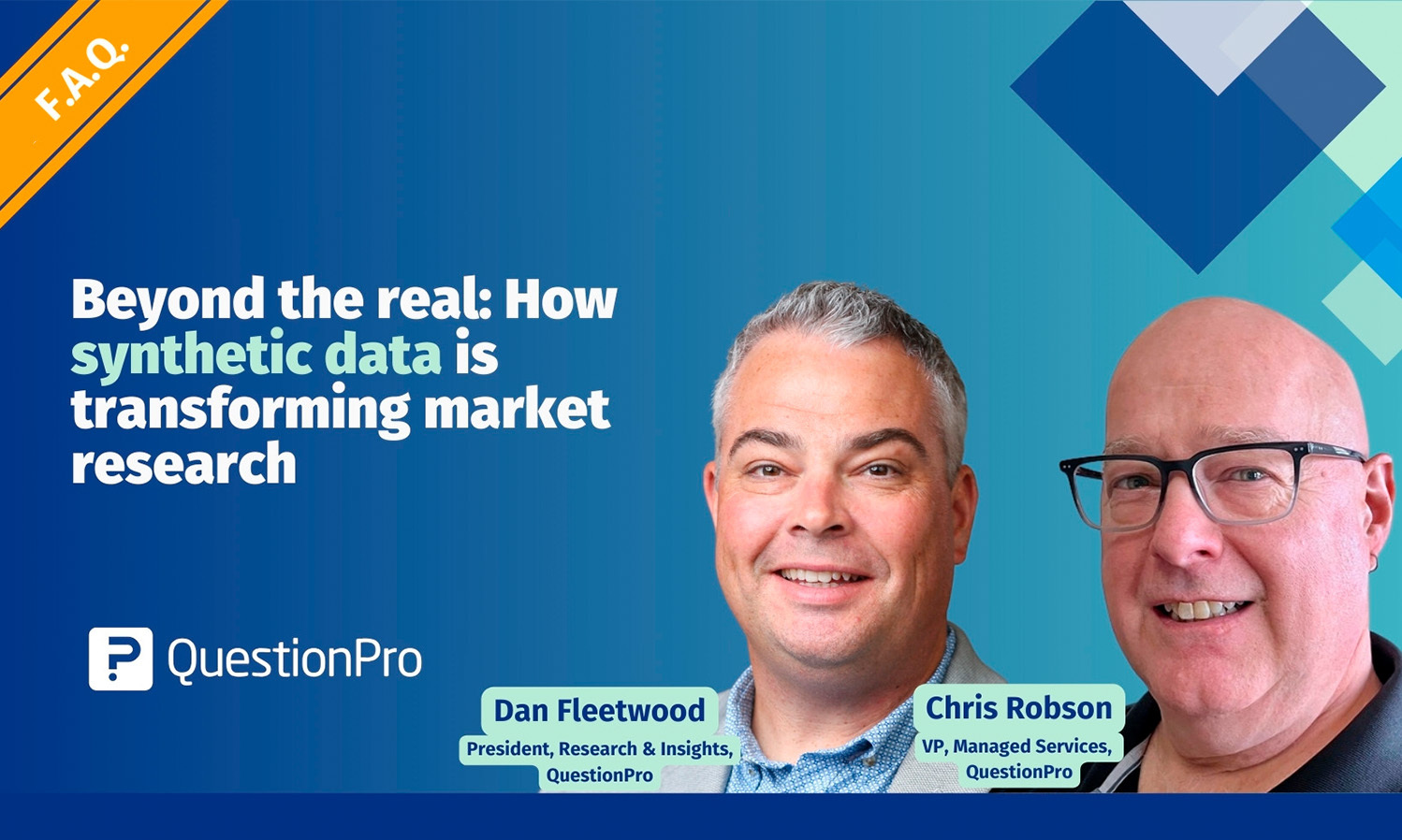
The challenge is clear: traditional data gathering can be time-consuming, costly, and often limits the research scale. The solution? Synthetic and augmented data. These two innovative techniques are changing the landscape of data generation and offering exciting new possibilities for optimizing data collection methods.
What Are Synthetic And Augmented Data?
First, let’s clarify what synthetic data and augmented data are. While these terms are often used interchangeably, they refer to distinct concepts:
- Synthetic data is entirely generated by algorithms rather than collected from real-world sources. It can be used to simulate scenarios and create datasets when real data is scarce or expensive to acquire.
- Augmented data takes existing, real-world data and enhances or modifies it to create more diverse datasets. This method doesn’t replace the original data but adds to it, offering a richer perspective.
To learn more, you can watch our webinar an slides on Synthetic Data below.
The Rise & Impact Of Synthetic Data: Moving Fast And Cost-Effectively
Synthetic data is gaining traction because it offers a practical solution to two significant challenges: speed and cost. Research teams can generate large datasets quickly and at a fraction of the cost of traditional methods. This agility makes synthetic data particularly appealing for businesses needing quick insights or working with limited resources.
However, it’s important to note that synthetic data is yet to be a substitute for high-quality, real-world data. While synthetic data excels at moving quickly and cheaply, it often lacks the depth and accuracy that come from traditional methods like qualitative research or expert sampling. In other words, synthetic data is best used in conjunction with real data to complement and enhance research efforts.
