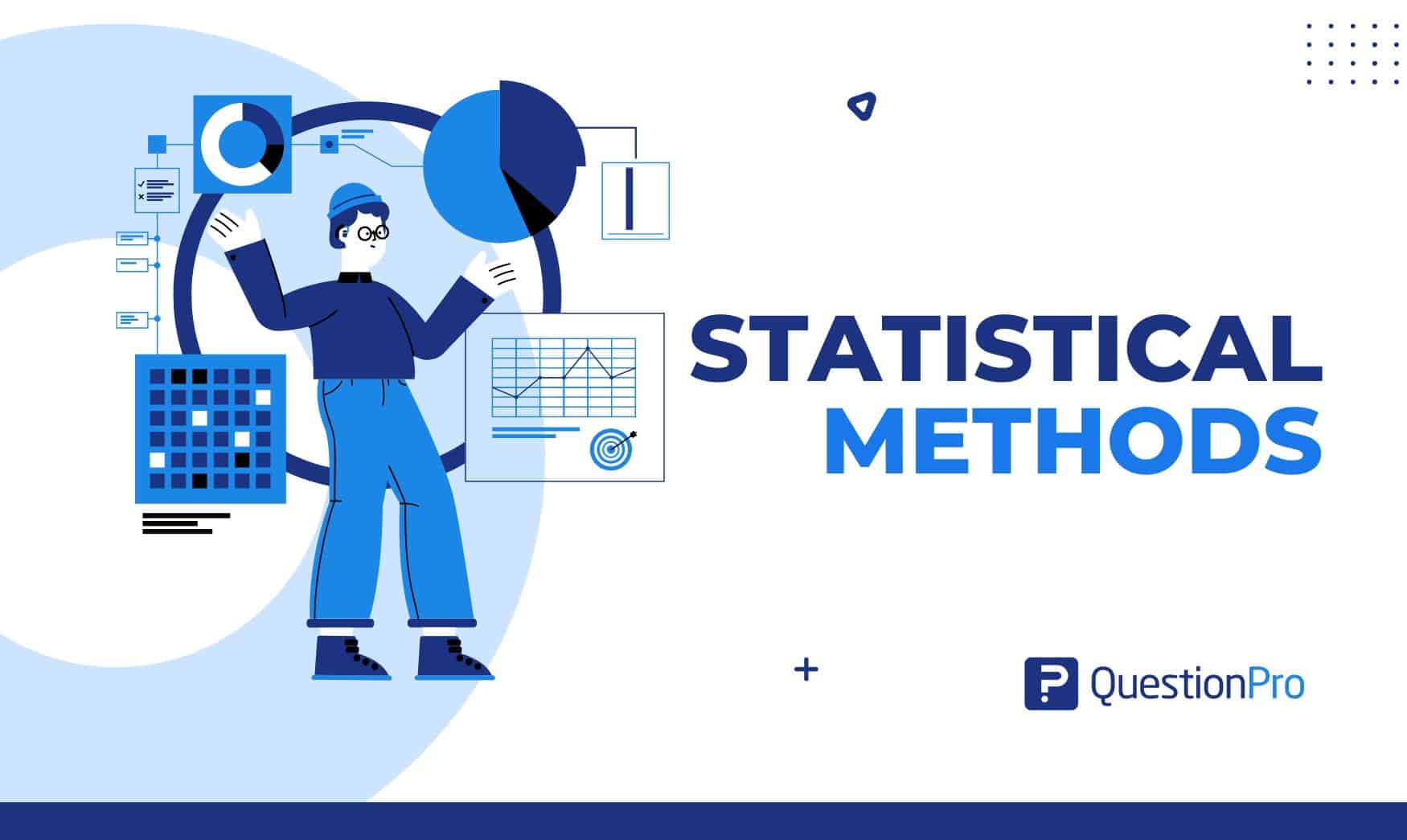
Statistical methods are vital in transforming raw data into actionable insights across various fields. Researchers, analysts, and decision-makers can collect, organize, analyze, interpret, and present data effectively using these mathematical techniques.
These methods facilitate understanding complex data sets, uncovering patterns, and making informed decisions in business, healthcare, social sciences, and engineering.
Statistical methods provide a systematic approach to data analysis, from summarizing data with descriptive statistics to making predictions and testing hypotheses with inferential techniques.
This blog explores key components of statistical methods, including data collection, organization, analysis, interpretation, and presentation. It also discusses best practices, common challenges, and how QuestionPro Research enhances statistical analysis to support exceptional decision-making.
