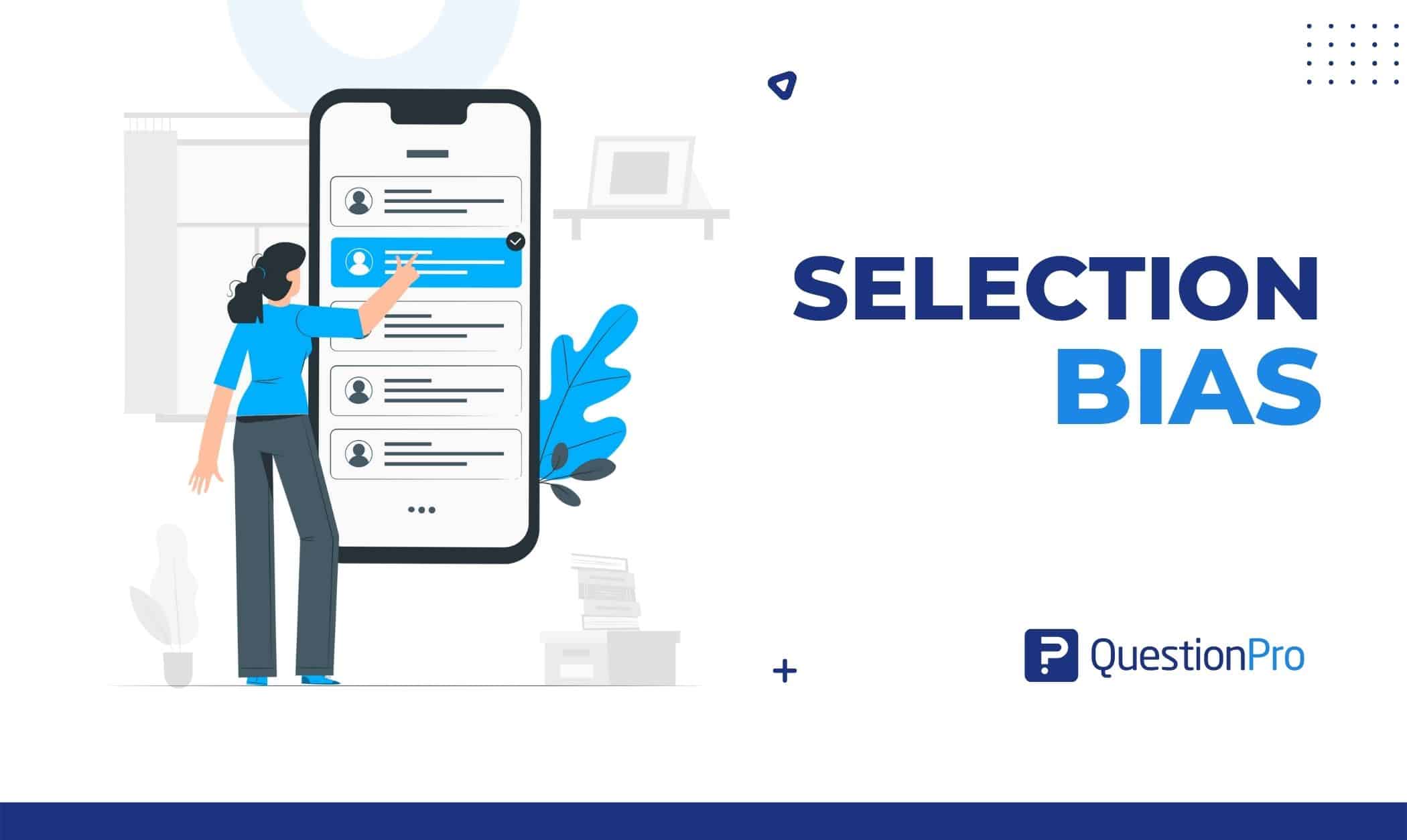
Researchers may need help with findings that don’t match the realities of the target community. There are numerous causes, but one of the most important is selection bias. It occurs when the study sample needs to accurately represent the population of interest, resulting in variations in the research results.
Understanding selection bias, its practical impacts, and the best ways to avoid it will help you deal with its effects. Everything you need to know about how to enhance your data collection process will be covered in this post.
What is selection bias?
Selection bias refers to experimental mistakes that lead to an inaccurate representation of your research sample. It arises when the participant pool or data does not represent the target group.
A significant cause of selection bias is when the researcher fails to consider subgroup characteristics. It causes fundamental disparities between the sample data variables and the actual research population.
Selection bias arises in research for several reasons. If the researcher chooses the sample population using the incorrect criteria, they may find numerous examples of this bias. It may also happen due to elements that affect study volunteers’ willingness to continue participating.
What are the types of selection bias in research?
Several types of selection bias can happen at various points in the research process. The following are some of them:
- Sampling bias
Sampling bias is a form of selection bias brought on by non-random population sampling. It occurs when specific subsets are removed from the research sample, leading to an inaccurate representation of the subgroups in the sample population.
For example, imagine you are researching the prevalence of heart disease in your area. To collect data, you decide to conduct interviews with shoppers at the shopping mall.
This strategy excludes hospitalized and heart disease patients. Your sample is biased because many people are not present in that shopping mall but staying at their homes or hospitals.
- Self-selection bias
Self-selection bias is also known as volunteer bias. This arises when the qualities of the people who voluntarily participate in the study are important to the goals of the investigation.
Self-selection causes biased data if the sample group consists of volunteers rather than the ideal target population. It is quite likely that researchers will likely get biased results.