Addressing Data Quality Concerns
One key concern when working with synthetic data is the quality of the data it’s based on. The classic “garbage in, garbage out” rule still applies. If synthetic data is built on poor-quality data, the resulting dataset will likely be flawed.
For instance, if the underlying data includes errors or biases, synthetic data will replicate and amplify these issues. This is why ensuring that the data used to generate synthetic datasets is accurate and reliable is crucial. Synthetic data works best when grounded in solid, high-quality, real-world data.
Emerging Applications Of Synthetic Data Across Industries
While synthetic data may seem like a niche tool today, it is poised to become mainstream in the near future.
One area where synthetic data is already gaining traction is in the creation of synthetic personas. Businesses are increasingly using synthetic personas to bring customer segments to life, providing a more detailed and human-like view of their target audiences. This trend is set to continue, and it’s likely that personas will become standard components of segmentation reports.
Another area where synthetic data will play a more prominent role is in upfront research planning. For example, when launching a new product, companies can use synthetic data to simulate consumer responses and anticipate potential market reactions. This allows for more informed decision-making without the time and cost associated with traditional focus groups or surveys.
Key Questions About Synthetic Data
In addition to the insights shared in our webinar, here are some of our audience’s most relevant and thought-provoking questions, along with our experts’ answers. These questions highlight the key challenges and opportunities surrounding synthetic and augmented data.
These questions have been answered by Chris Robson, Vice President of Managed Services at QuestionPro, and Dan Fleetwood, President of Research and Insights at QuestionPro. They share with us their unified experiences and reflections about the impact of synthetic data in the recent evolution of the research market.
Q) What are the main challenges in generating high-quality synthetic data?
- The main challenge in generating high-quality synthetic data is ensuring that the models used to create it are accurate and unbiased. If the underlying algorithms are flawed, the synthetic data could fail to reflect real-world scenarios, affecting the outcomes of tests or simulations. Additionally, maintaining privacy while generating synthetic data from real-world sources is a challenge that must be carefully managed.
Q) How can augmented data improve decision-making in industries like healthcare?
- Augmented data can be used in healthcare to add additional layers of information to patient records or clinical data, allowing for more comprehensive analyses. By enriching the data with new variables, healthcare providers can improve diagnostic accuracy, predict outcomes more effectively, and personalize patient treatments. For instance, combining patient history with lifestyle factors could lead to more precise predictions of health risks.
Q) Can synthetic data be used to train machine learning models?
- Absolutely. Synthetic data is particularly valuable for training machine learning models when access to real-world data is limited or costly. Machine learning models can be trained and tested in a controlled, safe environment by generating synthetic data that mirrors real-world conditions. This is especially useful in fields like autonomous vehicles, where generating real-world data for training purposes can be expensive and dangerous.
Q) How do you ensure the ethical use of synthetic and augmented data?
- Ethical concerns related to synthetic and augmented data can be addressed by ensuring transparency and fairness in the data generation process. It’s essential to use algorithms and models that are unbiased and representative of diverse populations. Additionally, when working with augmented data, it’s crucial to respect privacy and avoid distorting real-world data in ways that could mislead decision-makers or harm individuals.
Q) What is the future of synthetic data in mainstream industries?
- The future of synthetic data is bright, as it is increasingly being adopted across various industries. We will likely see more widespread use in sectors like healthcare, finance, automotive, and retail. As the technology improves, we can expect synthetic data to become a standard tool for training AI models, conducting simulations, and enhancing research, all while maintaining privacy and efficiency.
Get the inside scoop: Bonus Q&A Session
After watching our synthetic data webinar, don’t miss out on the bonus Q&A session where we answer your most pressing questions about synthetic and augmented data. In this exclusive follow-up, we dive deeper into specific use cases, address audience concerns, and share tips on how you can leverage these data strategies in your own work.
A Bright Future For Data Generation
The future of data generation is bright, with synthetic data playing a big role in driving innovation and efficiency across industries. These synthetic data generation tools provide a way to create and enhance data that can fuel advancements in AI, machine learning, and research without the limitations of traditional data collection methods.
If you are ready to take advantage of synthetic and augmented data in your research projects, then you are at the right place. QuestionPro offers powerful tools to help you effectively leverage these innovative data techniques.
About Our Speakers
Chris Robson is the Vice President of Managed Services at QuestionPro, bringing over two decades of experience in data science, innovation, and analytics. Prior to joining QuestionPro, he was the Global Head of Data Science at Human8, a leading global brand consultancy, where he pioneered new methodologies, particularly in the application of Generative AI and Large Language Models (LLMs) to drive cutting-edge solutions.
Chris’s earlier career includes leading advanced research and software teams at HP, where he managed over 70 individuals to deliver innovative technology solutions. As Chief Innovation Officer and Global Head of Research Science at ORC, he spearheaded the adoption of novel data approaches, shaping the company’s data strategy with a focus on actionable insights.
A seasoned entrepreneur, Chris co-founded and successfully ran two research analytics agencies: Parametric Marketing and Deckchair Data. He holds a Bachelor of Science with Honors in Mathematics from Brunel University of London.