple points before and after an intervention or event.
Time series data can be affected by other changes happening during the same period, so it’s hard to say for sure that the observed effects were due to the intervention.
- Example: A government implements a new law banning smoking in public places. A researcher tracks the number of smoking-related hospital admissions in the city over several years before and after the law to see if there’s a noticeable decline.
- Challenge: Other factors, like new healthcare initiatives, changes in public health campaigns, or even seasonal trends, might affect hospital admissions, making it difficult to attribute any changes solely to the smoking ban.
5. Regression Discontinuity Design
This design assigns participants to different groups based on a cutoff score or threshold. Those above the cutoff get the treatment, and those below do not. Researchers then compare the outcomes of those just above and just below the cutoff.
- Example: A scholarship is offered to students who score above a certain grade on an entrance exam. A researcher compares the academic performance of students who scored just above the cutoff (who received the scholarship) with students who scored just below it (who did not receive the scholarship).
- Challenge: The results may only apply to students near the cutoff, and it’s unclear if students who scored much higher or lower than the cutoff would show the same results.
6. Propensity Score Matching
This design tries to match participants from different groups who have similar characteristics, except for the treatment they received. It aims to create “matched pairs” of individuals who are alike in every way but one, reducing bias in comparisons. While this method reduces bias, it’s not perfect. There could still be unmeasured differences between the groups that affect the results.
- Example: A researcher studies the impact of a new job training program on employment rates. Instead of randomly assigning people to the program, the researcher matches people who joined the program with similar people who didn’t, based on factors like age, experience, and education level.
- Challenge: While this technique controls for some biases, it can’t account for every factor that might influence whether someone participates in the program, such as personal motivation or external circumstances.
How to Conduct a Quasi-Experimental Study?
While it’s not as strict as a true experiment, a well-designed quasi-experiment can still offer valuable insights. Here’s a step-by-step guide on how to conduct a quasi-experimental study in simple terms:
1. Define Your Research Question
The first step is to clearly define what you want to study. What is the intervention or treatment you’re interested in? What outcomes do you expect to measure? Your research question will help guide the design of your study and determine what kind of quasi-experimental method you’ll use.
2. Choose Your Design Type
Once you have your question, you need to decide which type of quasi-experimental design will work best. Here are a few options:
- One-Group Pretest-Posttest Design: Measure the outcome before and after the intervention in the same group of people.
- Non-Equivalent Groups Design: Compare two groups that are not randomly assigned, like a treatment group and a control group.
- Time Series Design: Observe the same group over time, both before and after the intervention.
- Regression Discontinuity Design: Use a cutoff to assign participants to a treatment based on a score or measurement.
- Propensity Score Matching: Match participants from different groups based on similar characteristics to compare them.
Your choice depends on your research context and the resources available.
3. Select Your Participants
Since quasi-experimental designs don’t involve random assignment, you’ll need to choose your participants carefully. In many cases, groups are pre-existing, such as different classrooms, schools, or neighborhoods.
- Treatment Group: The group that will receive the intervention or treatment (e.g., the people who will follow the exercise program).
- Comparison Group: The group that does not receive the treatment (e.g., people who do not join the exercise program).
Make sure both groups are as similar as possible, or control for differences that might affect the results.
4. Collect Data Before and After the Intervention
For most quasi-experiments, you’ll want to measure the outcome both before and after the intervention. This helps you compare how the outcome changes over time.
The data collection will depend on your research question. In some designs, you might only measure the outcome after the intervention, while in others, you’ll gather more detailed, repeated data over time.
5. Analyze Your Data
Once you’ve collected your data, the next step is to analyze it and see if there’s any significant difference between the groups or the pre-and post-intervention measurements.
6. Interpret the Results
you’ll need to interpret your findings carefully after analyzing the data. Since quasi-experiments don’t have the same level of randomized controlled trials, it’s important to be cautious about drawing strong conclusions.
7. Report Your Findings
Finally, share your findings with others! Write a clear report or paper that explains your research question, methodology, results, and conclusions.
Be transparent about any limitations of your study. Since quasi-experiments have limitations (like the inability to randomize participants), it’s important to acknowledge these and suggest how future research could overcome them.
Quasi-Experimental vs. Experimental Design
When it comes to research, one of the most important decisions researchers have to make is choosing the right design. The two most common types of designs are experimental and quasi-experimental, and while they might seem similar, they have key differences.
Understanding these differences can help you know when to use each design and what kind of conclusions you can draw from them. Let’s break it down in a simple way!
Experimental Design
In an experimental design, researchers create a controlled environment to test a hypothesis. The key feature is random assignment—participants are randomly assigned to either a treatment group (the group that gets the intervention) or a control group (the group that does not receive the treatment).
This randomization helps ensure that the groups are similar at the start of the experiment so any differences in outcomes can be more confidently attributed to the intervention.
Quasi-Experimental Design
In a quasi-experimental design, researchers also study the effects of an intervention, but they don’t randomly assign participants to groups. Instead, they rely on pre-existing groups or use other non-random methods to assign people to different conditions. This design is often used when random assignment is not possible or ethical.
Key Differences Between Experimental and Quasi-Experimental Designs
Here’s a breakdown of the key differences between experimental designs and quasi-experimental designs:
1. Random Assignment
- Experimental Design: Random assignment will be used to divide participants into different groups. This helps eliminate biases and ensures that the groups are as similar as possible at the start of the study.
- Quasi-Experimental Design: No random assignment. Participants are placed in groups based on existing factors (like schools, neighborhoods, or other natural groupings).