What are Statistical Methods?
Statistical Methods are mathematical techniques and processes used to collect, organize, analyze, interpret, and present data. These methods are helpful for:
- Researchers
- Analysts
- Decision-makers
They are usually used to make sense of large data sets, identify patterns, and draw meaningful conclusions. Statistical methods are essential in transforming raw data into actionable insights, making them a cornerstone of business, healthcare, social sciences, engineering, and more.
Key Components of Statistical Methods:
- Data Collection: Gathering data through various means such as surveys, experiments, or observational studies.
- Data Organization: Structuring and summarizing the collected data meaningfully using tables, graphs, and summary statistics are everywhere.
- Data Analysis: Applying statistics techniques to explore relationships, test hypotheses, and make predictions based on the data.
- Data Interpretation: Concluding the analysis, understanding the implications of the findings, and making decisions based on the results.
- Presentation: Communicating the findings effectively through reports, charts, and presentations to make the information accessible to others.
Statistical methods provide a systematic approach to understanding and interpreting data, allowing for informed decision-making in various disciplines.
Types of Statistical Methods
Statistical methods can be broadly categorized into several types based on their purpose and the nature of the data they analyze. Here are the main types:
01. Descriptive Statistics
Descriptive Statistics are used to summarize and describe the main features of a data set. They provide simple summaries of the sample and the measures, offering a way to understand the basic aspects of the data.
- Mean: The arithmetic average of a data set, calculated by adding all the values and dividing by the number of observations. It is a measure of central tendency that provides insight into the general magnitude of the data.
- Median: The middle value of a data set when ordered from least to greatest. If the data set has an even number of observations, the median is the average of the two middle numbers. The median helps us understand the central tendency, especially in skewed distributions.
- Mode: The value that appears most frequently in a data set. A data set may have one mode, more than one, or no mode. The mode is particularly useful in categorical data analysis.
- Standard Deviation: A measure of the dispersion or spread of data around the mean. It indicates how much the values in a data set deviate from the mean, with a higher standard deviation signifying greater variability.
- Range: The difference between a data set’s maximum and minimum values. The range provides a measure of the spread of the data, but it is sensitive to outliers.
02. Inferential Statistics
Inferential Statistics allow researchers to make predictions or inferences about a population based on a sample of data. These methods test hypotheses, estimate population parameters, and explore relationships between variables.
- Hypothesis Testing: A method for testing a hypothesis about a population parameter based on sample data. It involves formulating a null hypothesis (H0) and an alternative hypothesis (H1) and using statistical tests to determine whether to reject the null hypothesis.
- T-Test: A hypothesis test used to compare the means of two groups. It assesses whether the difference between the means is statistically significant. The t-test is commonly used in small sample sizes.
- Chi-Square Test: A statistical test used to examine the association between categorical variables. It compares the observed frequencies of categories with the expected frequencies to determine if there is a significant relationship.
- ANOVA (Analysis of Variance): A technique used to compare the means of three or more groups. ANOVA tests whether the differences among group means are statistically significant and is often used in experimental research.
- Confidence Intervals: A range of values derived from sample data likely to contain the true population parameter. For example, a 95% confidence interval suggests a 95% chance that the interval contains the actual parameter value. Confidence intervals provide a measure of the precision of an estimate.
- Regression Analysis: A statistical technique used to examine the relationship between dependent and independent variables. It helps predict the dependent variable’s value based on one or more independent variables.
- Linear Regression: A type of regression analysis where the relationship between the dependent variable and one independent variable is modeled as a straight line. Linear regression is used to predict outcomes and understand the strength of the relationship between variables.
- Multiple Regression: An extension of linear regression that involves two or more independent variables. It allows for a more comprehensive analysis of how various factors contribute to the outcome of the dependent variable.
- Correlation: A measure of the strength and direction of the relationship between two variables. The correlation coefficient ranges from -1 to 1, where -1 indicates a perfect negative correlation, 0 means no correlation, and 1 indicates a perfect positive correlation. Correlation is used to identify and quantify relationships between variables.
Applications of Statistical Methods
Statistical methods are indispensable across various industries and fields. They enable data-driven decision-making, optimize processes, and provide insights that drive innovation and improvements. Below are key applications of statistical methods in different sectors:
1. Business
In business, statistical methods are critical for analyzing data to inform strategies, optimize operations, and predict future trends.