For example, a pro-auto fan might participate in a study that examines the perception of a new car entering the target market since they see themselves as experts in the field.
Due to the self-selection bias, they could respond inappropriately or provide more information that wasn’t asked for.
- Nonresponse bias
Nonresponse bias happens when people don’t answer a survey or participate in a research project. It often happens in survey research when participants lack the appropriate abilities, lack time, or feel guilt or shame about the topic.
For Example, Researchers are interested in how computer scientists view a new piece of software. They conducted a survey and found many computer scientists didn’t respond or finish.
Researchers found that the respondents believe the software is excellent and high-quality after receiving the data. However, they discover that they receive mainly unfavorable criticism after releasing the new software to the full population of computer scientists.
The survey participants were entry-level computer scientists who couldn’t spot program flaws. The survey respondents did not reflect the more significant computer scientist population. Hence the results were inaccurate.
- Survivorship bias
Survivorship bias occurs when a researcher subjects variables to a screening contest and selects those who successfully complete the procedure. This preliminary selection method eliminates failed variables because of their lack of visibility.
Survivorship bias focuses on the most successful factors, even if they don’t have relevant data. It can alter your research outcomes and lead to unnecessarily positive views that don’t reflect reality.
Suppose you’re researching entrepreneur success variables. Most famous entrepreneurs didn’t finish college. It could make you assume that leaving college with a strong concept is enough to launch a career. But the majority of college dropouts don’t end up rich.
Actuality, many more people dropped out of college to launch unsuccessful businesses. In this example, survivorship bias occurs when you only pay attention to dropouts who succeeded and ignore the vast majority of dropouts who failed.
- Attrition bias
Attrition bias occurs when some survey respondents drop out while it is still being conducted. As a result, there are many unknowns in your research findings, which lowers the quality of the conclusions.
Most of the time, the researcher looks for trends among the drop-out variables. If you can identify these tendencies, you might be able to determine why the respondents left your survey suddenly and take appropriate action.
- Recall bias
Recall bias happens when some sample members struggle to recall crucial information, which impacts your research process. It takes place when researchers reject what is in front of them and instead see what they want to see.
You’ll get a biased result if you just survey those who’ve seen a new movie. Those who have seen it will say they loved it, while those who haven’t will say they didn’t. This is because people who like the movie are more willing to discuss it than those who don’t.
- Undercoverage bias
Undercoverage bias arises when a representative sample is drawn from a smaller proportion of the target population. Online surveys are especially vulnerable to undercoverage bias.
In an online survey on self-reported health, let’s say you are focusing on excessive drinking and smoking behaviors. Although, because of your way of conducting the survey, you are deliberately excluding people who don’t use the Internet.
This way, older and less educated individuals are left out of your sample. Since internet users and non-users differ significantly, you can’t draw reliable results from your online survey.
What are the impacts of selection bias?
There is always the possibility of random or systematic errors in research that compromise the reliability of research outcomes. Selection bias can have various impacts, and it’s often hard to tell how significant or in which direction those effects are. The impacts can lead to several issues for businesses, including the following:
- Risk of losing revenue and reputation
For business planning and strategy, insights obtained from non-representative samples are significantly less helpful because they don’t align with the target population. There is a risk of losing money and reputation if business decisions are based on these findings.
- Impacts the external validity of the analysis
Research becomes less trustworthy as a result of inaccurate data. Therefore, the analysis’s external validity compromises because of the biased sample.
- This leads to inappropriate business decisions
If the final results are biased and unrepresentative of the topic, it is unsafe to rely on the study’s findings when making important business decisions.
We have published a blog that talks about subgroup analysis; why don’t you check it out for more ideas?
How to avoid selection bias?
There’s a good chance you affected your survey results through selection bias. Review the following advice to help you avoid selection bias:
Avoid selection bias during survey design
Try some of these suggestions to avoid selection bias when you are developing the structure for your survey:
- Make sure that your survey objectives are apparent.
- Specify the standards that should be met for your intended audience.
- Allow every possible participant a fair opportunity to take part in the survey.
Avoid selection bias during sampling
Consider putting some of these strategies into practice during the process of selecting samples:
- When employing random sampling in your processes, ensure proper randomization.
- Be sure that your list of participants is up to date and accurately represents the intended audience.
- Make sure that the subgroups represent the population as a whole and share the essential factors.
Avoid selection bias during the evaluation
When going through the evaluation and validation process, you need to think about putting some of these ideas into action to avoid selection bias:
- If you want to ensure that your sample selection, procedure, and data collection are free of bias, having a second researcher look over your back is a good idea.
- Apply technology to monitor how the data changes so you may identify unexpected outcomes and investigate quickly to repair or avoid inaccurate data.
- Check previous basic research data trends to verify if your research is on track for strong internal validity.
- Invite the people who didn’t answer the survey to an additional one. A second round might yield more votes for a clearer understanding of the findings.
Conclusion
Understanding selection bias, its types, and how it affects research outcomes is the beginning step in working with it. We’ve discovered crucial data that will help in identifying it and working to reduce its impacts to a minimum. You can avoid selection bias by using QuestionPro to gather reliable research data.
Various situations can result in selection bias, such as when non-neutral samples are combined with system problems. An enterprise-grade research tool to use in research and alter experiences is the QuestionPro research suite.
QuestionPro research suite provides survey templates based on professional research, making it easier to develop surveys. Learn more about surveys and get started with our survey software by creating a free account.