2. Control Over Variables
- Experimental Design: Researchers have more control over variables because random assignment helps balance out factors like age, gender, or background between the groups.
- Quasi-Experimental Design: Less control over the variables. Since the groups aren’t randomly assigned, other factors could be influencing the outcome, making it harder to attribute changes to the intervention itself.
3. Causality
- Experimental Design: Because of random assignment, researchers can more confidently claim cause-and-effect relationships. They can say that the treatment caused the observed changes.
- Quasi-Experimental Design: While you can identify associations, it’s harder to prove causality. Since the groups aren’t random, there’s a greater risk that other factors are influencing the results.
4. Ethical or Practical Concerns
- Experimental Design: Random assignment might not always be ethical or practical. For example, you can’t randomly assign people to harmful conditions just to test the effects.
- Quasi-Experimental Design: When random assignment is not feasible for ethical or practical reasons, quasi-experiments are a good alternative.
How to Conduct a Quasi-Experimental Study With QuestionPro?
QuestionPro is a great tool for conducting quasi-experimental studies because it helps you easily collect and analyze data. Here’s how you can use QuestionPro to run your own quasi-experimental study.
Step 1: Clarify Your Research Question and Design
Before you begin, think about the question you want to answer and decide on the type of quasi-experimental design you’ll use. A few examples of common designs are:
- One-Group Pretest-Posttest: You measure a group before and after the intervention to see if there are any changes.
- Non-Equivalent Groups: You compare two groups that aren’t randomly assigned—one gets the intervention, the other doesn’t.
- Time Series: You look at data over time, before and after an event or intervention.
For example, let’s say you’re investigating whether a new online training program improves employees’ productivity. You might want to use the One-Group Pretest-Posttest design, where you measure productivity before the training and then again after the training.
Step 2: Choose Your Participants
In a quasi-experimental study, participants aren’t randomly assigned. Instead, you either work with existing groups or measure changes in a single group over time.
Step 3: Create Your Survey in QuestionPro
Now that you’ve chosen your participants, it’s time to create your surveys. These surveys will help you collect the data you need for your quasi-experiment.
- Pretest Survey: This is the survey you sent out before the intervention. For example, if you are testing a new wellness program, your pretest survey might ask about participants’ current health habits or productivity levels.
- Posttest Survey: After the intervention (e.g., after the training program), send a follow-up survey to measure any changes. Make sure the questions in the posttest survey are similar to those in the pretest survey so you can compare the results.
- Survey Questions: Use a mix of multiple-choice, Likert scale (e.g., rate your agreement from 1 to 5), and open-ended questions to gather different types of data.
Step 4: Administer the Survey and Collect Data
Once your surveys are ready, it’s time to distribute them and start collecting data.
- Pretest: Send out your pretest survey to your participants before the intervention starts. For example, if you’re testing a wellness program, measure employees’ health and productivity levels before the program begins.
- Posttest: After the intervention, send out your posttest survey to measure the changes. For instance, after the wellness program ends, ask the same questions to see if there were improvements.
You can distribute the surveys via email, share a link, or use QR codes for easy access on mobile devices. QuestionPro makes it simple to track responses so you’ll know how many people have completed the survey.
Step 5: Ensure Good Data Quality
Good quality data is key to getting meaningful results. QuestionPro has tools to help you make sure your data is accurate:
- Skip Logic: This ensures that participants only see the questions that are relevant to them. For example, if someone didn’t take part in the program, they don’t need to answer follow-up questions about it.
- Validation Rules: Use these to ensure participants enter correct answers (like limiting responses to a certain number range).
- Response Monitoring: You can keep track of responses in real-time. It will allow you to see how many people have answered.
Step 6: Analyze Your Data
the next step is analyzing your data. QuestionPro makes this easy with tools to help you dig into the results and spot any significant changes. Also, you can check out things like:
- Average scores
- Percentages and
- Trends across different questions all right within the platform.
Step 7: Interpret Your Results
Once you’ve completed your analysis, take time to interpret the findings. Here are a few things to consider:
- For a pretest-posttest design, did you see a significant change in your participants’ behavior or performance after the intervention? Were there any surprises or unexpected results?
- If you used a Non-Equivalent Groups design, compare the two groups to see if the intervention had a positive effect on one group but not the other.
- Remember that quasi-experimental designs are more susceptible to bias because participants aren’t randomly assigned. Consider any outside factors that may have influenced the results, like external events or individual differences.
Step 8: Report Your Findings
After interpreting your data, you’ll need to report your findings. QuestionPro makes it easy to generate reports with visuals, like charts and graphs, that help others understand your results clearly. Share your findings with stakeholders or use them to refine future interventions.
If the intervention led to improvements, you can recommend expanding it or trying similar interventions in other settings. If the intervention didn’t work as expected, look at the data to understand why. Did the program fail to engage participants? Were there external factors affecting the results?
Conclusion
Though a quasi-experimental design comes with its challenges, they’re a great way to explore the impact of programs, treatments, or changes in everyday settings. They allow us to make informed decisions and improvements, all while working within the limits of what’s actually possible in the real world.
Running a quasi-experimental study with QuestionPro can be an effective way to understand the impact of real-world interventions. QuestionPro simplifies the entire process, from creating surveys to analyzing results, making it easier to conduct and manage your study.
Whether you’re testing a new program, policy, or practice, QuestionPro helps you collect the data you need to make informed decisions. Contact QuestionPro for further information!