- Marketing Analysis: Statistical methods help businesses understand customer behavior, segment markets, and measure the effectiveness of marketing campaigns. Techniques like regression analysis and hypothesis testing are used to identify which factors drive sales and how to allocate marketing budgets efficiently.
- Sales Forecasting: Businesses use statistical models to predict future sales based on historical data. Time series analysis and regression models are commonly employed to forecast demand, helping companies manage inventory, plan production, and set sales targets.
- Product Quality Improvement: Statistical methods such as control charts, Six Sigma, and design of experiments (DOE) are used to monitor and improve product quality. These techniques help identify defects, optimize manufacturing processes, and ensure that products meet customer expectations.
2. Healthcare
In healthcare, statistical methods are vital for research, diagnosis, and treatment planning, contributing to better patient outcomes and advancements in medical science.
- Clinical Trials: Statistical analysis is essential in designing and evaluating clinical trials. It helps determine the efficacy and safety of new treatments or drugs. Techniques like randomization, hypothesis testing, and survival analysis are used to analyze trial data and draw reliable conclusions.
- Disease Pattern Analysis: Epidemiologists use statistical methods to study the distribution and determinants of diseases in populations. Logistic regression and survival analysis help identify risk factors, track disease outbreaks, and develop public health interventions.
- Treatment Effectiveness: Statistical methods are used to assess the effectiveness of medical treatments by comparing patient outcomes before and after treatment. Methods such as paired t-tests, ANOVA, and meta-analysis are commonly used in these evaluations.
3. Social Sciences
In the social sciences, statistical methods study human behavior, social trends, and relationships between variables. They provide empirical evidence that supports theories and informs policy decisions.
- Survey Analysis: Surveys are a common data collection method in social sciences, and statistical analysis helps in interpreting the results. Techniques like factor analysis, regression, and correlation are used to analyze survey data, identify trends, and draw conclusions about populations.
- Behavioral Studies: Researchers use statistical methods to explore underlying patterns in human behavior, such as consumer preferences, social interactions, and decision-making processes. Cluster analysis, ANOVA, and structural equation modeling (SEM) help uncover underlying factors and relationships in behavioral data.
4. Engineering
In engineering, statistical methods improve the design, production, and reliability of products and processes, ensuring efficiency and quality in manufacturing and operations.
- Quality Control: Statistical Process Control (SPC) techniques, such as control charts and process capability analysis, monitor production processes and maintain product quality. These methods help detect and correct variations before they lead to defects.
- Reliability Testing: Engineers use statistical methods to evaluate product reliability and durability. Techniques like life data analysis, Weibull analysis, and failure mode and effects analysis (FMEA) help predict product lifespans and identify potential points of failure.
- Process Optimization: Statistical methods, such as the design of experiments (DOE) and response surface methodology (RSM), are used to optimize manufacturing processes. These techniques help identify the best combination of factors to achieve desired outcomes, such as maximizing efficiency or minimizing costs.
Best Practices for Using Statistical Methods
Using statistical methods effectively requires adherence to several best practices to ensure the results’ accuracy, reliability, and relevance. Here are some key best practices to consider:
- Define Clear Objectives: Before selecting any statistical method, clearly define the objectives of your analysis. Understanding your goal will guide your choice of appropriate techniques and tools.
- Understand Your Data: Conduct thorough exploratory data analysis (EDA) to understand your data’s distribution, patterns, and potential anomalies. This step helps you select the right statistical methods and avoid incorrect assumptions.
- Choose the Right Method: Select statistical methods that align with your data type and research objectives. For example, use regression analysis to predict outcomes, ANOVA to compare group means, and chi-square tests to test categorical data.
- Check Assumptions: Most statistical methods have underlying assumptions (e.g., normality, homoscedasticity, independence). Ensure your data meets these assumptions; if not, consider data transformation or alternative methods.
- Avoid Overfitting: When building predictive models, avoid overfitting by using less complex models that fit the noise in your data rather than the underlying trend. Cross-validation techniques can help assess model performance.
- Ensure Data Quality: Your data quality directly impacts the quality of your results. Ensure data is clean, consistent, and error-free before applying statistical methods.
- Interpret Results in Context: Statistical significance does not always imply practical significance. Interpret your results in the context of your research question and real-world implications.
- Document Your Process: Keep detailed records of your data analysis process, including the methods used, assumptions made, and rationale behind your choices. This ensures the transparency and reproducibility of your work.
- Validate Findings: Use multiple methods or datasets to validate your findings. Consistent results across different approaches enhance the credibility of your analysis.
By following these best practices, you can leverage statistical methods to produce meaningful, actionable insights.
Challenges and Limitations
When using statistical methods, several challenges and limitations can impact the quality and reliability of your analysis. Here are some key challenges:
1. Data Quality Issues:
One of the primary challenges in statistical analysis is ensuring data quality. Poor data quality, such as missing values, outliers, and inconsistencies, can lead to biased or inaccurate results.
Data collected from various sources might have errors or not be representative of the population, which compromises the reliability of the analysis. Addressing these issues often requires substantial preprocessing, which can be time-consuming and complex.
2. Misinterpretation of Results:
Statistical methods can produce complex results that are sometimes counterintuitive. A common limitation is the misinterpretation of statistical significance as practical significance. For example, a statistically significant result may have little real-world impact.
Also, misunderstanding the implications of p-values, confidence intervals, and correlation versus causation can lead to incorrect conclusions that misinform decision-making processes.
3. Selection of Appropriate Methods:
Choosing the correct statistical method is crucial, yet it can be challenging, especially for complex data sets or when multiple variables are involved. Inappropriate method selection can lead to invalid results or missed insights.
This challenge is compounded by the vast array of available statistical techniques, each with its assumptions and applicability. The complexity increases when dealing with non-standard data types, such as time series or categorical data, where specialized methods are required.
These challenges highlight the need for a solid foundational understanding of statistical principles, careful data handling, and a thoughtful approach to method selection and result interpretation. Awareness of these limitations can help mitigate their impact and improve the robustness of statistical analyses.
QuestioPro Research Suite in Statistical Methods
QuestionPro Research Suite offers tools designed to enhance careers in statistical analysis and data interpretation, providing valuable insights for decision-making. Here’s an overview of how QuestionPro integrates statistical methods to support robust research:
01. Advanced Statistical Tools
QuestionPro Research Suite provides advanced statistical tools to help users quickly perform complex analyses. Features include descriptive statistics, cross-tabulations, and inferential tests such as Pearson’s Chi-Square Test. These tools allow researchers to explore data patterns, test hypotheses, and draw meaningful conclusions.
02. Customizable Analysis Options
The platform offers customizable analysis options, enabling users to tailor their statistical approach based on specific research needs. Users can select from various statistical methods and configure parameters to fit their unique data characteristics. This flexibility ensures that the analysis aligns with the research objectives and provides relevant insights.
03. Data Quality Assurance
QuestionPro emphasizes the importance of data quality in statistical analysis. The platform includes data cleaning and validation features, helping users identify and address missing values, outliers, and inconsistencies. By ensuring high-quality data, users can enhance the accuracy and reliability of their statistical computing results.
04. Visualizations and Reporting
QuestionPro provides robust visualization tools to facilitate the interpretation of statistical results. Users can generate charts, graphs, and dashboards visually representing data and statistical findings. These visualizations make it easier to understand complex results and communicate insights effectively to stakeholders.
05. User-Friendly Interface
Despite offering advanced statistical capabilities, QuestionPro maintains a user-friendly interface that simplifies the process of performing statistical analyses. Intuitive navigation and guided workflows help users efficiently conduct and interpret analyses, regardless of their statistical expertise.
06. Integration and Support
QuestionPro Research Suite integrates with other data sources and analytical tools, enhancing the flexibility of statistical analysis. The platform also offers support and resources to help users apply statistical methods and interpret results accurately.
QuestionPro equips users with the tools and supports to conduct thorough and accurate statistical analyses, facilitating informed decision-making based on reliable data insights.
Conclusion
Statistical methods are essential for converting raw data into actionable insights across diverse fields. Techniques like descriptive statistics summarize data characteristics, while inferential methods predict, test hypotheses, and conclude broader populations.
Applications span business, healthcare, social sciences, and engineering, helping optimize strategies, assess treatment effectiveness, analyze data behavior, and improve product quality.
Best practices for statistical analysis include defining objectives, understanding data, choosing appropriate methods, checking assumptions, avoiding overfitting, ensuring data quality, and interpreting results contextually. Despite their utility, data quality issues and method selection difficulties can arise.
QuestionPro Research Suite enhances statistical analysis with advanced tools, customizable options, data quality assurance, and user-friendly interfaces, supporting accurate and effective data-driven decision-making.